Multi-level graph learning for audio event classification and human-perceived annoyance rating prediction
CoRR(2023)
摘要
WHO's report on environmental noise estimates that 22 M people suffer from
chronic annoyance related to noise caused by audio events (AEs) from various
sources. Annoyance may lead to health issues and adverse effects on metabolic
and cognitive systems. In cities, monitoring noise levels does not provide
insights into noticeable AEs, let alone their relations to annoyance. To create
annoyance-related monitoring, this paper proposes a graph-based model to
identify AEs in a soundscape, and explore relations between diverse AEs and
human-perceived annoyance rating (AR). Specifically, this paper proposes a
lightweight multi-level graph learning (MLGL) based on local and global
semantic graphs to simultaneously perform audio event classification (AEC) and
human annoyance rating prediction (ARP). Experiments show that: 1) MLGL with
4.1 M parameters improves AEC and ARP results by using semantic node
information in local and global context aware graphs; 2) MLGL captures
relations between coarse and fine-grained AEs and AR well; 3) Statistical
analysis of MLGL results shows that some AEs from different sources
significantly correlate with AR, which is consistent with previous research on
human perception of these sound sources.
更多查看译文
关键词
Noise,annoyance,audio event classification,annoyance rating prediction,multi-level graph learning
AI 理解论文
溯源树
样例
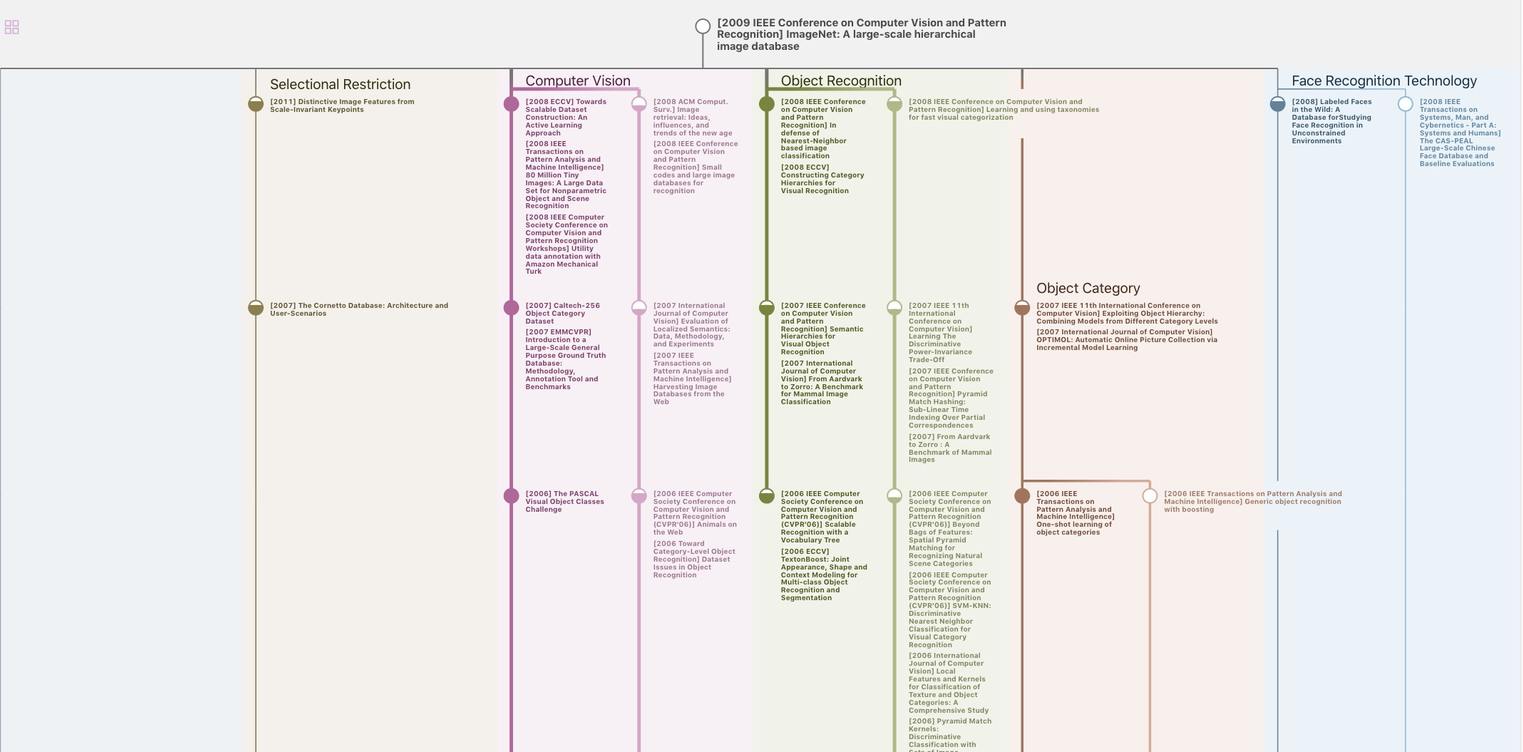
生成溯源树,研究论文发展脉络
Chat Paper
正在生成论文摘要