Data Assimilation Using Co-processors for Ocean Circulation
Lecture notes in mechanical engineering(2023)
摘要
Data Assimilation is a procedure for fusion from the observational system and previous forecasting to calculate the initial condition – also called analysis – for the next prediction cycle. Several methods have been developed and applied for data assimilation (DA). We can cite the Kalman filter, particle filter, and variational approach as methods employed for DA. However, the mentioned methods are computer intensive. One alternative to reduce the computational effort for DA is to apply a neural network (NN) to emulate a computationally expensive technique. The NN approach has been also applied for ensemble prediction to address uncertainty quantification for each forecasting cycle. A self-configuring framework was applied to design the best NN architecture to emulate the Kalman filter for DA, using the metaheuristic: Multiple Particles Collision Algorithm (MPCA). The optimal artificial neural network is implemented on two types of co-processors: FPGA (Field-Programmable Gate Array), and TPU (tensor processing unit). Shallow water 2D system is designed to simulate ocean circulation dynamics, where the finite difference scheme is used for numerical integration of the model. The artificial neural network was effective, with reduction of processing time. The use of FPGA or TPU as co-processors for data assimilation have similar precision in comparison with analysis calculated by software. The better processing time performance among multi-core CPU, FPGA, and TPU was obtained by the TPU when the number of grid points ( $$N \times N$$ ) is greater than 150. For $$N \le 150$$ , the CPU presented a smaller execution time.
更多查看译文
关键词
assimilation,co-processors
AI 理解论文
溯源树
样例
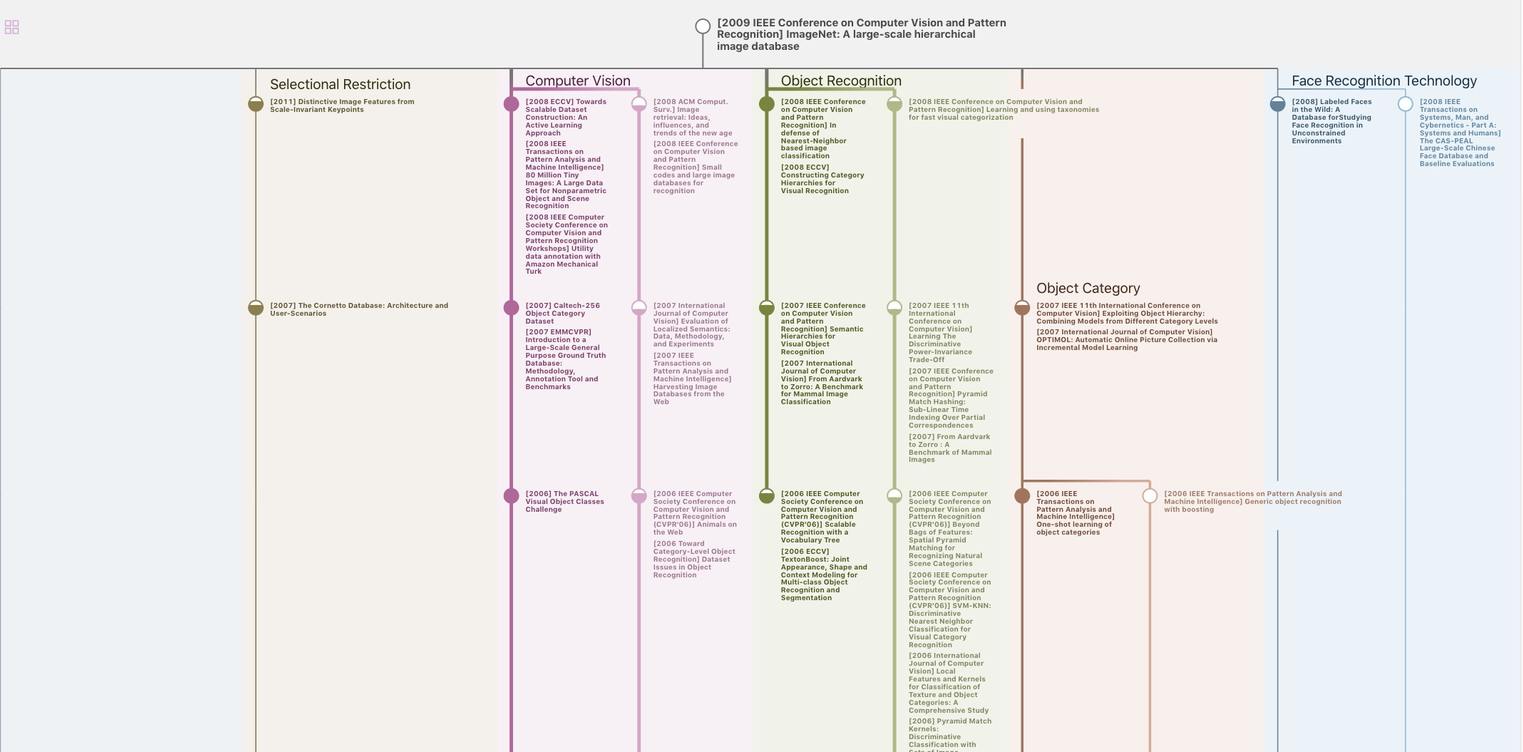
生成溯源树,研究论文发展脉络
Chat Paper
正在生成论文摘要