A Modular Framework for Robot Embodied Instruction Following by Large Language Model
IEEE International Conference on Robotics and Biomimetics(2023)
摘要
In the ALFRED challenge for robot simulation, the robot still faces a challenge to schedule a task in the embodied instruction following (EIF) tasks. These tasks require the robot to accurately perceive visual features and understand language instructions. However, the previous approaches typically employed end-to-end structures that utilize a shallow understanding of language instructions, while EIF tasks demand a deeper understanding of the semantic relationships in these instructions. To overcome these limitations, we propose a method which named REIF. The method incorporates modules for visual perception, language understanding, semantic search, closed container prediction, navigation, and operation to form a modular framework based on visual language multi-modal learning. The semantic search module supports more efficient object search, while the closed container prediction module enables deeper language understanding. Through learning multiple task instructions, the robot can efficiently and accurately complete EIF tasks in unseen scenes under certain step lengths. Our framework performs significantly well on unseen scene tasks within the ALFRED benchmark, achieving state-of-the-art accuracy and efficiency rates of 50.83% and 23.06% respectively. These results demonstrate that our method is capable of efficiently and accurately inferring the presence of closed container in unseen scenes, and can successfully execute a series of actions to interact with target object within closed container. Our method has achieved the first place in the ALFRED data-set competition. You can find our submissions and results at the following link: https://leaderboard.allenai.org/alfred/submissions/public.
更多查看译文
AI 理解论文
溯源树
样例
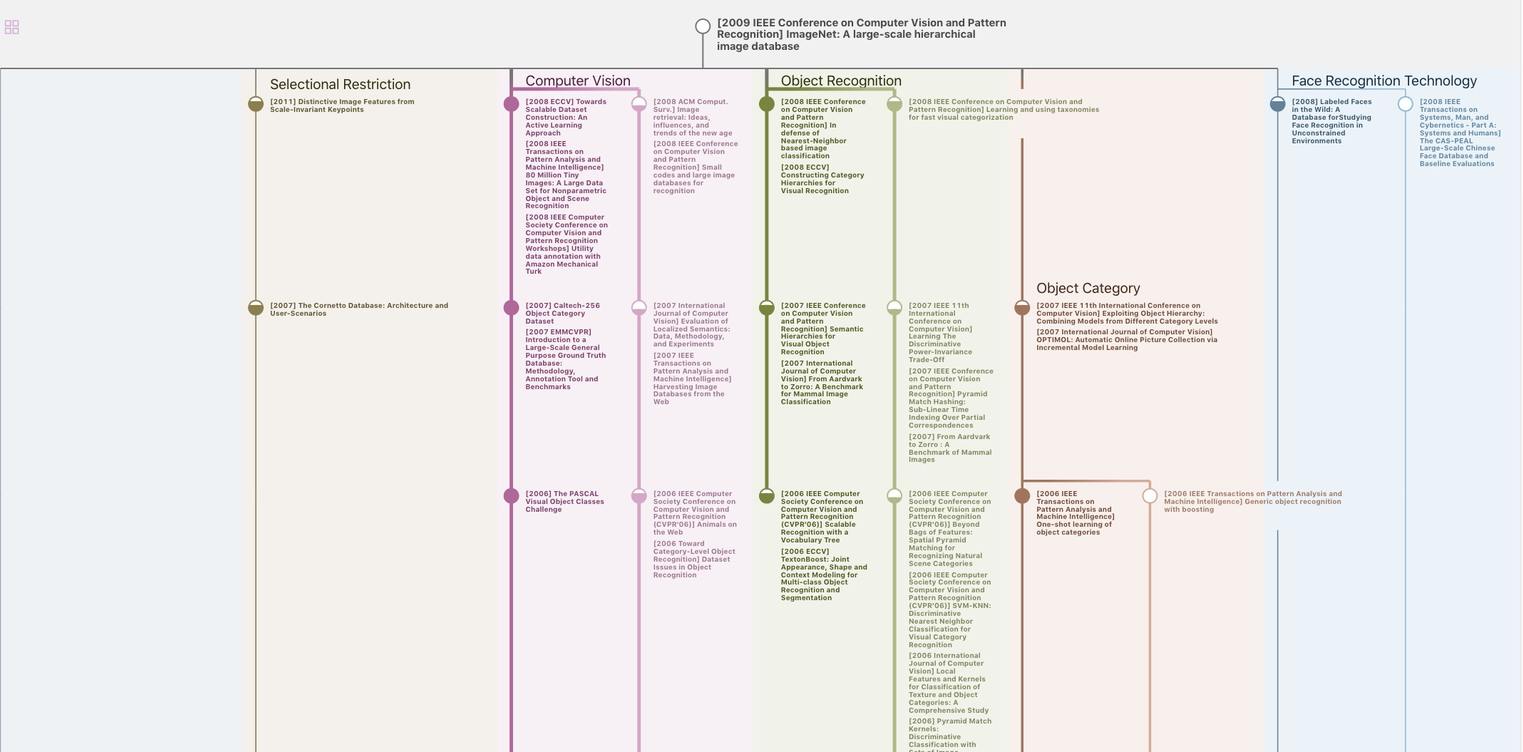
生成溯源树,研究论文发展脉络
Chat Paper
正在生成论文摘要