Surrogate Models Based on Back-Propagation Neural Network for Parameters Prediction of the PWR Core
Proceedings of the 23rd Pacific Basin Nuclear Conference, Volume 2 Springer Proceedings in Physics(2023)
摘要
Predicting the key neutronics parameters of reactor core accurately and efficiently is necessary during the reactor operation. On account of the nonlinearity and complexity of the reactor core, as well as the current development of computer, it still be difficult to solve the neutron transport equation accurately in real time. The neural network, which has been widely used in the nuclear industry in recent years, has the ability to obtain the accurate numerical solution for complex nonlinear problem in real time. In this paper two non-intrusive surrogate models based on Back-propagation (BP) neural network are proposed to predict the core key parameters accurately and effectively. The proposed surrogate models are applied to the modified 2D C5G7 MOX benchmark problems with a quarter symmetric PWR core. The interested inputs are the Plutonium isotope contents in MOX fuel with different enrichment and the target outputs are the effective multiplication factor $$(k_{{\text{eff}}} )$$ and neutron pin-by-pin flux distribution. Then the hyper-parameters optimization is performed to improve the prediction performance of the surrogate models. The dataset is generated by using Monte Carlo code OpenMC through changing Plutonium isotope content in MOX fuel. The numerical results show that the predicted values of the surrogate models are in good agreement with the values calculated by the Monte Carlo code. Moreover, the desired outputs can be obtained in seconds through the surrogate models, which is much more efficient than traditional directly numerical methods. The work demonstrates the feasibility and advantage of the proposed surrogate models to predict the key neutronics parameters of the reactor core, which has the important significance for the real-time online prediction of key parameters and the optimization design of the reactor core.
更多查看译文
AI 理解论文
溯源树
样例
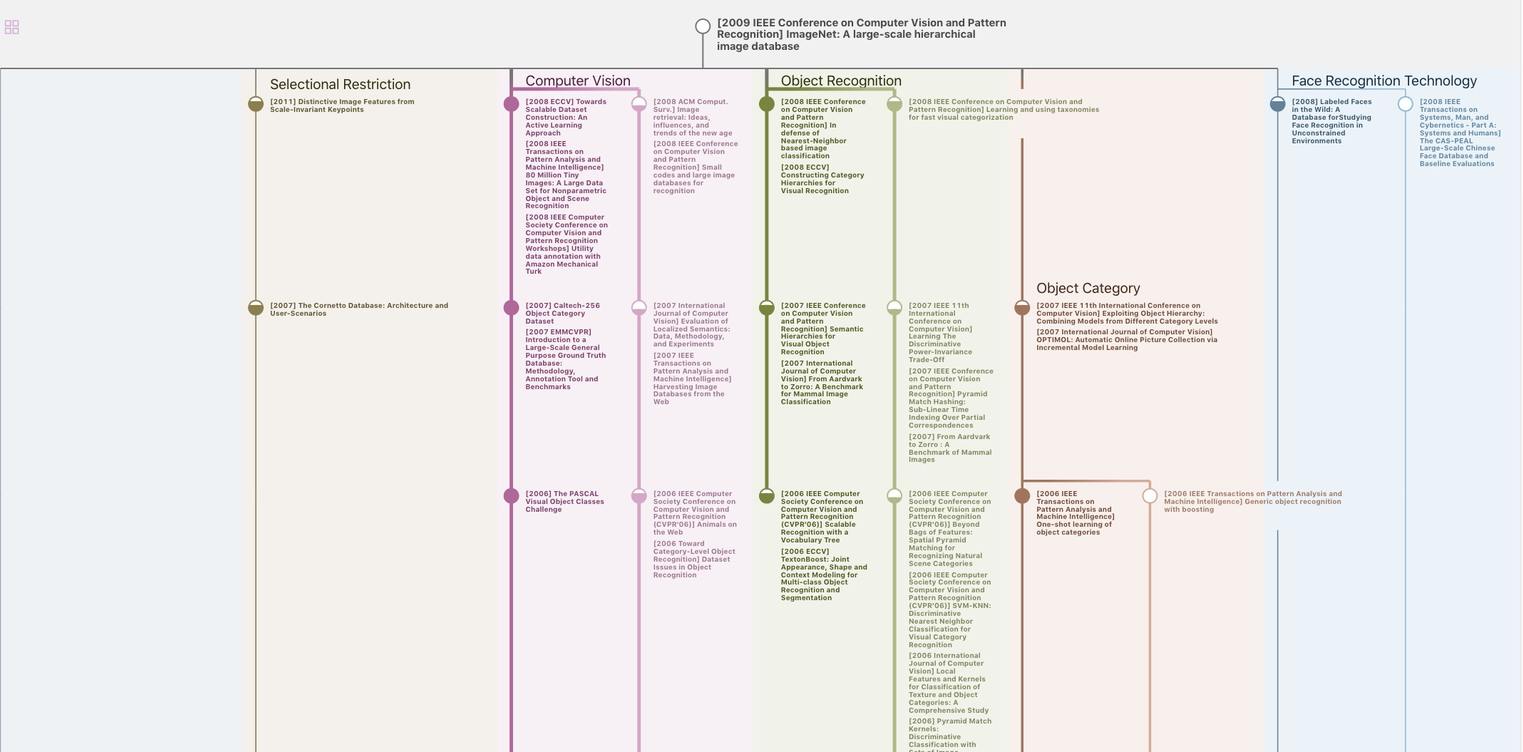
生成溯源树,研究论文发展脉络
Chat Paper
正在生成论文摘要