Accelerating Reliability-Based Topology Optimization Via Gradient Online Learning and Prediction
Aerospace science and technology(2024)
摘要
In reliability-based topology optimization (RBTO), both quantification and maximization of structure reliability considering uncertainty of various quantities are essential. In this study, A novel gradient online learning and prediction (GoLap) method is proposed to accelerate the double-looped RBTO and reduce total computational time by using a one-hidden-layer neural networks (NN) and gradient and non-gradient based reliability quantification. The total computational time saving is achieved by (i) skipping the finite element analysis (FEA) runs and sensitivity analyses and (ii) updating the design and random variables using the gradients predicted by the NN model in selected inner and outer loops. 2D and 3D numerical examples, including an aircraft engine pylon, are presented to demonstrate the total computational time saving of up to 84.4%, and the prediction accuracy of the reliability of optimum structures for the GoLap implemented RBTO methods.
更多查看译文
关键词
reliability -based topology optimization,neural networks,machine learning
AI 理解论文
溯源树
样例
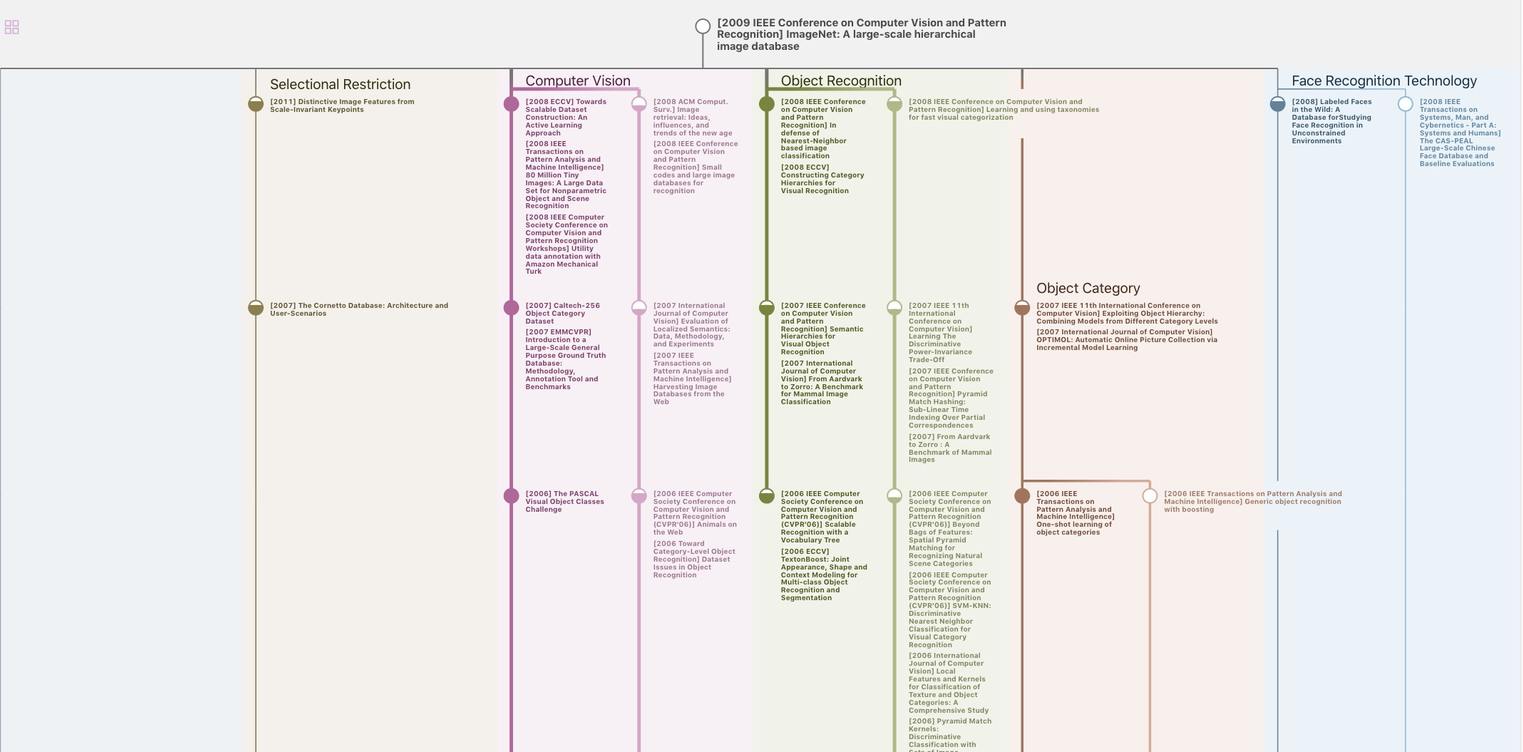
生成溯源树,研究论文发展脉络
Chat Paper
正在生成论文摘要