SCD:Sampling-based Class Distribution for Imbalanced Semi-Supervised Learning
2023 IEEE 35TH INTERNATIONAL CONFERENCE ON TOOLS WITH ARTIFICIAL INTELLIGENCE, ICTAI(2023)
摘要
Currently, many methods in the field of Semi-Supervised Learning (SSL) assume that the datasets are balanced, but imbalanced datasets are very common in real scenarios. To solve the imbalance problem, many methods require prior knowledge of the class distribution of the entire dataset, so as to use the prior distribution to guide the model training on the unlabeled data. These methods often assume that the unlabeled data distribution is uniform or identical to the labeled data distribution, which may differ from the real scenario distribution. In this work, we provide a new perspective for setting the class distribution for imbalanced SSL. Without assuming the unlabeled data distribution in advance, we propose to record the model’s sampling frequency results for each class as the sampling distribution of the whole data, which can reflect the model’s true sampling situation. Based on this, we adopt logits adjustment method to deal with the imbalance problem of sampled data. To utilize more pseudo-labels, we further adjust the threshold according to the class distribution. The final experiments show that our method achieves excellent performance in various imbalanced semi-supervised settings.
更多查看译文
关键词
Class-imbalanced Learning,Semi-Supervised Learning(SSL),Computer Vision
AI 理解论文
溯源树
样例
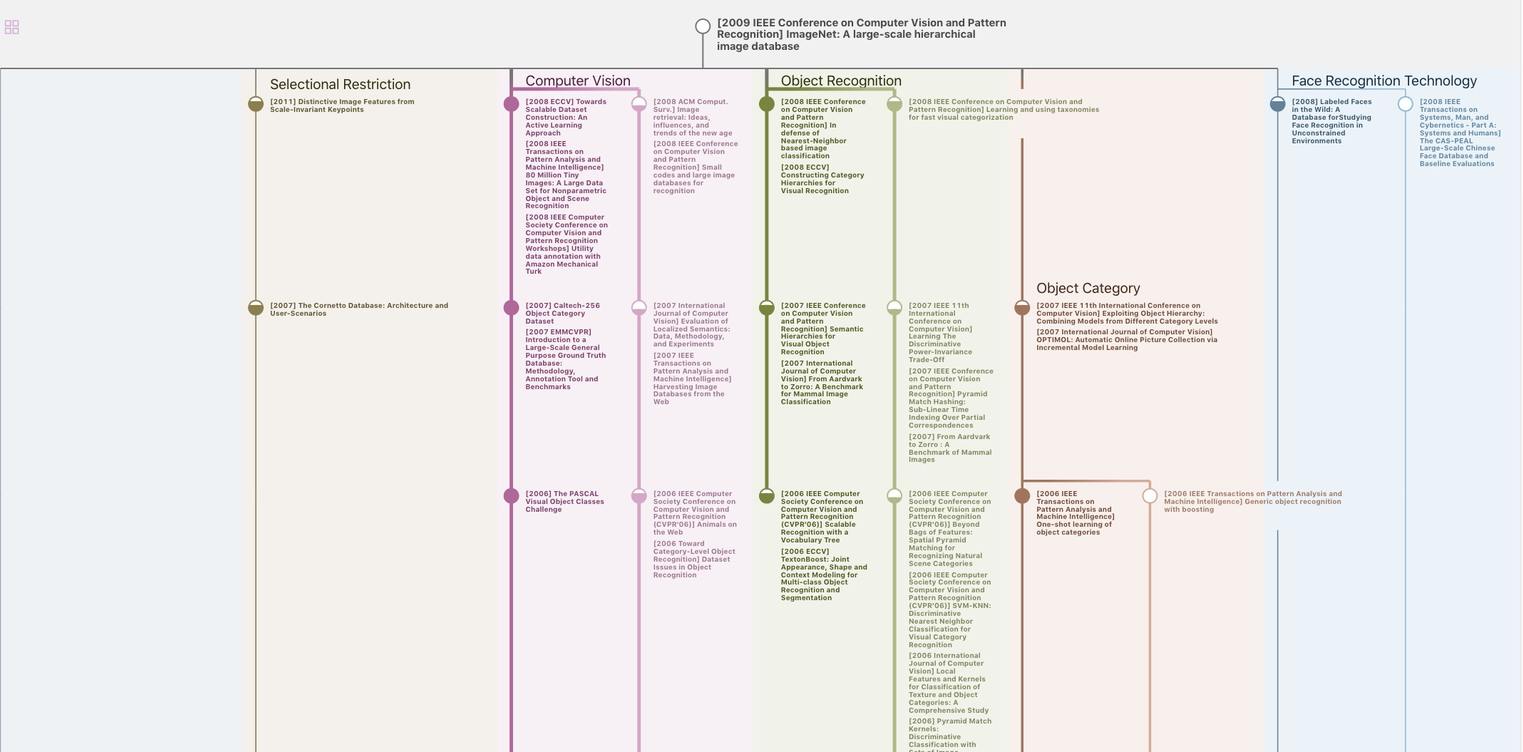
生成溯源树,研究论文发展脉络
Chat Paper
正在生成论文摘要