Unlearnable Games and "satisficing” Decisions: A Simple Model for a Complex World
arXiv (Cornell University)(2023)
摘要
As a schematic model of the complexity economic agents are confronted with, we introduce the “SK-game”, a discrete time binary choice model inspired from mean-field spin-glasses. We show that even in a completely static environment, agents are unable to learn collectively-optimal strategies. This is either because the learning process gets trapped in a sub-optimal fixed point, or because learning never converges and leads to a never ending evolution of agents intentions. Contrarily to the hope that learning might save the standard “rational expectation” framework in economics, we argue that complex situations are generically unlearnable and agents must do with satisficing solutions, as argued long ago by Herbert Simon (Simon 1955). Only a centralized, omniscient agent endowed with enormous computing power could qualify to determine the optimal strategy of all agents. Using a mix of analytical arguments and numerical simulations, we find that (i) long memory of past rewards is beneficial to learning whereas over-reaction to recent past is detrimental and leads to cycles or chaos; (ii) increased competition destabilizes fixed points and leads first to chaos and, in the high competition limit, to quasi-cycles; (iii) some amount of randomness in the learning process, perhaps paradoxically, allows the system to reach better collective decisions; (iv) non-stationary, “aging” behaviour spontaneously emerge in a large swath of parameter space of our complex but static world. On the positive side, we find that the learning process allows cooperative systems to coordinate around satisficing solutions with rather high (but markedly sub-optimal) average reward. However, hyper-sensitivity to the game parameters makes it impossible to predict ex ante who will be better or worse off in our stylized economy.
更多查看译文
关键词
Complex Systems,Interdisciplinary Physics,Statistical Physics
AI 理解论文
溯源树
样例
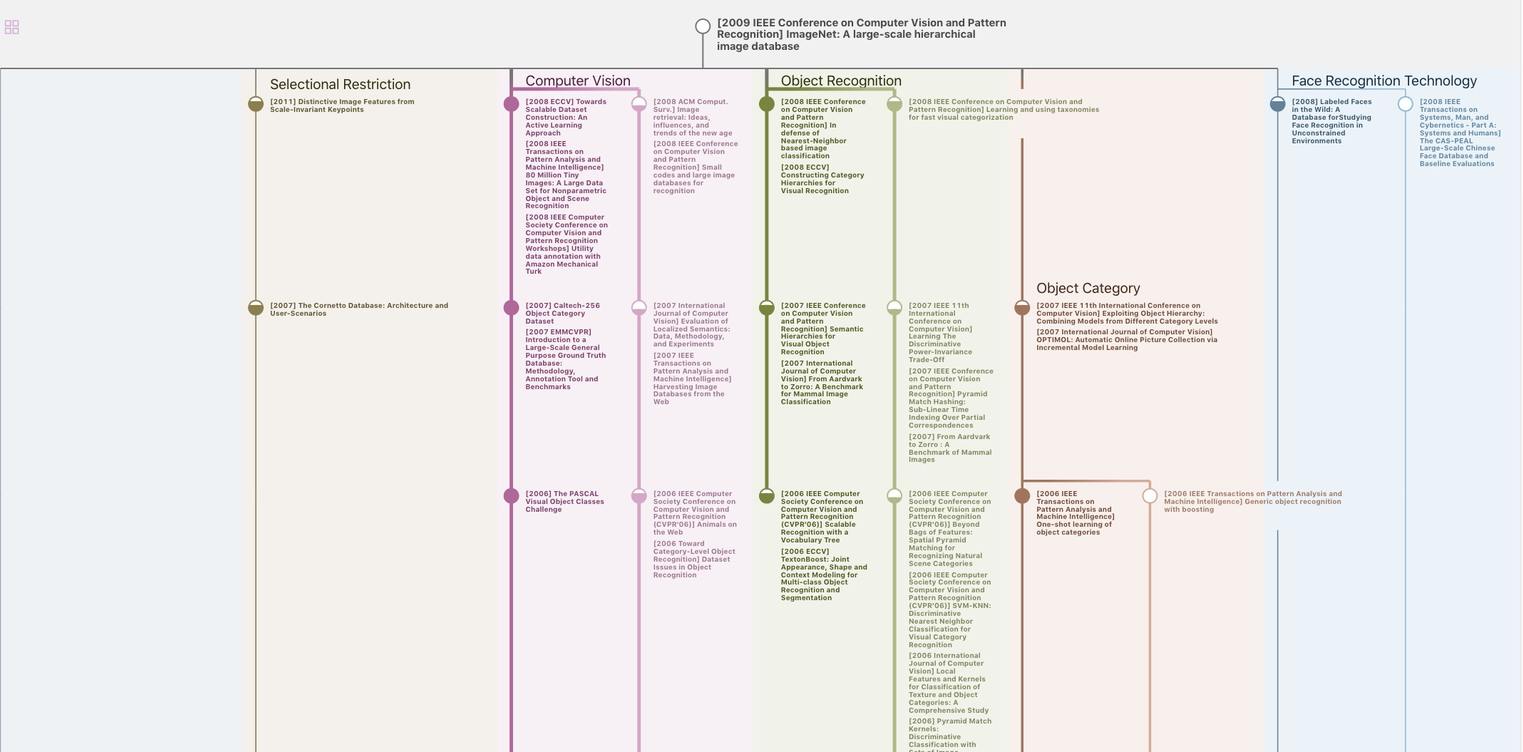
生成溯源树,研究论文发展脉络
Chat Paper
正在生成论文摘要