Laplace Power-Expected-Posterior Priors for Logistic Regression
Bayesian analysis(2023)
Abstract
Power-expected-posterior (PEP) methodology, which borrows ideas from the literature on power priors, expected-posterior priors and unit information priors, provides a systematic way to construct objective priors. The basic idea is to use imaginary training samples to update a (possibly improper) prior into a proper but minimally-informative one. In this work, we develop a novel definition of PEP priors for logistic regression models that relies on a Laplace expansion of the likelihood of the imaginary training sample. This approach has various advantages over previous proposals for non-informative priors in logistic regression, and can be easily extended to other generalized linear models. We study theoretical properties of the prior and provide a number of empirical studies that demonstrate superior performance both in terms of model selection and of parameter estimation, especially for heavy-tailed versions.
MoreTranslated text
AI Read Science
Must-Reading Tree
Example
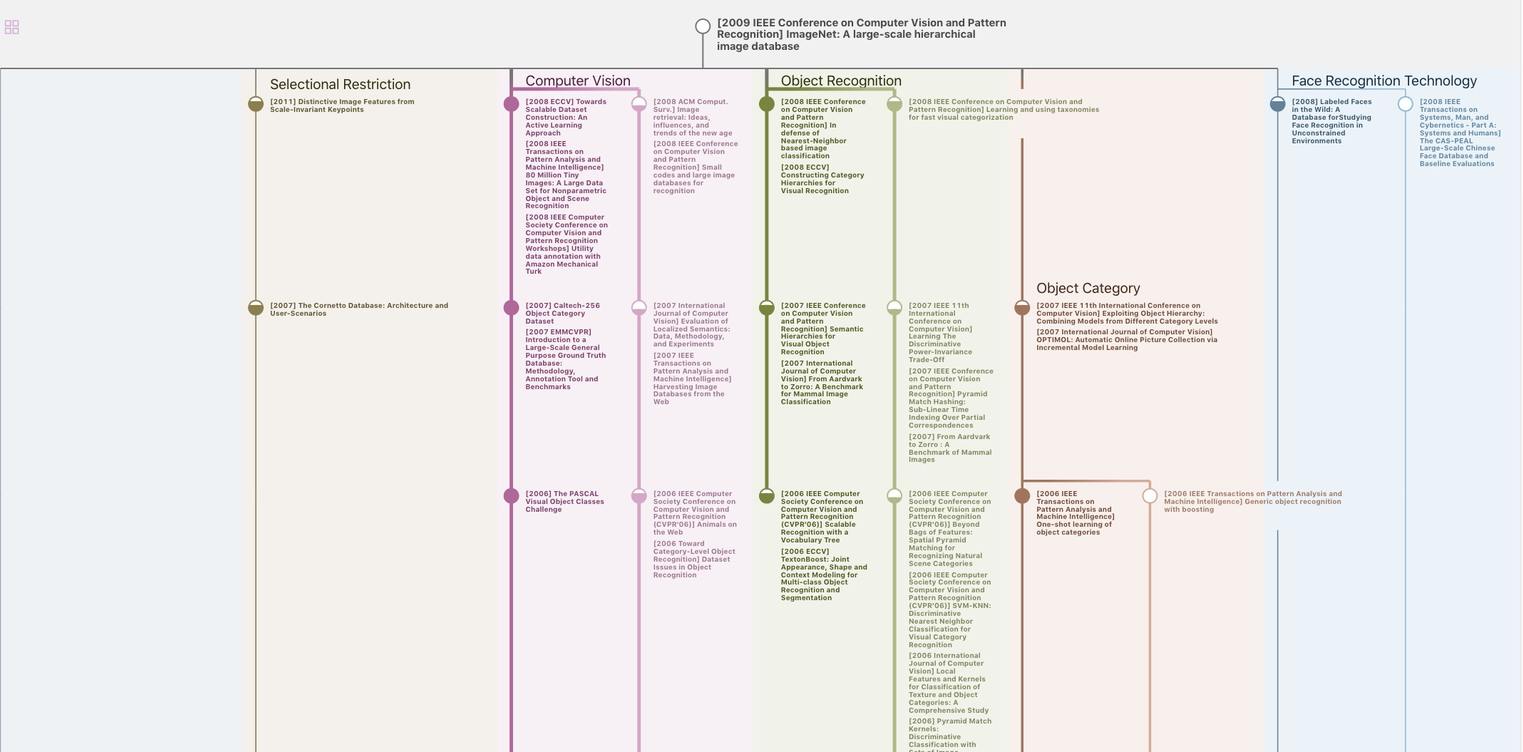
Generate MRT to find the research sequence of this paper
Chat Paper
Summary is being generated by the instructions you defined