Comparing artificial intelligence strategies for early sepsis detection in the ICU: an experimental study
Applied Intelligence(2023)
摘要
Sepsis is a life-threatening condition whose early recognition is key to improving outcomes for patients in intensive care units (ICUs). Artificial intelligence can play a crucial role in mining and exploiting health data for sepsis prediction. However, progress in this field has been impeded by a lack of comparability across studies. Some studies do not provide code, and each study independently processes a dataset with large numbers of missing values. Here, we present a comparative analysis of early sepsis prediction in the ICU by using machine learning (ML) algorithms and provide open-source code to the community to support future work. We reviewed the literature and conducted two phases of experiments. In the first phase, we analyzed five imputation strategies for handling missing data in a clinical dataset (which is often sampled irregularly and requires hand-crafted preprocessing steps). We used the MIMIC-III dataset, which includes more than 5,800 ICU hospital admissions from 2001 to 2012. In the second phase, we conducted an extensive experimental study using five ML methods and five popular deep learning models. We evaluated the performance of the methods by using the area under the precision-recall curve, a standard metric for clinical contexts. The deep learning methods (TCN and LSTM) outperformed the other methods, particularly in early detection tasks more than 4 hours before sepsis onset. The motivation for this work was to provide a benchmark framework for future research, thus enabling advancements in this field.
更多查看译文
关键词
Sepsis,Early prediction,Onset,Machine learning,Deep learning,Comparative study
AI 理解论文
溯源树
样例
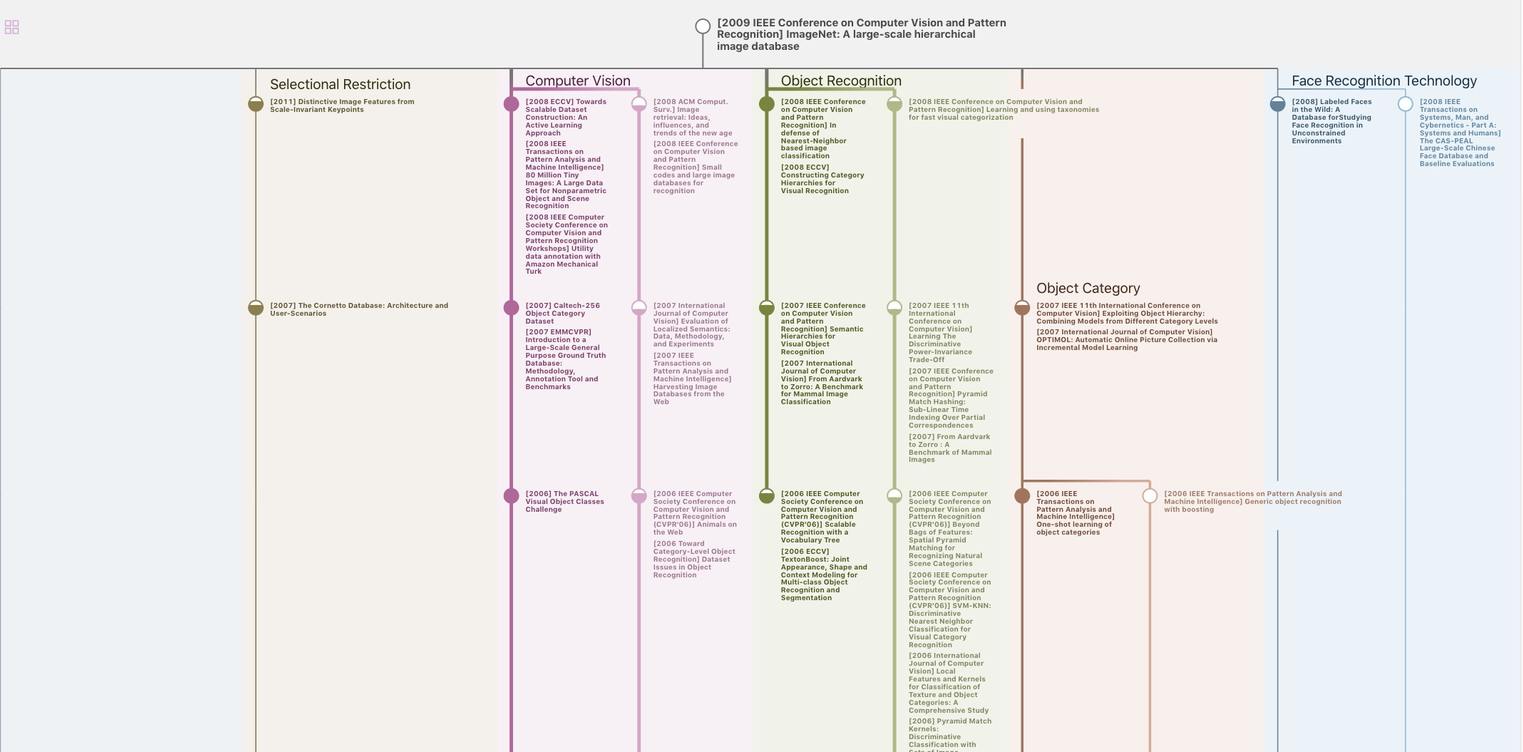
生成溯源树,研究论文发展脉络
Chat Paper
正在生成论文摘要