Regularization Based Incremental Learning in TCNN for Robust Speech Enhancement Targeting Effective Human Machine Interaction
SPEECH AND COMPUTER, SPECOM 2023, PT I(2023)
摘要
In general, the performance of deep learning based speech enhancement degrades in presence of unseen noisy environments for any signal-to-noise ratio (SNR) conditions. Although model adaptation techniques may help in improving the performance, they lead to catastrophic forgetting of the previously learned knowledge. Under such conditions, incremental learning or life-long learning has been reported to help in gradually learning the new tasks while maintaining the existing inferred knowledge. In this work, we propose a regularization-based incremental learning strategy for adapting temporal convolutional neural network (TCNN) based speech enhancement novel framework named as RIL-TCN. We investigate the effect of incorporating various weight regularization strategies such as curvature and path regularization on time-domain Scale-Invariant SNR (SI-SNR) loss function associated with TCNN based enhancement framework. We evaluate and compare the performance of our proposed model with the state-of-the-art frequency domain incremental learning model using objective measures such as SI-SNR and PESQ (Perceptual Evaluation of Speech Quality). We show that our proposed approach outperforms on unseen noises from standard CHiME-3 corpus compared to competitive TCNN baseline.
更多查看译文
关键词
Speech Enhancement,Incremental Learning,Life-Long Learning,Adaptation,TCN
AI 理解论文
溯源树
样例
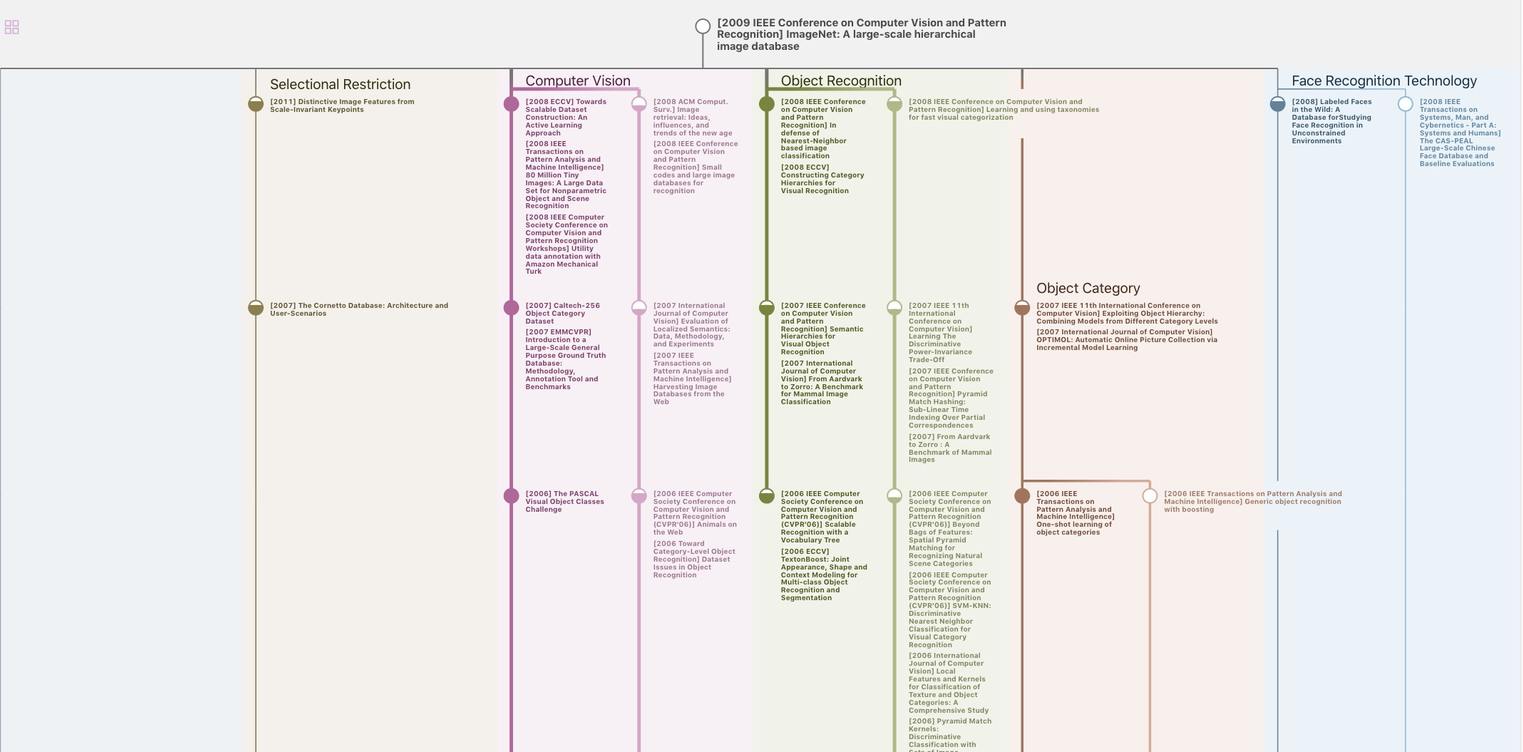
生成溯源树,研究论文发展脉络
Chat Paper
正在生成论文摘要