Process Monitoring by Deep Neural Networks in Directed Energy Deposition: CNN-based Detection, Segmentation, and Statistical Analysis of Melt Pools
ROBOTICS AND COMPUTER-INTEGRATED MANUFACTURING(2024)
摘要
•Conducting experiments using a Central Composite Design of Experiments (CCD) with approved parameters on Stainless Steel (SS), low carbon steel (S355) substrate, and Inconel alloy 625 wire.•Annotating, preparing, training, testing, and comparing YOLO-based instance segmentation methods for real-time weld bead geometry prediction.•Small versions of the CCN-based segmentation models exhibited a notable processing speed of over 114 frames per second, which indicates its suitability for real-time process control.•Introducing and applying a novel stability assessment criterion based on the segmented melt pool area derived from the proposed CNN-based architectures.•A clear correlation was established between real-time melt pool geometry variation and bead geometry, including penetration depth and heat-affected zone. The correlation led to the development of a CNN-based model utilizing segmented melt pool masks obtained from segmentation models to predict bead geometrical characteristics, serving as a use case for the segmentation models.
更多查看译文
关键词
Additive manufacturing,Direct energy deposition,Artificial intelligence,Melt pool monitorings,CNN-based segmentation,Statistical analysis
AI 理解论文
溯源树
样例
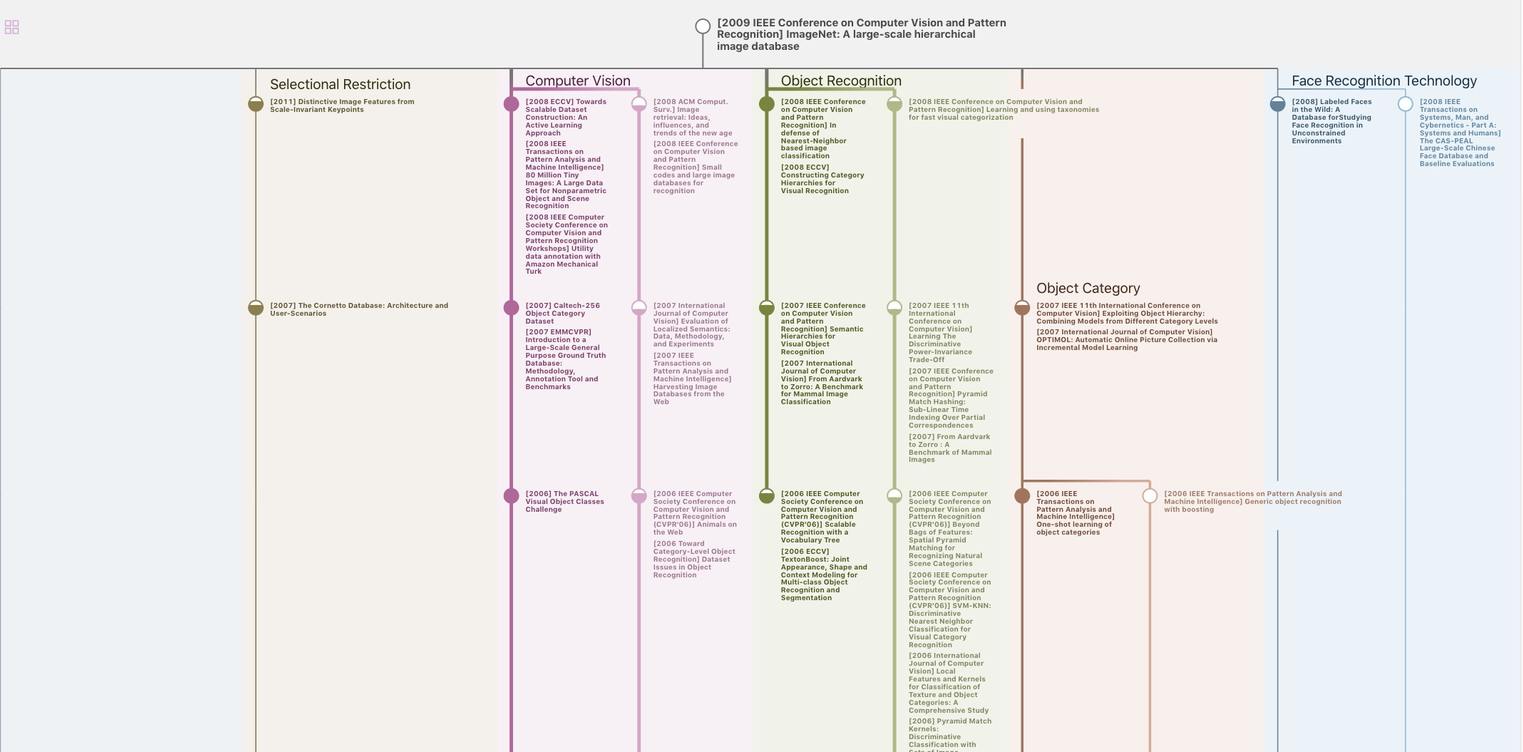
生成溯源树,研究论文发展脉络
Chat Paper
正在生成论文摘要