Cell Nuclei Segmentation With Dynamic Token-Based Attention Network
2023 45TH ANNUAL INTERNATIONAL CONFERENCE OF THE IEEE ENGINEERING IN MEDICINE & BIOLOGY SOCIETY, EMBC(2023)
摘要
Cell nuclei segmentation is crucial for analyzing cell structure in different tasks, i.e., cell identification, classification, etc., to treat various diseases. Several convolutional neural network-based architectures have been proposed for segmenting cell nuclei. Although these methods show superior performance, they lack the ability to predict reliable masks when using biomedical image data. This paper proposes a novel Dynamic Token-based Attention Network (DTA-Net). Combining convolutional neural networks (CNN) with a vision transformer (ViT) allows us to capture detailed spatial information from images efficiently by encoding local and global features. Dynamic Token-based Attention (DTA) module calculates attention maps keeping the overall computational and training costs minimal. For the nuclei segmentation task on the 2018 Science Bowl dataset, our proposed method outperformed SOTA networks with the highest Dice similarity score (DSC) of 93.02% and Intersection over Union (IoU) of 87.91% without using image pre- or post-processing techniques. The results showed that high-quality segmentation masks could be obtained by configuring a ViT in the most straight forward manner.
更多查看译文
AI 理解论文
溯源树
样例
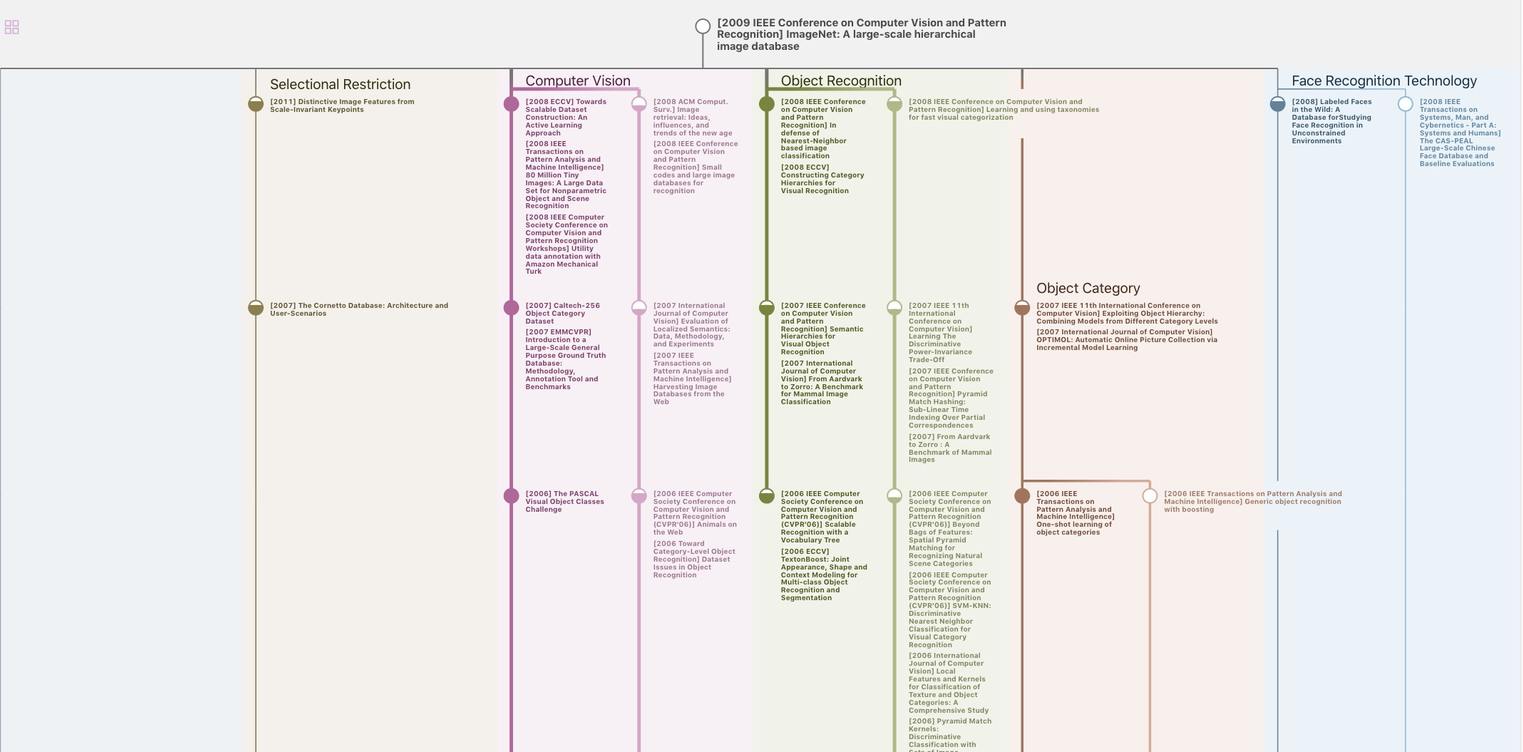
生成溯源树,研究论文发展脉络
Chat Paper
正在生成论文摘要