Low-Latency ML Inference by Grouping Correlated Data Objects and Computation
CoRR(2023)
摘要
ML inference workflows often require low latency and high throughput, yet we lack good options for addressing this need. Techniques that reduce latency in other streaming settings (such as caching and optimization-driven scheduling) are of limited value because ML data dependencies are often very large and can change dramatically depending on the triggering event. In this work, we propose a novel correlation grouping mechanism that makes it easier for developers to express application-specific data access correlations, enabling coordinated management of data objects in server clusters hosting streaming inference tasks. Experiments based on a latency-sensitive ML-based application confirm the limitations of standard techniques while showing that our solution yields dramatically better performance. The proposed mechanism is able to maintain significantly lower and more consistent latency, achieves higher node utilization as workload and scale-out increase, and yet requires only minor changes to the code implementing the application.
更多查看译文
AI 理解论文
溯源树
样例
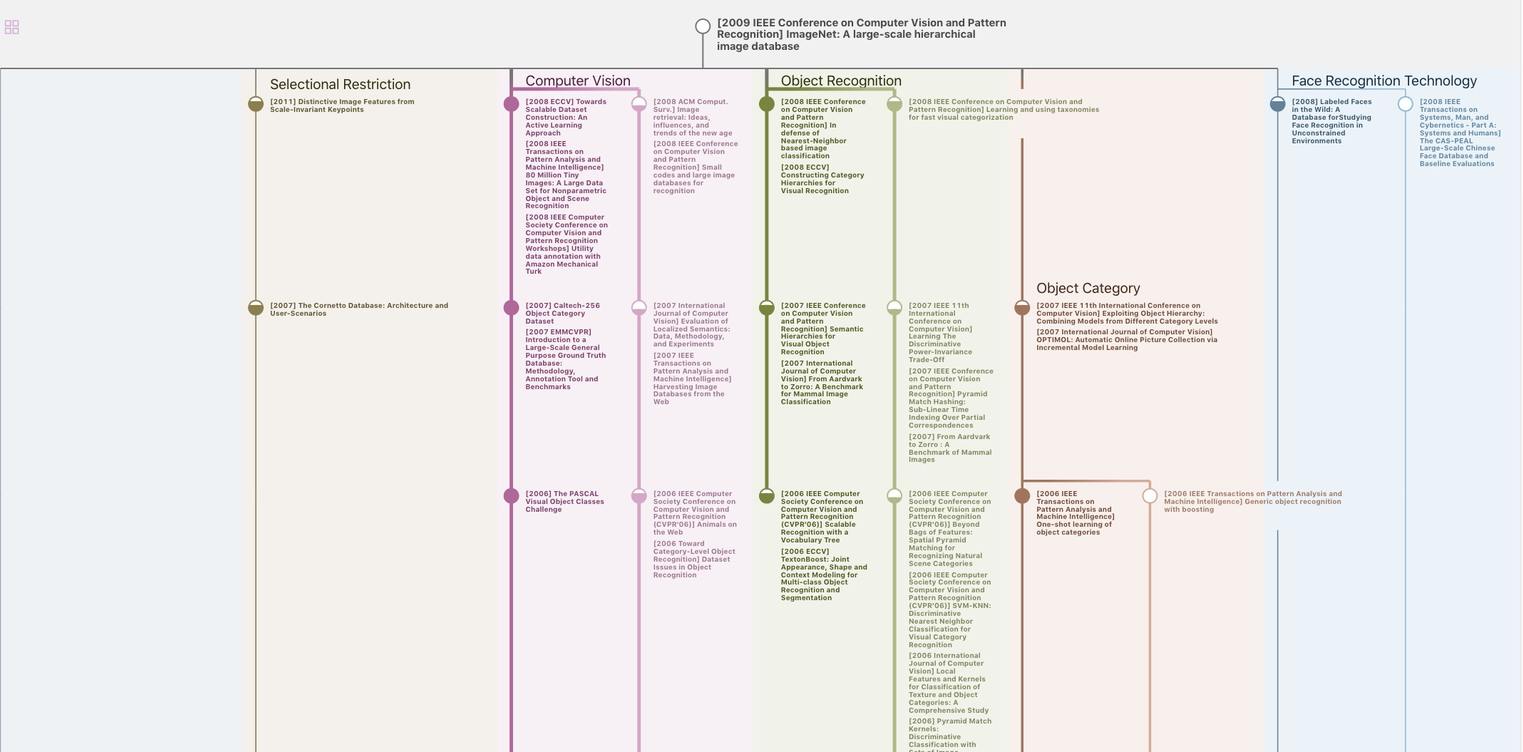
生成溯源树,研究论文发展脉络
Chat Paper
正在生成论文摘要