Global Latent Neural Rendering.
2024 IEEE/CVF Conference on Computer Vision and Pattern Recognition (CVPR)(2024)
摘要
A recent trend among generalizable novel view synthesis methods is to learn a rendering operator acting over single camera rays. This approach is promising because it removes the need for explicit volumetric rendering, but it effectively treats target images as collections of independent pixels. Here, we propose to learn a global rendering operator acting over all camera rays jointly. We show that the right representation to enable such rendering is the 5-dimensional plane sweep volume, consisting of the projection of the input images on a set of planes facing the target camera. Based on this understanding, we introduce our Convolutional Global Latent Renderer (ConvGLR), an efficient convolutional architecture that performs the rendering operation globally in a low-resolution latent space. Experiments on various datasets under sparse and generalizable setups show that our approach consistently outperforms existing methods by significant margins.
更多查看译文
关键词
General Method,Input Image,Target Image,Latent Space,Convolutional Architecture,Set Of Planes,View Synthesis,Transformer,Image Features,Deep Features,Image Patches,Light Field,Density Field,Latent Representation,Masked Images,Angular Distance,Light Rays,Representation Layer,Positional Encoding,Scene Geometry,Cost Volume,Alpha Matte,Depth Planes,Geometric Reasoning,Depth Dimension,Target View,Training Scenes,Multi-view Stereo,Set Of Views
AI 理解论文
溯源树
样例
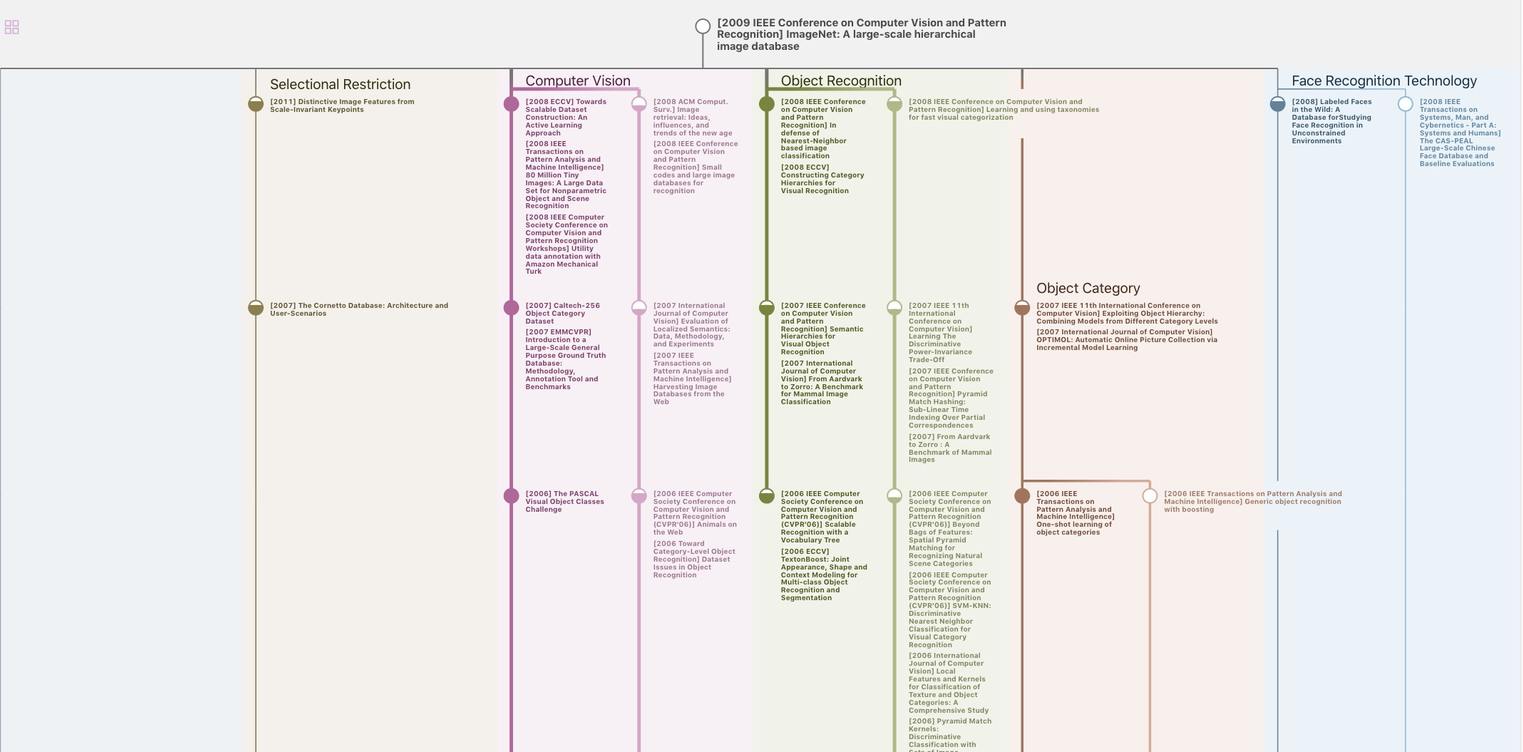
生成溯源树,研究论文发展脉络
Chat Paper
正在生成论文摘要