A comparison review of transfer learning and self-supervised learning: Definitions, applications, advantages and limitations
EXPERT SYSTEMS WITH APPLICATIONS(2024)
摘要
Deep learning has emerged as a powerful tool in various domains, revolutionising machine learning research. However, one persistent challenge is the scarcity of labelled training data, which hampers the performance and generalisation of deep learning models. To address this limitation, researchers have developed innovative methods to overcome data scarcity and enhance deep model learning capabilities. Two prevalent techniques that have gained significant attention are transfer learning and self-supervised learning. Transfer learning leverages knowledge learned from pre-training on a large-scale dataset, such as ImageNet, and applies it to a target task with limited labelled data. This approach allows models to benefit from the learned representations and effectively transfer knowledge to new tasks, resulting in improved learning performance and generalisation. On the other hand, self-supervised learning focuses on training models using pretext tasks that do not require manual annotation, allowing them to learn valuable representations from large amounts of unlabelled data. These learned representations can then be fine-tuned for downstream tasks, mitigating the need for extensive labelled data. In recent years, transfer and self-supervised learning have found applications in various fields, including medical image processing, video recognition, and natural language processing. These approaches have demonstrated remarkable achievements, enabling breakthroughs in areas such as disease diagnosis, object recognition, and language understanding. However, while these methods offer numerous advantages, they also have limitations. For example, transfer learning may face domain mismatch issues between the pre-training and target domains, while self-supervised learning requires careful design of pretext tasks to ensure meaningful representations. This review paper explores the recent applications of these pre-training methods in various fields within the past three years. It delves into the advantages and limitations of each approach, assesses the performance of models employing these techniques, and identifies potential directions for future research. By providing a comprehensive review of current pre-training methods, this article offers guidance for selecting the best technique for specific deep learning applications to address the data scarcity issue.
更多查看译文
关键词
Deep learning,Data scarcity,Transfer learning,Self-supervised learning
AI 理解论文
溯源树
样例
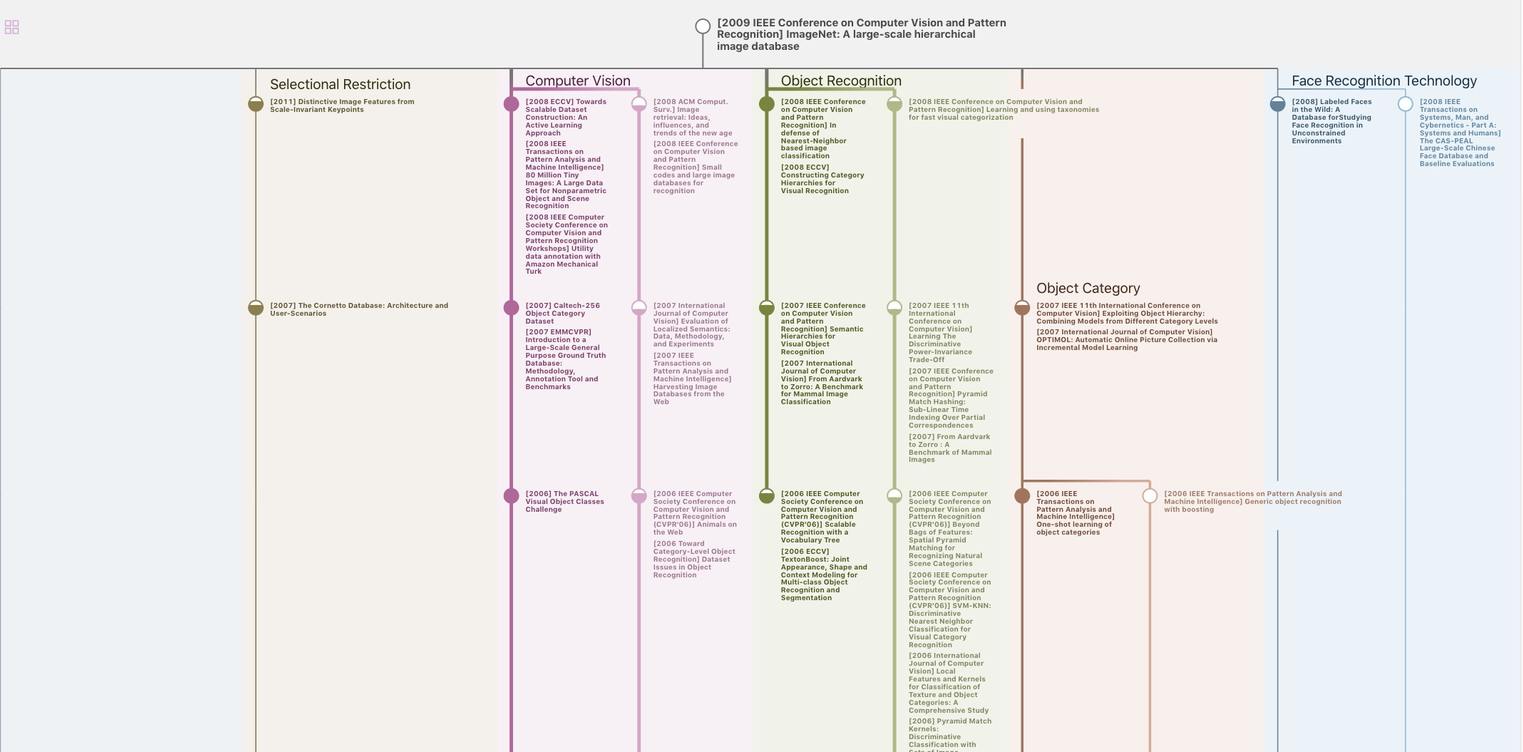
生成溯源树,研究论文发展脉络
Chat Paper
正在生成论文摘要