Retrieved Sequence Augmentation for Protein Representation Learning
bioRxiv (Cold Spring Harbor Laboratory)(2023)
摘要
Abstract The advancement of protein representation learning has been significantly influenced by the remarkable progress in language models. Accordingly, protein language models perform inference from individual sequences, thereby limiting their capacity to incorporate evolutionary knowledge present in sequence variations. Existing solutions, which rely on Multiple Sequence Alignments (MSA), suffer from substantial computational overhead and suboptimal generalization performance for de novo proteins. In light of these problems, we introduce a novel paradigm called Retrieved Sequence Augmentation (RSA) that enhances protein representation learning without necessitating additional alignment or preprocessing. RSA associates query protein sequences with a collection of structurally or functionally similar sequences in the database and integrates them for subsequent predictions. We demonstrate that protein language models benefit from retrieval enhancement in both structural and property prediction tasks, achieving a 5% improvement over MSA Transformer on average while being 373 times faster. Furthermore, our model exhibits superior transferability to new protein domains and outperforms MSA Transformer in de novo protein prediction. This study fills a much-encountered gap in protein prediction and brings us a step closer to demystifying the domain knowledge needed to understand protein sequences. Code is available at https://github.com/HKUNLP/RSA .
更多查看译文
关键词
protein,learning,sequence
AI 理解论文
溯源树
样例
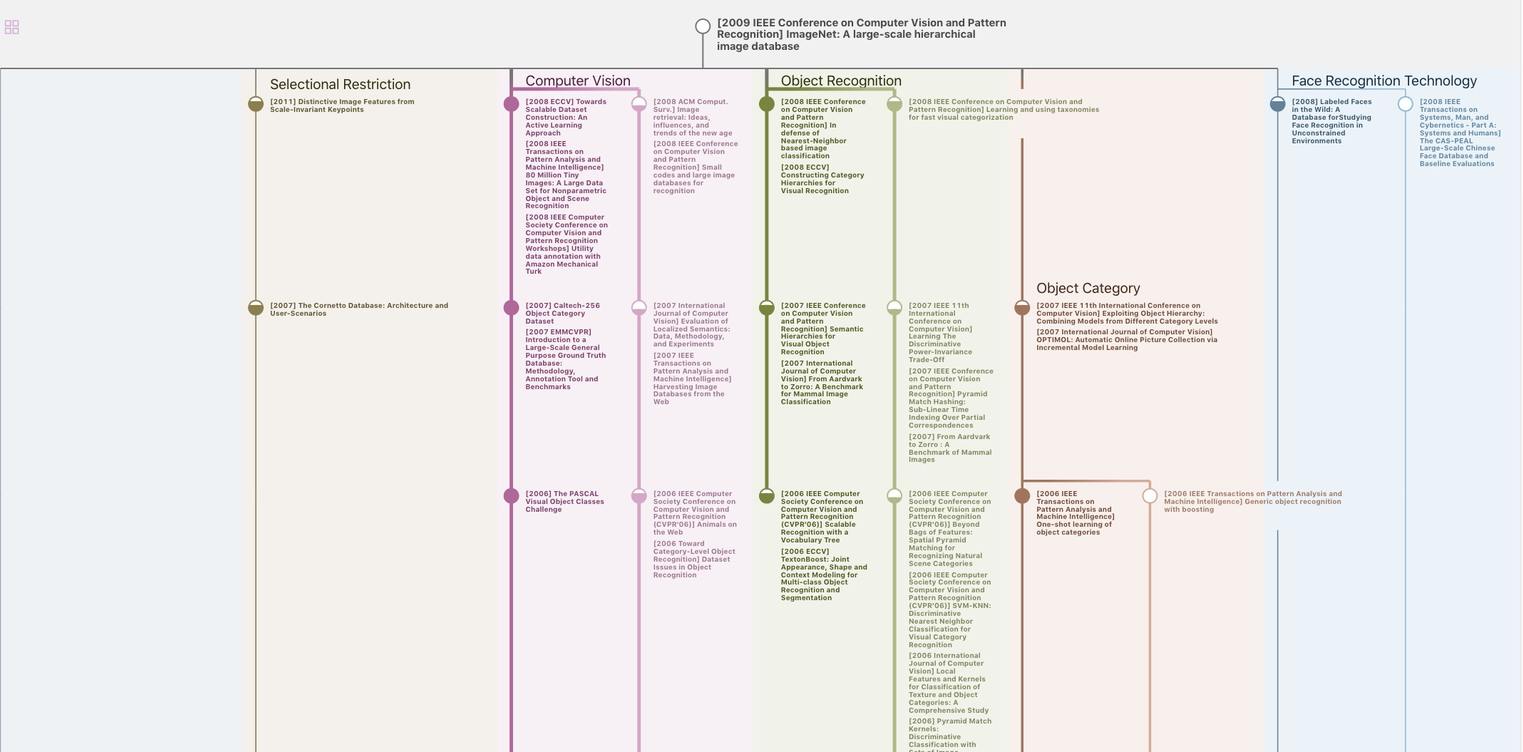
生成溯源树,研究论文发展脉络
Chat Paper
正在生成论文摘要