Multiple Imputation for Non-Monotone Missing Not at Random Data Using the No Self-Censoring Model
STATISTICAL METHODS IN MEDICAL RESEARCH(2023)
摘要
Although approaches for handling missing data from longitudinal studies are well-developed when the patterns of missingness are monotone, fewer methods are available for non-monotone missingness. Moreover, the conventional missing at random assumption-a natural benchmark for monotone missingness-does not model realistic beliefs about the non-monotone missingness processes (Robins and Gill, 1997). This has provided the impetus for alternative non-monotone missing not at random mechanisms. The "no self-censoring" model is such a mechanism and assumes the probability an outcome variable is missing is independent of its value when conditioning on all other possibly missing outcome variables and their missingness indicators. As an alternative to "weighting" methods that become computationally demanding with increasing number of outcome variables, we propose a multiple imputation approach under no self-censoring. We focus on the case of binary outcomes and present results of simulation and asymptotic studies to investigate the performance of the proposed imputation approach. We describe a related approach to sensitivity analysis to departure from no self-censoring. We discuss the relationship between missing at random and no self-censoring and prove that one is not a special case of the other. Finally, we discuss extensions to non-binary data settings. The proposed methods are illustrated with application to a substance use disorder clinical trial.
更多查看译文
关键词
Missing at random,missing data,sensitivity analysis,fully conditional specification
AI 理解论文
溯源树
样例
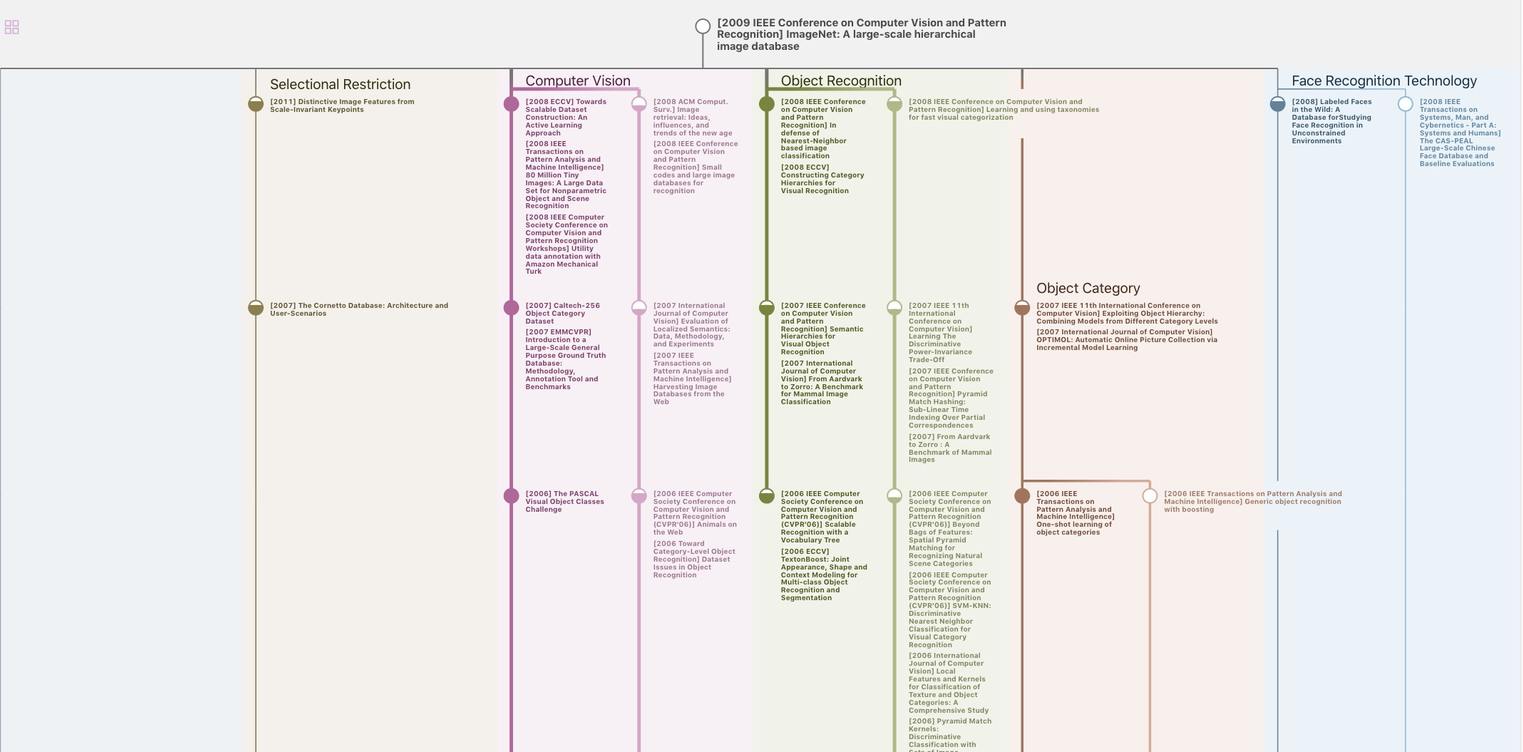
生成溯源树,研究论文发展脉络
Chat Paper
正在生成论文摘要