Development of the Artificial Neural Network’s Swarm-Based Approaches Predicting East Azerbaijan Landslide Susceptibility Mapping
ENVIRONMENT DEVELOPMENT AND SUSTAINABILITY(2023)
Abstract
The objective of this investigation is to produce maps identifying areas prone to landslides (LSMs) by utilizing multiple machine learning techniques, including the harmony search algorithm (HS), shuffled frog leaping algorithm (SFLA), evaporation rate water cycle algorithm (ERWCA), and whale optimization algorithm (WOA). To create a comprehensive inventory of landslide occurrences, high-resolution satellite imagery, topographic maps, historical records, and GPS data gathered through fieldwork were employed. In total, 402 known landslide sites were used for modeling and validating the landslide occurrences in the study area. Sixteen factors were integrated: topography, hydrology, soil type, geology, and ecology. The training dataset comprised 72% of all landslide locations, while the remaining 28% was used to validate the generated landslide occurrences. The different models were evaluated using the area under the receiver operating characteristic curve. The analysis of both the training and validation datasets was used to determine the model’s accuracy. To improve the performance of the multilayer perceptron neural network architecture, the HS, ERWCA, SFLA, and WOA algorithms were applied to improve its efficiency. The accuracy of the applied prediction models was evaluated using the area under the curve (AUC) measure before creating landslide vulnerability charts in a GIS framework. The results showed that the HS-MLP had the greatest estimated AUCs for population sizes in training databases equal to 50, with values of 0.9921 and 0.9828 for training databases and testing databases, respectively. Similar to this, in the training and testing databases, the AUC values for the ER_WCAMLP with a 200-swarm swarm size were determined to be 0.9998 and 0.9821, respectively. For population sizes of 150 and 500, respectively, the training and testing AUCs for the SFLA-MLP were determined to be 0.984 and 0.9821, and 0.9867 and 0.9873, respectively, for the WOA-MLP. The best solution was found after 50, 200, 150, and 500 iterations for the models that used the population in the best fit HS, ER_WCA, SFLA, and WOA algorithms, respectively. In light of these findings, managers and planners can benefit from this research's findings in pre-crisis management as it demonstrates how optimization algorithms have improved the neural network’s accuracy and performance in the evaluation and detection of problematic areas.
MoreTranslated text
Key words
Landslide susceptibility map,Optimization algorithm of ANN,Evolutionary methods,Crisis management
AI Read Science
Must-Reading Tree
Example
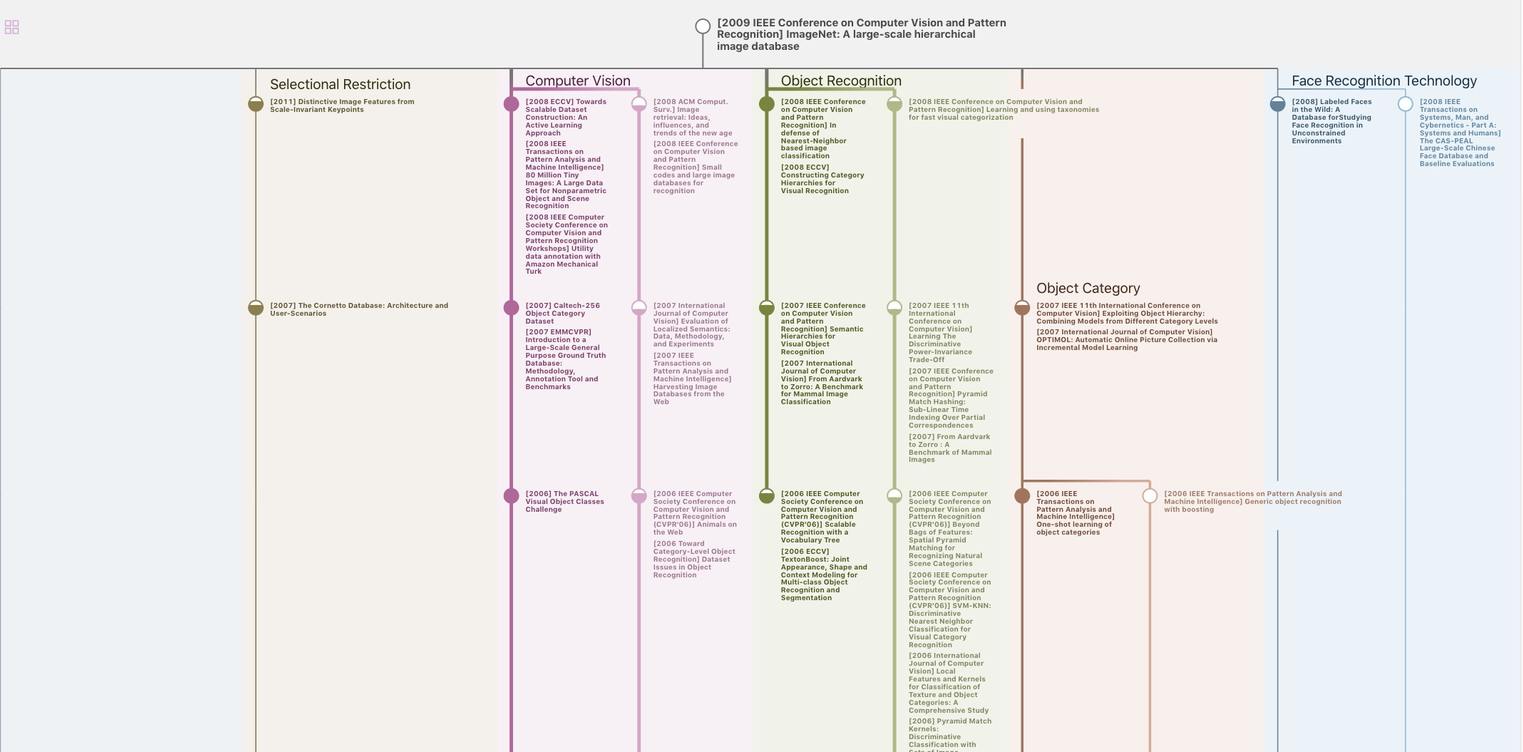
Generate MRT to find the research sequence of this paper
Chat Paper
Summary is being generated by the instructions you defined