Limitations in Evaluating Machine Learning Models for Imbalanced Binary Outcome Classification in Spine Surgery: A Systematic Review
BRAIN SCIENCES(2023)
摘要
Clinical prediction models for spine surgery applications are on the rise, with an increasing reliance on machine learning (ML) and deep learning (DL). Many of the predicted outcomes are uncommon; therefore, to ensure the models' effectiveness in clinical practice it is crucial to properly evaluate them. This systematic review aims to identify and evaluate current research-based ML and DL models applied for spine surgery, specifically those predicting binary outcomes with a focus on their evaluation metrics. Overall, 60 papers were included, and the findings were reported according to the PRISMA guidelines. A total of 13 papers focused on lengths of stay (LOS), 12 on readmissions, 12 on non-home discharge, 6 on mortality, and 5 on reoperations. The target outcomes exhibited data imbalances ranging from 0.44% to 42.4%. A total of 59 papers reported the model's area under the receiver operating characteristic (AUROC), 28 mentioned accuracies, 33 provided sensitivity, 29 discussed specificity, 28 addressed positive predictive value (PPV), 24 included the negative predictive value (NPV), 25 indicated the Brier score with 10 providing a null model Brier, and 8 detailed the F1 score. Additionally, data visualization varied among the included papers. This review discusses the use of appropriate evaluation schemes in ML and identifies several common errors and potential bias sources in the literature. Embracing these recommendations as the field advances may facilitate the integration of reliable and effective ML models in clinical settings.
更多查看译文
关键词
machine learning,artificial intelligence,deep learning,predictive modeling,spine surgery
AI 理解论文
溯源树
样例
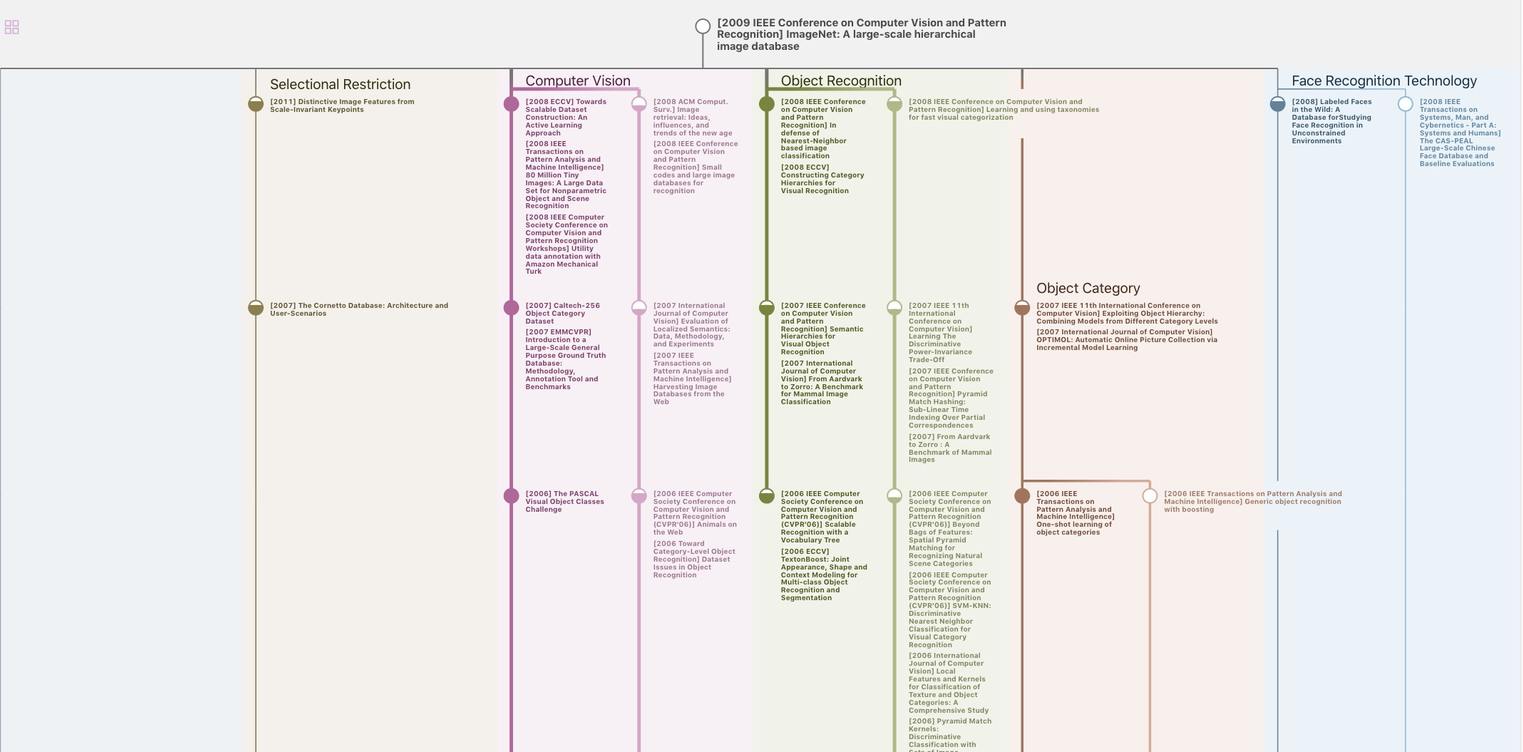
生成溯源树,研究论文发展脉络
Chat Paper
正在生成论文摘要