Cross-Modal Object Tracking via Modality-Aware Fusion Network and a Large-Scale Dataset.
IEEE transactions on neural networks and learning systems(2024)
摘要
Visual object tracking often faces challenges such as invalid targets and decreased performance in low-light conditions when relying solely on RGB image sequences. While incorporating additional modalities like depth and infrared data has proven effective, existing multimodal imaging platforms are complex and lack real-world applicability. In contrast, near-infrared (NIR) imaging, commonly used in surveillance cameras, can switch between RGB and NIR based on light intensity. However, tracking objects across these heterogeneous modalities poses significant challenges, particularly due to the absence of modality switch signals during tracking. To address these challenges, we propose an adaptive cross-modal object tracking algorithm called modality-aware fusion network (MAFNet). MAFNet efficiently integrates information from both RGB and NIR modalities using an adaptive weighting mechanism, effectively bridging the appearance gap and enabling a modality-aware target representation. It consists of two key components: an adaptive weighting module and a modality-specific representation module. The adaptive weighting module predicts fusion weights to dynamically adjust the contribution of each modality, while the modality-specific representation module captures discriminative features specific to RGB and NIR modalities. MAFNet offers great flexibility as it can effortlessly integrate into diverse tracking frameworks. With its simplicity, effectiveness, and efficiency, MAFNet outperforms state-of-the-art methods in cross-modal object tracking. To validate the effectiveness of our algorithm and overcome the scarcity of data in this field, we introduce CMOTB, a comprehensive and extensive benchmark dataset for cross-modal object tracking. CMOTB consists of 61 categories and 1000 video sequences, comprising a total of over 799K frames. We believe that our proposed method and dataset offer a strong foundation for advancing cross-modal object-tracking research. The dataset, toolkit, experimental data, and source code will be publicly available at: https://github.com/mmic-lcl/ Datasets-and-benchmark-code.
更多查看译文
关键词
Cross-modal object tracking,dataset,modality-aware fusion network (MAFNet)
AI 理解论文
溯源树
样例
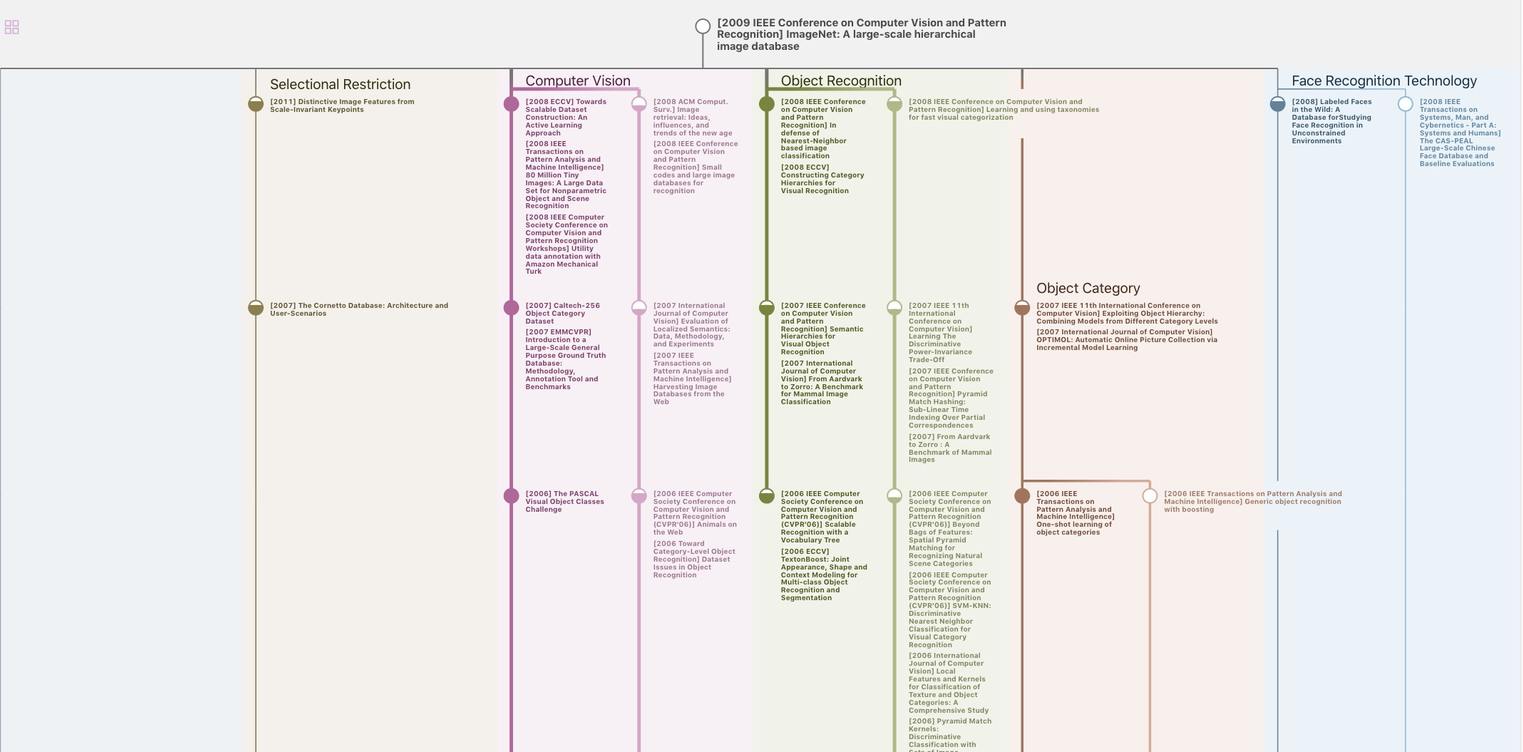
生成溯源树,研究论文发展脉络
Chat Paper
正在生成论文摘要