Knowledge-aware image understanding with multi-level visual representation enhancement for visual question answering
Machine Learning(2023)
摘要
Existing visual question answering (VQA) methods tend to focus excessively on visual objects in images, neglecting the understanding of implicit knowledge within the images, thus limiting the comprehension of image content. Furthermore, current mainstream VQA methods employ a bottom-up attention mechanism, which was initially proposed in 2017 and has become a bottleneck in visual question answering. In order to address the aforementioned issues and improve the ability to understand images, we have made the following improvements and innovations: (1) We utilize an OCR model to detect and extract scene text in the images, further enriching the understanding of image content. And we introduce the descriptive information from the images to enhance the model’s comprehension of the images. (2) We have made improvements to the bottom-up attention model by obtaining two region features from the images, we concatenate the two region features to form the final visual feature, which better represents the image. (3) We design an extensible deep co-attention model, which includes self-attention units and co-attention units. This model can incorporate both image description information and scene text into the model, and it can be extended with other knowledge to further enhance the model’s reasoning ability. (4) Experimental results demonstrate that our best single model achieves an overall accuracy of 74.38
更多查看译文
关键词
Visual question answering,Faster R-CNN,Co-attention,Multi-view region features,Image implicit knowledge
AI 理解论文
溯源树
样例
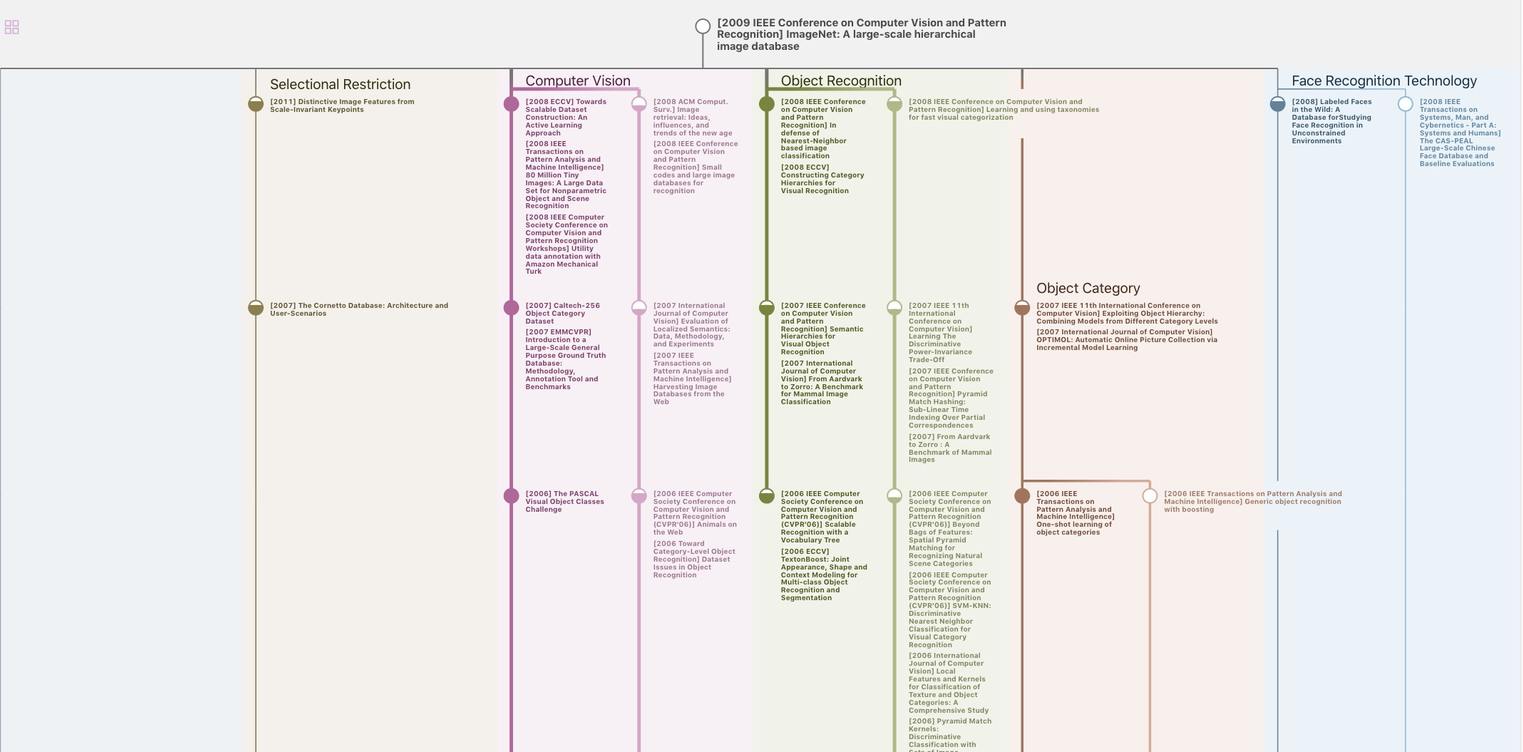
生成溯源树,研究论文发展脉络
Chat Paper
正在生成论文摘要