Solving Partially Observable 3D-Visual Tasks with Visual Radial Basis Function Network and Proximal Policy Optimization
Machine learning and knowledge extraction(2023)
摘要
Visual Reinforcement Learning (RL) has been largely investigated in recent decades. Existing approaches are often composed of multiple networks requiring massive computational power to solve partially observable tasks from high-dimensional data such as images. Using State Representation Learning (SRL) has been shown to improve the performance of visual RL by reducing the high-dimensional data into compact representation, but still often relies on deep networks and on the environment. In contrast, we propose a lighter, more generic method to extract sparse and localized features from raw images without training. We achieve this using a Visual Radial Basis Function Network (VRBFN), which offers significant practical advantages, including efficient and accurate training with minimal complexity due to its two linear layers. For real-world applications, its scalability and resilience to noise are essential, as real sensors are subject to change and noise. Unlike CNNs, which may require extensive retraining, this network might only need minor fine-tuning. We test the efficiency of the VRBFN representation to solve different RL tasks using Proximal Policy Optimization (PPO). We present a large study and comparison of our extraction methods with five classical visual RL and SRL approaches on five different first-person partially observable scenarios. We show that this approach presents appealing features such as sparsity and robustness to noise and that the obtained results when training RL agents are better than other tested methods on four of the five proposed scenarios.
更多查看译文
关键词
visual reinforcement learning,state representation learning,radial basis function network,computer vision
AI 理解论文
溯源树
样例
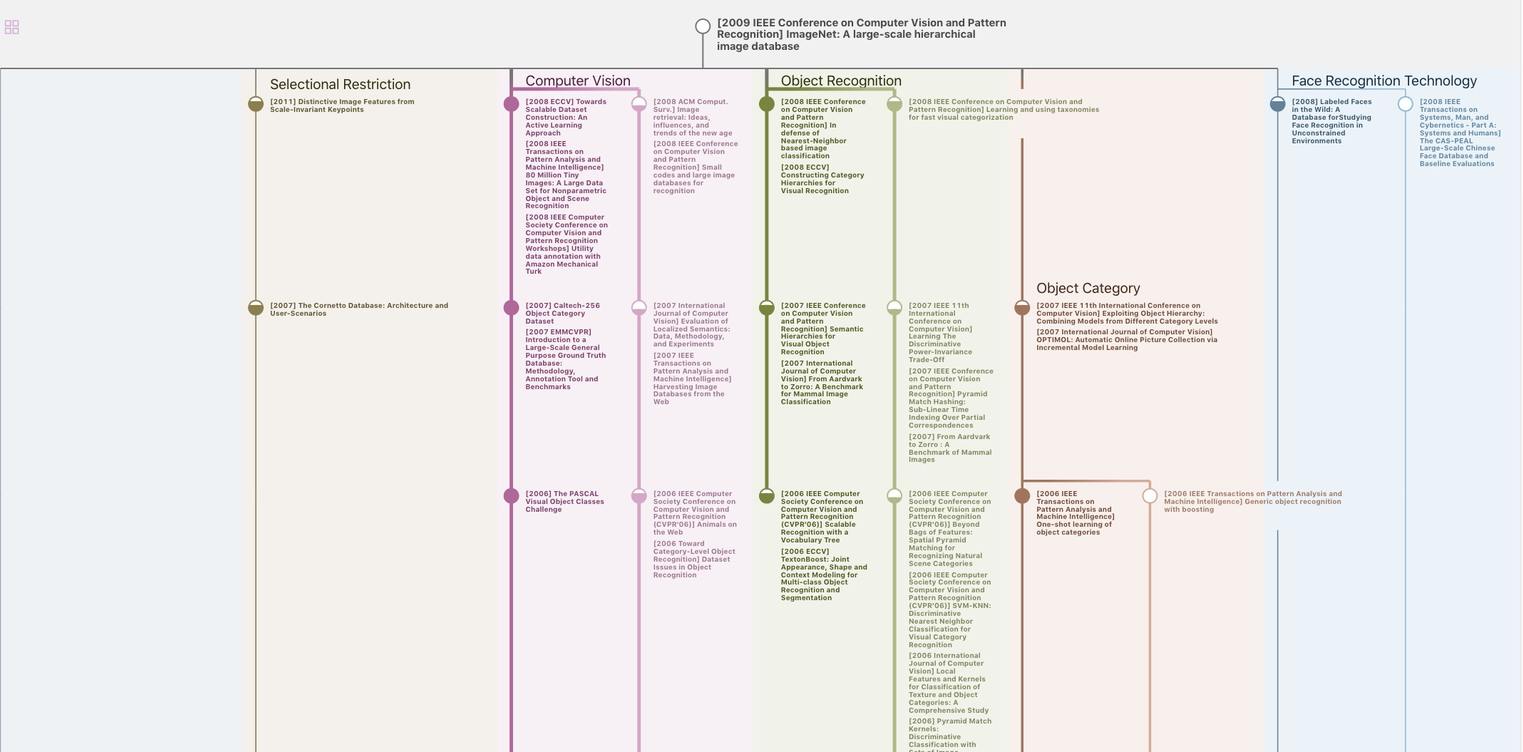
生成溯源树,研究论文发展脉络
Chat Paper
正在生成论文摘要