Robustness Verification of Deep Reinforcement Learning Based Control Systems Using Reward Martingales
AAAI 2024(2024)
摘要
Deep Reinforcement Learning (DRL) has gained prominence as an effective approach for control systems. However, its practical deployment is impeded by state perturbations that can severely impact system performance. Addressing this critical challenge requires robustness verification about system performance, which involves tackling two quantitative questions: (i) how to establish guaranteed bounds for expected cumulative rewards, and (ii) how to determine tail bounds for cumulative rewards. In this work, we present the first approach for robustness verification of DRL-based control systems by introducing reward martingales, which offer a rigorous mathematical foundation to characterize the impact of state perturbations on system performance in terms of cumulative rewards. Our verified results provide provably quantitative certificates for the two questions. We then show that reward martingales can be implemented and trained via neural networks, against different types of control policies. Experimental results demonstrate that our certified bounds tightly enclose simulation outcomes on various DRL-based control systems, indicating the effectiveness and generality of the proposed approach.
更多查看译文
关键词
PEAI: Safety, Robustness & Trustworthiness
AI 理解论文
溯源树
样例
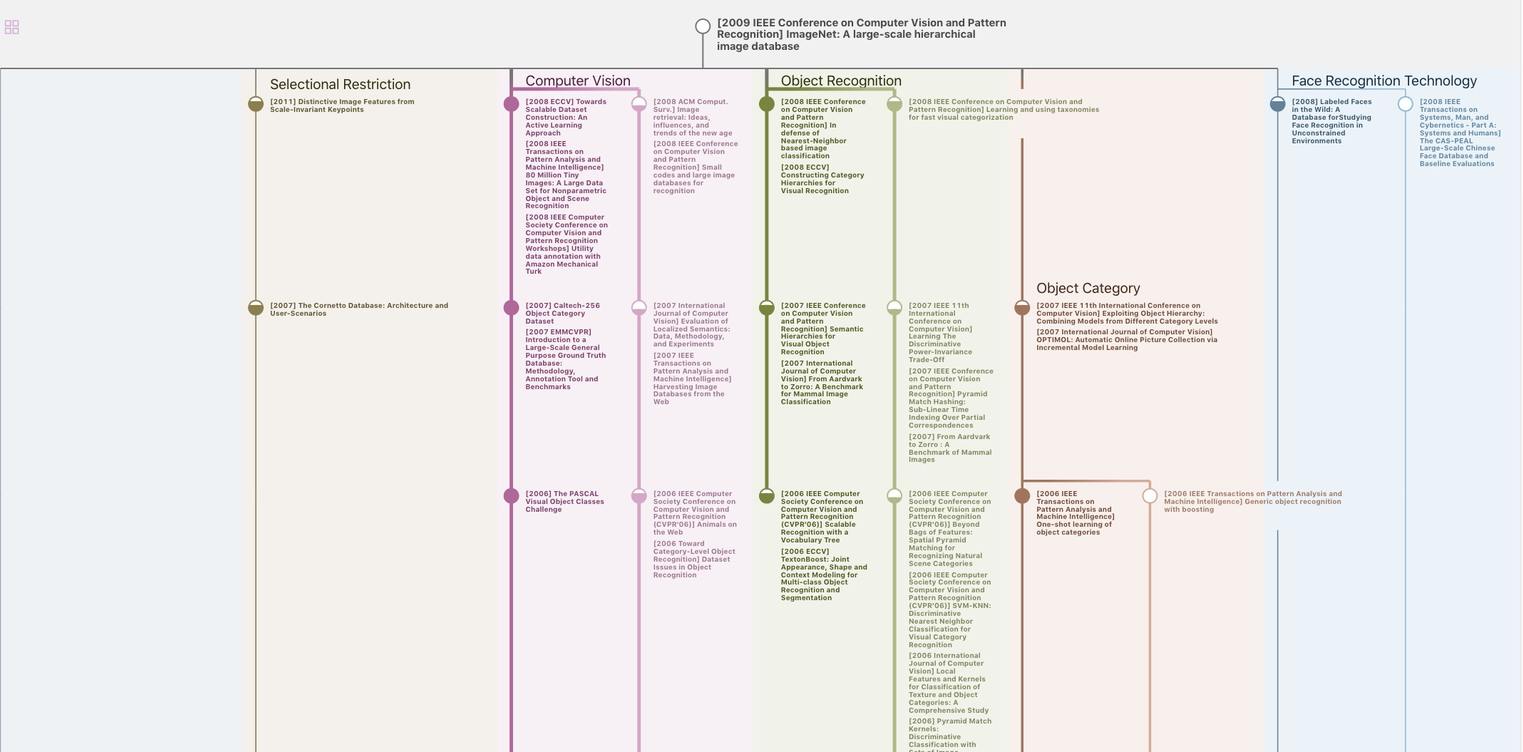
生成溯源树,研究论文发展脉络
Chat Paper
正在生成论文摘要