MARML: Motif-Aware Deep Representation Learning in Multilayer Networks
IEEE TRANSACTIONS ON NEURAL NETWORKS AND LEARNING SYSTEMS(2023)
摘要
The rapid increase in high-throughput, complex, and heterogeneous data has led to the adoption of network-structured models and analyses for interpretation. However, these data are inherently complex and challenging to understand, prompting researchers to turn to graph embedding methods to facilitate analysis. While general network embedding techniques have shown promise in improving downstream prediction and classification tasks, real-world data are complicated due to cross-domain interactions between different types of entities. Multilayered networks have been successful in integrating biological data to represent biological systems' hierarchy, but embedding nodes based on different types of interactions remains an unsolved problem. To address this challenge, we propose the Motif-aware deep representation learning in multilayer (MARML) networks for learning network representations. Our method considers recurring motif patterns, topological information, and attributive information from other sources as node features. We validated the MARML method using various multilayer network datasets. In addition, by incorporating motif information, MARML considers higher order connections across different hierarchies. The learned features exhibited excellent accuracy in tasks related to link prediction and link differentiation, enabling us to distinguish between existing and disconnected triplets. Through the integration of both intrinsic node attributes and topological network structures, we enhance our understanding of complex biological systems.
更多查看译文
关键词
Drug networks,motif embedding,multilayer network,network embedding
AI 理解论文
溯源树
样例
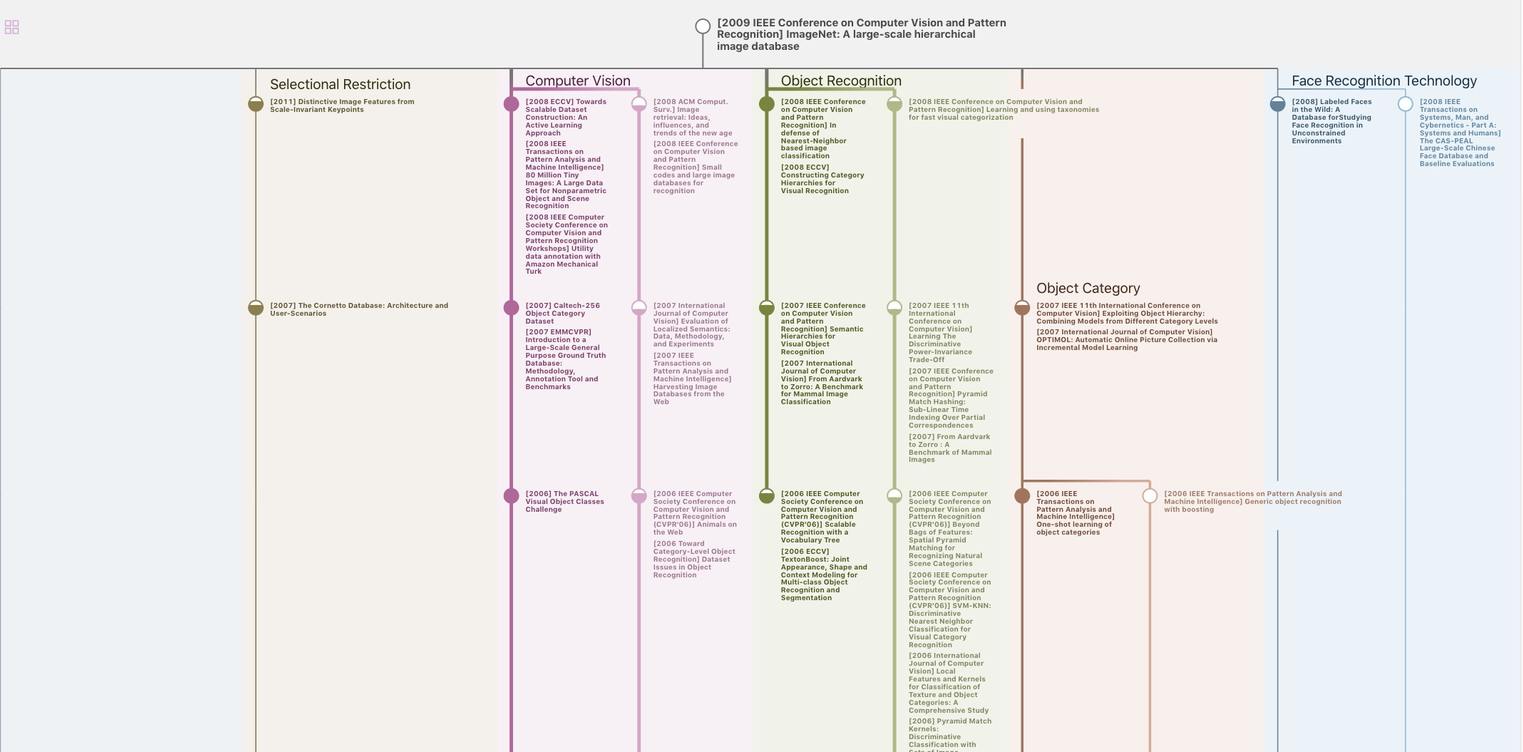
生成溯源树,研究论文发展脉络
Chat Paper
正在生成论文摘要