Current Practice and Unmet Training Needs in Robotic-Assisted Radical Prostatectomy: Investigation from the Junior ERUS / YAU Working Group
European Urology(2024)
AdventHealth Global Robotics Institute | The Royal Marsden NHS Foundation Trust | ASST Grande Ospedale Metropolitano Niguarda | ASST Santi Paolo e Carlo - La Statale University | OLV Hospital | University of Freiburg Medical Centre | University Hospital | Luzerner Kantonsspital | University of Florence | University of Turin | Iuliu Hatieganu University of Medicine and Pharmacy | Autonoma University of Barcelona at Fundacio Puigvert | San Raffaele Hospital
Abstract
Purpose To access the current scenario of robotic-assisted radical prostatectomy training in multiple centers worldwide. Methods We created a multiple-choice questionnaire assessing all details of robotic-assisted radical prostatectomy training with 41 questions divided into three different categories (responder demography, surgical steps, and responder experience). The questionnaire was created and disseminated using the “Google Docs” platform. All responders had an individual invitation by direct message or Email. We selected urologists who had recently finished a postgraduation urologic robotic surgery training (fellowship) in the last five years. We sent 624 invitations to urologists from 138 centers, from January 10th to April 10th, 2022. The answers were reported as percentages and illustrated in pie charts. Results The response rate was 58% among all centers invited (138/81), 20% among all individual invitations (122/624 answers). Globally, we gathered responses from 23 countries. Most surgeons were older than 34 years, 71% trained in an academic center, and 64% performed less than ten full RARP cases. Transperitoneal is the most common access, and 63% routinely opens the endopelvic fascia. Almost 90% perform the Rocco’s stitch, and 94% perform the anastomosis with barbed sutures. Finally, only 31% of surgeons assisted more than 100 cases before moving to the console, and most surgeons (63.9%) performed less than ten full RARP cases during their training. Conclusion By assessing the robotic-assisted radical prostatectomy training status in 23 countries and 81 centers worldwide, we assessed the trainees’ demography, step-by-step surgical technique, training perspectives, and impressions of surgeons who trained in the last five years. This data is crucial for a better understanding the trainee's standpoint, addressing potential deficiencies, and implementing improvements needed in the training process. Our study clearly indicates elements of current training modalities that are prone to major improvement.
MoreTranslated text
Key words
Robotic radical prostatectomy,Robotic surgery training,Robotic surgery fellowship,Prostate cancer
求助PDF
上传PDF
View via Publisher
AI Read Science
AI Summary
AI Summary is the key point extracted automatically understanding the full text of the paper, including the background, methods, results, conclusions, icons and other key content, so that you can get the outline of the paper at a glance.
Example
Background
Key content
Introduction
Methods
Results
Related work
Fund
Key content
- Pretraining has recently greatly promoted the development of natural language processing (NLP)
- We show that M6 outperforms the baselines in multimodal downstream tasks, and the large M6 with 10 parameters can reach a better performance
- We propose a method called M6 that is able to process information of multiple modalities and perform both single-modal and cross-modal understanding and generation
- The model is scaled to large model with 10 billion parameters with sophisticated deployment, and the 10 -parameter M6-large is the largest pretrained model in Chinese
- Experimental results show that our proposed M6 outperforms the baseline in a number of downstream tasks concerning both single modality and multiple modalities We will continue the pretraining of extremely large models by increasing data to explore the limit of its performance
Upload PDF to Generate Summary
Must-Reading Tree
Example
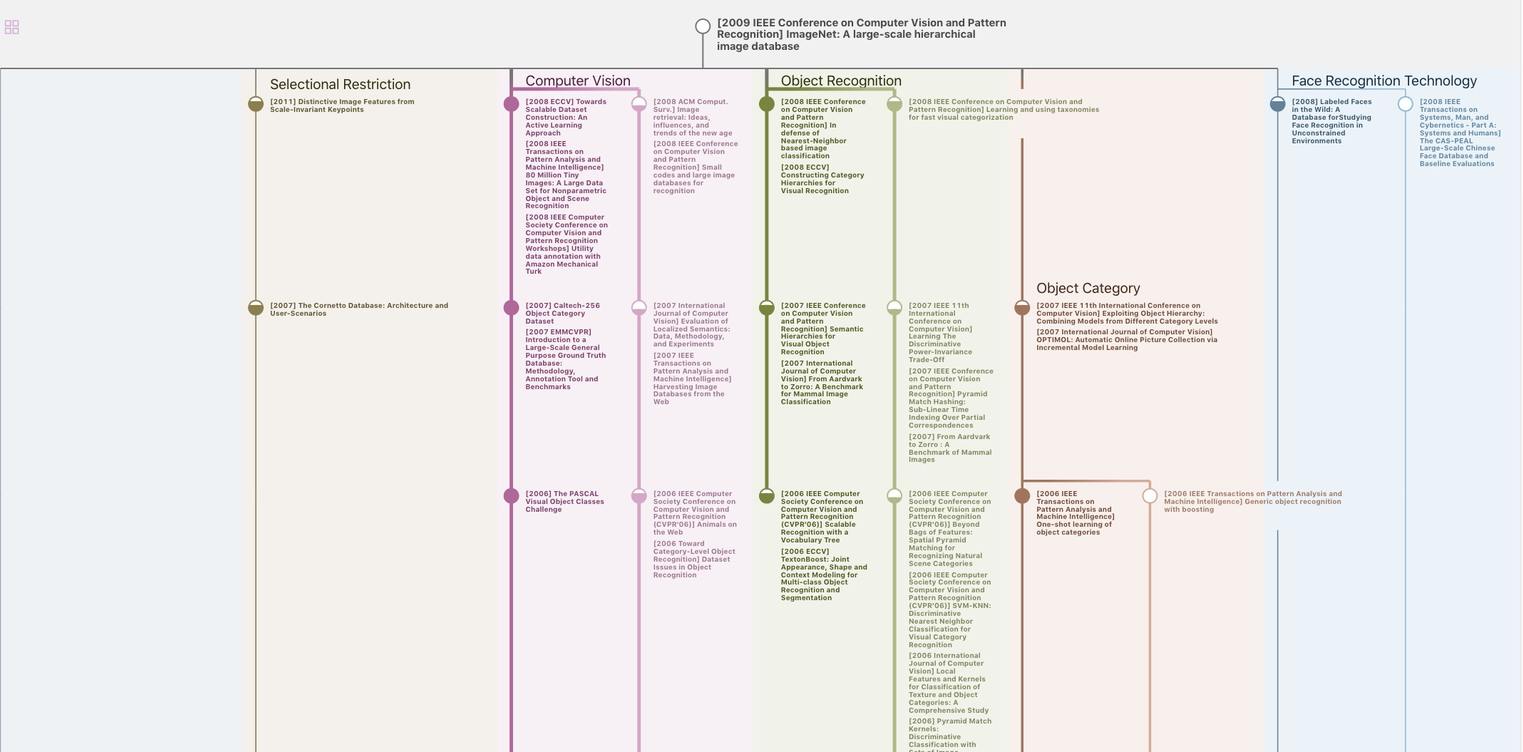
Generate MRT to find the research sequence of this paper
Related Papers
Data Disclaimer
The page data are from open Internet sources, cooperative publishers and automatic analysis results through AI technology. We do not make any commitments and guarantees for the validity, accuracy, correctness, reliability, completeness and timeliness of the page data. If you have any questions, please contact us by email: report@aminer.cn
Chat Paper