Adjacency Matrix Decomposition Clustering for Human Activity Data
arxiv(2024)
摘要
Mobile apps and wearable devices accurately and continuously measure human
activity; patterns within this data can provide a wealth of information
applicable to fields such as transportation and health. Despite the potential
utility of this data, there has been limited development of analysis methods
for sequences of daily activities. In this paper, we propose a novel clustering
method and cluster evaluation metric for human activity data that leverages an
adjacency matrix representation to cluster the data without the calculation of
a distance matrix. Our technique is substantially faster than conventional
methods based on computing pairwise distances via sequence alignment algorithms
and also enhances interpretability of results. We compare our method to
distance-based hierarchical clustering through simulation studies and an
application to data collected by Daynamica, an app that turns raw sensor data
into a daily summary of a user's activities. Among days that contain a large
portion of time spent at home, our method distinguishes days that also contain
full-time work or multiple hours of travel, while hierarchical clustering
groups these days together. We also illustrate how the computational advantage
of our method enables the analysis of longer sequences by clustering full weeks
of activity data.
更多查看译文
AI 理解论文
溯源树
样例
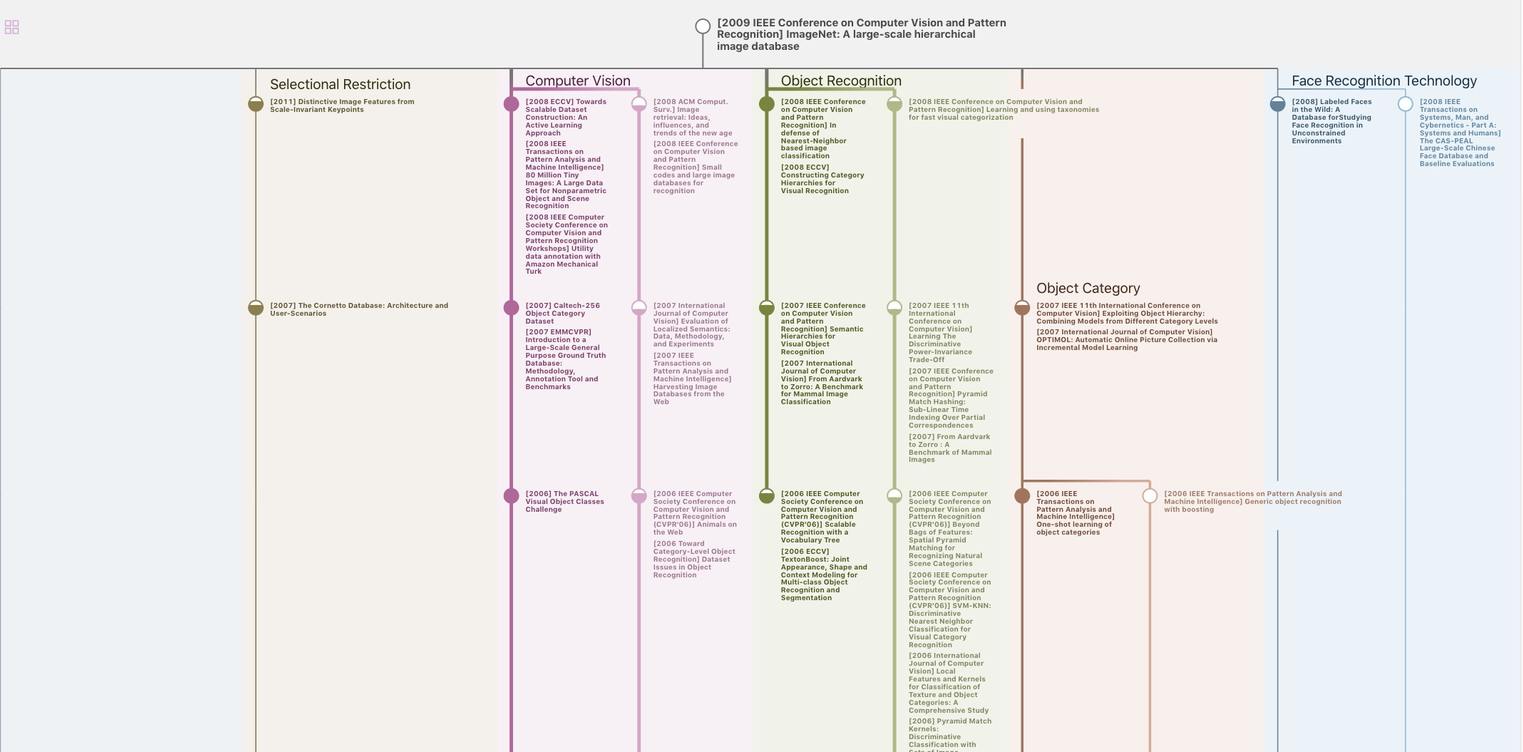
生成溯源树,研究论文发展脉络
Chat Paper
正在生成论文摘要