Unsupervised End-to-End Transformer based approach for Video Anomaly Detection.
2023 38th International Conference on Image and Vision Computing New Zealand (IVCNZ)(2023)
摘要
Anomaly detection in videos is a challenging task due to multiple constraints including the imbalanced nature of anomalies, limited annotated data, and limitation of existing supervised or semi-supervised algorithms to learn in such imbalanced situations. To overcome these issues, Transformer-based unsupervised learning may be a promising solution. In this paper, we proposed a generative transfer learning (GTL) algorithm for video anomaly detection using an unsupervised learning approach. This framework consists of three major parts: (1) a feature extractor; (2) a generator transformer; and (3) a discriminator transformer. The feature extractor generates spatio-temporal features from unlabeled input segments, and passes them to the generator transformer which tries to reconstruct these features. Using cooperative learning between the generator transformer and the discriminator transformer, we train our network so that anomalies have high reconstruction error and non-anomalies do not. We test our proposed model on two well-known anomaly detection datasets (UCF-Crime and ShangaiTech) and report state-of-the-art.
更多查看译文
AI 理解论文
溯源树
样例
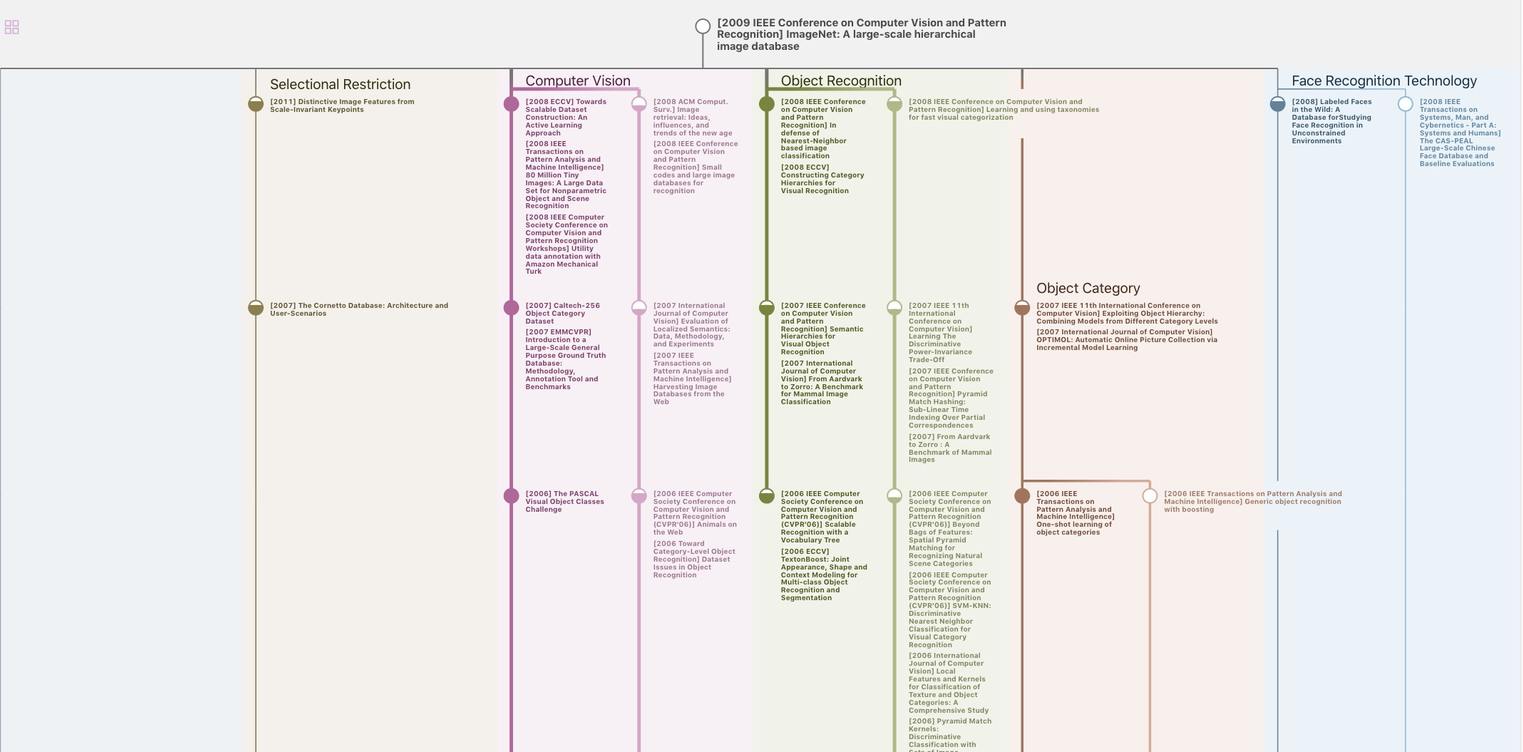
生成溯源树,研究论文发展脉络
Chat Paper
正在生成论文摘要