Quantifying the sampling error on burn counts in Monte-Carlo wildfire simulations using Poisson and Gamma distributions
Stochastic Environmental Research and Risk Assessment(2024)
摘要
This article provides a precise, quantitative description of the sampling error on burn counts in Monte-Carlo wildfire simulations - that is, the prediction variability introduced by the fact that the set of simulated fires is random and finite. We show that the marginal burn counts are (very nearly) Poisson-distributed in typical settings and infer through Bayesian updating that Gamma distributions are suitable summaries of the remaining uncertainty. In particular, the coefficient of variation of the burn count is equal to the inverse square root of its expected value, and this expected value is proportional to the number of simulated fires multiplied by the asymptotic burn probability. From these results, we derive practical guidelines for choosing the number of simulated fires and estimating the sampling error. Notably, the required number of simulated years is expressed as a power law. Such findings promise to relieve fire modelers of resource-consuming iterative experiments for sizing simulations and assessing their convergence: statistical theory provides better answers, faster.
更多查看译文
关键词
Wildland fire risk,Monte-Carlo simulations,Uncertainty quantification,Bayesian updating,Estimator variance,Statistical power analysis
AI 理解论文
溯源树
样例
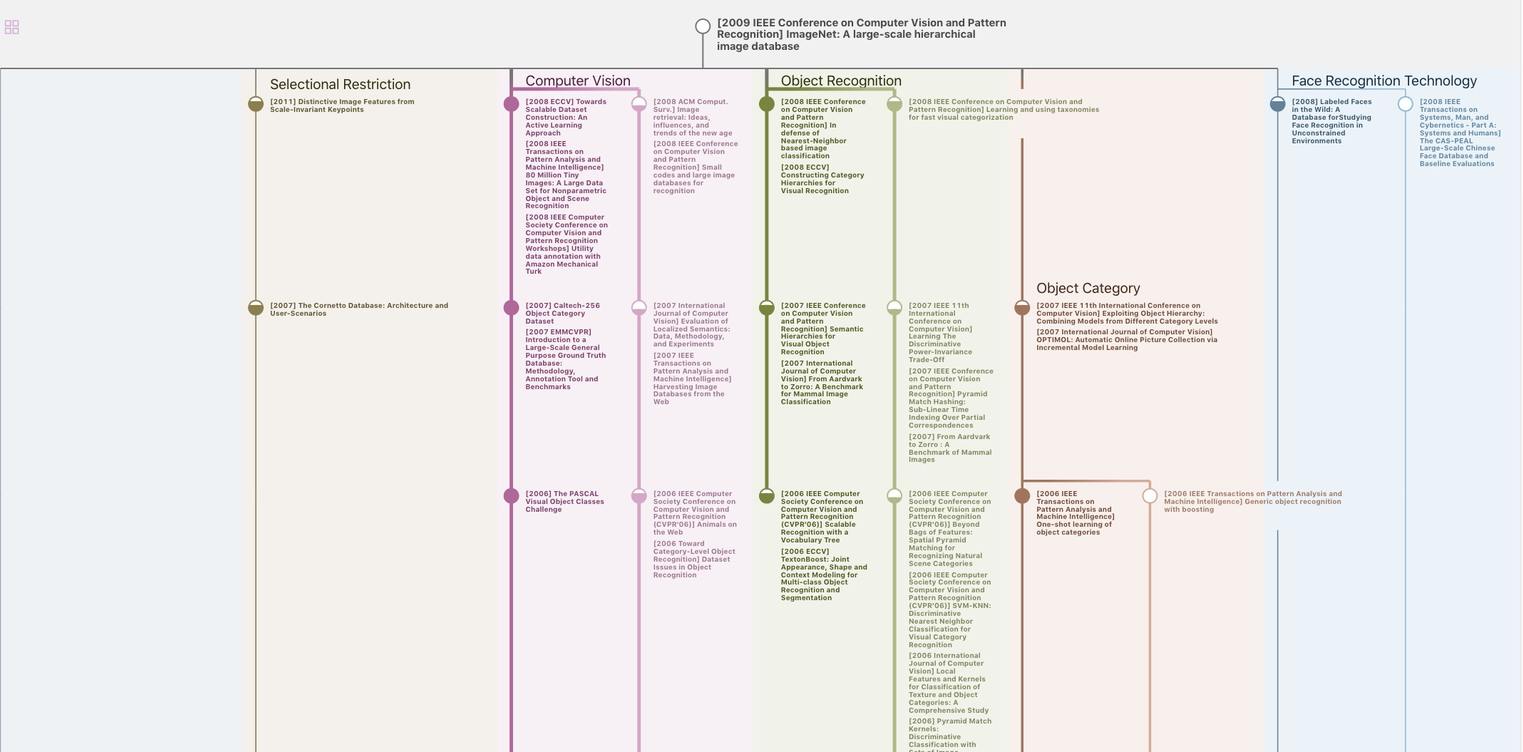
生成溯源树,研究论文发展脉络
Chat Paper
正在生成论文摘要