A multi-facet analysis of BERT-based entity matching models
The VLDB Journal(2023)
摘要
State-of-the-art Entity Matching approaches rely on transformer architectures, such as BERT , for generating highly contextualized embeddings of terms. The embeddings are then used to predict whether pairs of entity descriptions refer to the same real-world entity. BERT-based EM models demonstrated to be effective, but act as black-boxes for the users, who have limited insight into the motivations behind their decisions. In this paper, we perform a multi-facet analysis of the components of pre-trained and fine-tuned BERT architectures applied to an EM task. The main findings resulting from our extensive experimental evaluation are (1) the fine-tuning process applied to the EM task mainly modifies the last layers of the BERT components, but in a different way on tokens belonging to descriptions of matching/non-matching entities; (2) the special structure of the EM datasets, where records are pairs of entity descriptions, is recognized by BERT; (3) the pair-wise semantic similarity of tokens is not a key knowledge exploited by BERT-based EM models; (4) fine-tuning SBERT, a pre-trained version of BERT on the sentence similarity task, i.e., a task close to EM, does not allow the model to largely improve the effectiveness and to learn different forms of knowledge. Approaches customized for EM, such as Ditto and SupCon, seem to rely on the same knowledge as the other transformer-based models. Only the contrastive learning training allows SupCon to learn different knowledge from matching and non-matching entity descriptions; (5) the fine-tuning process based on a binary classifier does not allow the model to learn key distinctive features of the entity descriptions.
更多查看译文
关键词
BERT, Entity matching, Data integration, Transformers
AI 理解论文
溯源树
样例
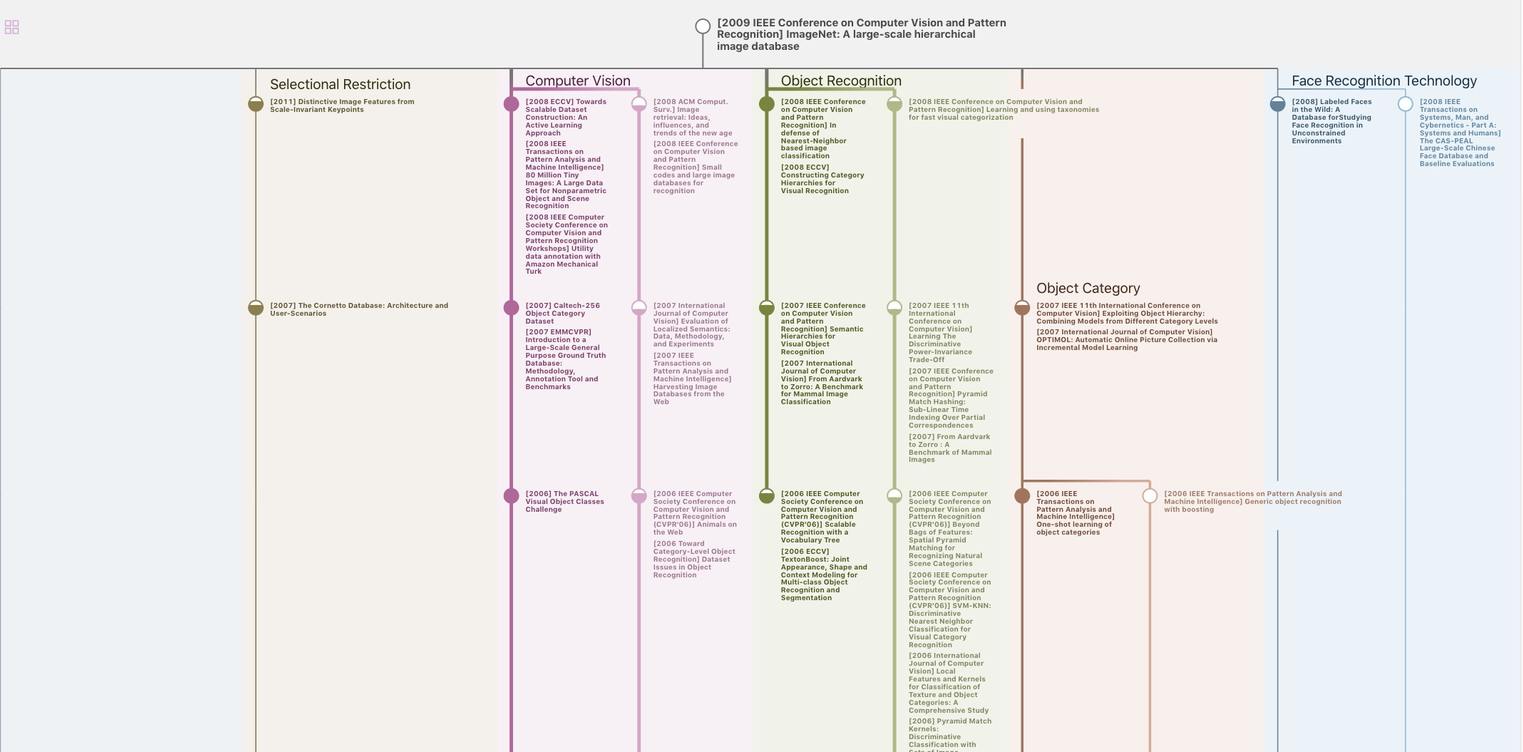
生成溯源树,研究论文发展脉络
Chat Paper
正在生成论文摘要