AdvReverb: Rethinking the Stealthiness of Audio Adversarial Examples to Human Perception
IEEE TRANSACTIONS ON INFORMATION FORENSICS AND SECURITY(2024)
摘要
As one of the most representative applications built on deep learning, audio systems, including keyword spotting, automatic speech recognition, and speaker identification, have recently been demonstrated to be vulnerable to adversarial examples, which have already raised general concerns in both academia and industry. Existing attacks follow the same adversarial example generation paradigm from computer vision, i.e., overlaying the optimized additive perturbations on original voices. However, due to the additive perturbations' nature on human audibility, balancing the stealthiness and attack capability remains a challenging problem. In this paper, we rethink the stealthiness of audio adversarial examples and turn to introduce another kind of audio distortion, i.e., reverberation, as a new perturbation format for stealthy adversarial example generation. Such convolutional adversarial perturbations are crafted as real-world impulse responses and behave as a natural reverberation for deceiving humans. Based on this idea, we propose AdvReverb to construct, optimize, and deliver phoneme-level convolutional adversarial perturbations on both speech and music carriers with a well-designed objective. Experimental results demonstrate that AdvReverb could realize high attack success rates over 95% on three audio-domain tasks while achieving superior perceptual quality and keeping stealthy from human perception in over-the-air and over-the-line delivery scenarios.
更多查看译文
关键词
Convolutional adversarial example,automatic audio system,impulse response,stealthy audio perturbation
AI 理解论文
溯源树
样例
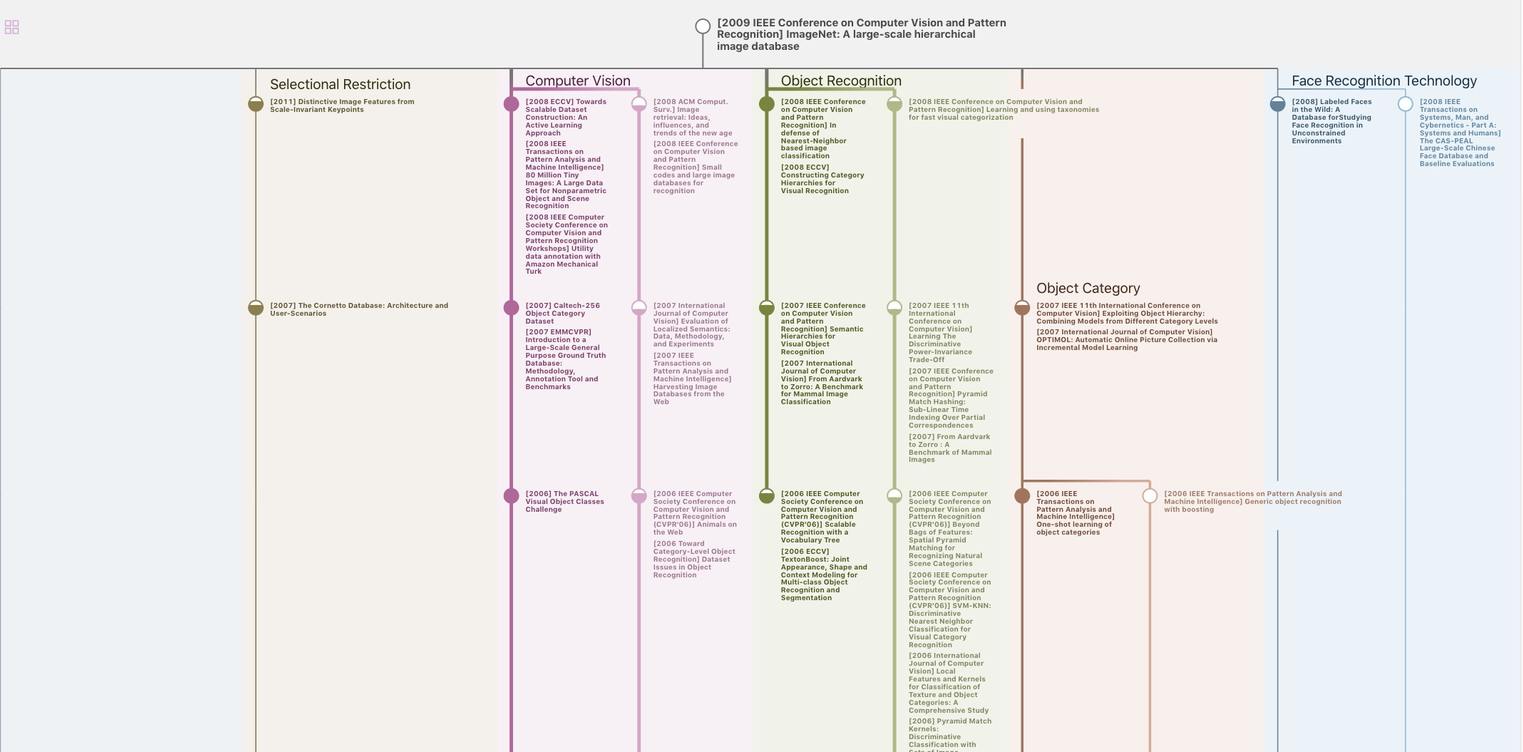
生成溯源树,研究论文发展脉络
Chat Paper
正在生成论文摘要