Spot The Odd One Out: Regularized Complete Cycle Consistent Anomaly Detector GAN
arXiv (Cornell University)(2023)
摘要
This study presents an adversarial method for anomaly detection in real-world applications, leveraging the power of generative adversarial neural networks (GANs) through cycle consistency in reconstruction error. Previous methods suffer from the high variance between class-wise accuracy which leads to not being applicable for all types of anomalies. The proposed method named RCALAD tries to solve this problem by introducing a novel discriminator to the structure, which results in a more efficient training process. Additionally, RCALAD employs a supplementary distribution in the input space to steer reconstructions toward the normal data distribution, effectively separating anomalous samples from their reconstructions and facilitating more accurate anomaly detection. To further enhance the performance of the model, two novel anomaly scores are introduced. The proposed model has been thoroughly evaluated through extensive experiments on six various datasets, yielding results that demonstrate its superiority over existing state-of-the-art models. The code is readily available to the research community at https://github.com/zahraDehghanian97/RCALAD.
更多查看译文
关键词
detector gan,complete cycle consistent anomaly
AI 理解论文
溯源树
样例
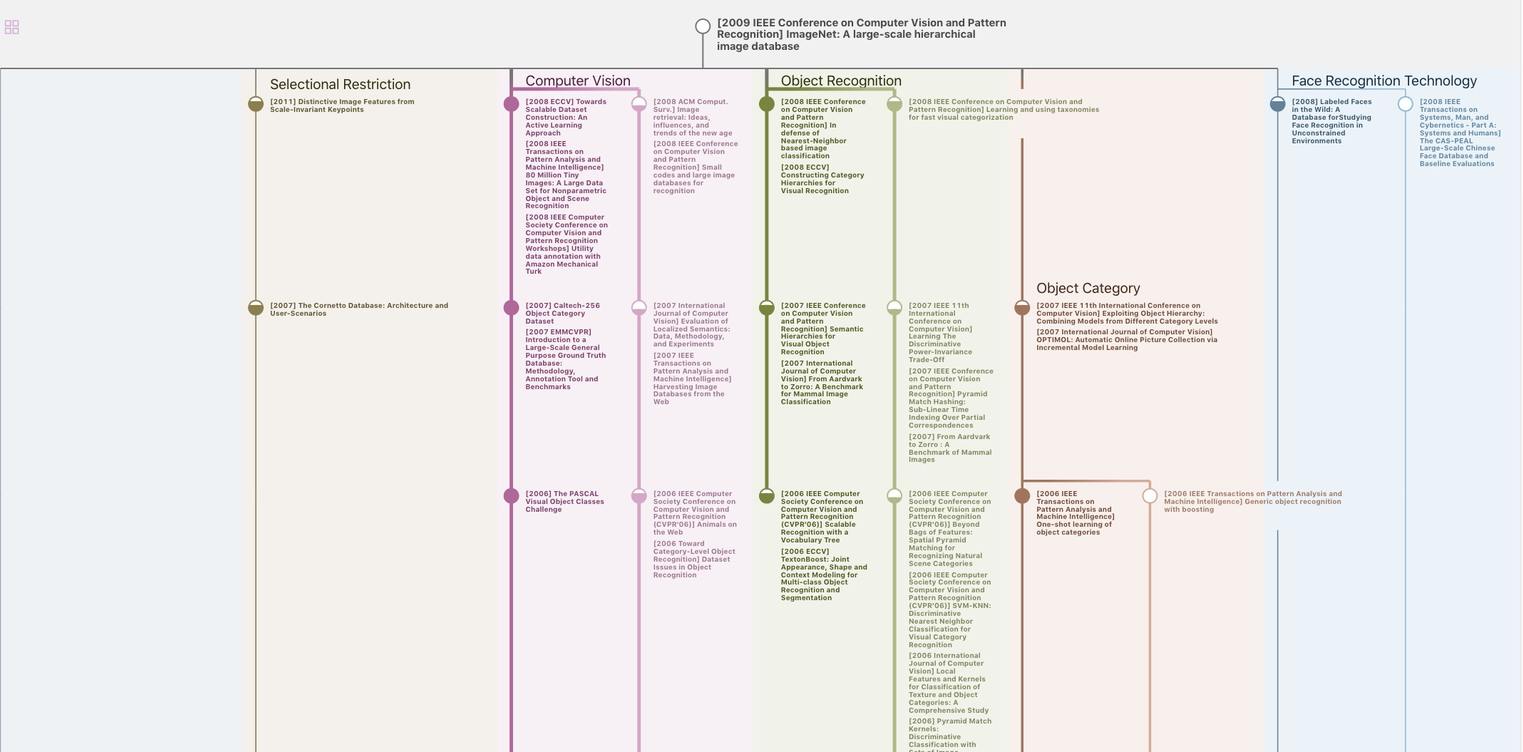
生成溯源树,研究论文发展脉络
Chat Paper
正在生成论文摘要