Fault detection using generalized autoencoder with neighborhood restriction for electrical drive systems of high-speed trains
CONTROL ENGINEERING PRACTICE(2024)
摘要
Over the past two decades, fault detection of high-speed trains has become an active issue in the transportation area. Recent work has demonstrated the benefits of autoencoder for fault detection in high-speed trains. The latent variable of autoencoder is generally viewed as features generated by the learning process, without explainable interpretation and assessment of the latent variable with respect to its information quality. In the context of representation learning, the latent variable of autoencoder plays an important role towards an fault detection. There is a lack of a methodical framework for explainable applications of AE-based technique to approach fault detection issues. This paper proposes a generalized autoencoder based on neighborhood restriction (NRGAE) to minimize the information loss caused by data compression and reconstruction. The main objective of this paper is to establish a framework for learning autoencoder-based fault detection in the electrical drive systems of high-speed trains. Meanwhile, this work introduces the concepts of mutual information and local linear embedding for electrical drive systems and fault detection specifications. A case study on an electrical drive system is given to illustrate the capability of proposed fault detection.
更多查看译文
关键词
Fault detection,Electrical drive systems,High-speed trains,Generalized autoencoder,Mutual information
AI 理解论文
溯源树
样例
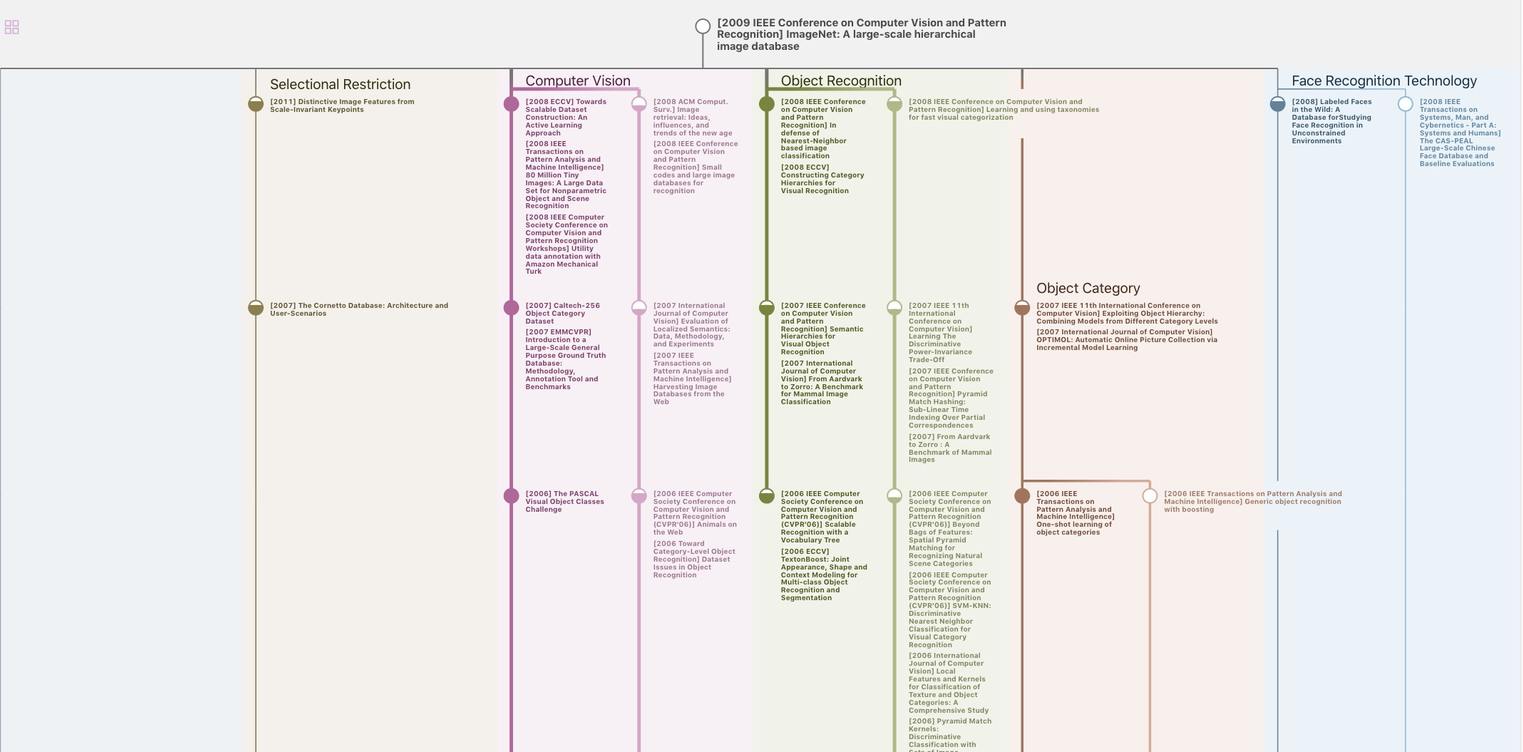
生成溯源树,研究论文发展脉络
Chat Paper
正在生成论文摘要