Deformable Kernel Expansion Model for Efficient Arbitrary-shaped Scene Text Detection
arXiv (Cornell University)(2023)
摘要
Scene text detection is a challenging computer vision task due to the high variation in text shapes and ratios. In this work, we propose a scene text detector named Deformable Kernel Expansion (DKE), which incorporates the merits of both segmentation and contour-based detectors. DKE employs a segmentation module to segment the shrunken text region as the text kernel, then expands the text kernel contour to obtain text boundary by regressing the vertex-wise offsets. Generating the text kernel by segmentation enables DKE to inherit the arbitrary-shaped text region modeling capability of segmentation-based detectors. Regressing the kernel contour with some sampled vertices enables DKE to avoid the complicated pixel-level post-processing and better learn contour deformation as the contour-based detectors. Moreover, we propose an Optimal Bipartite Graph Matching Loss (OBGML) that measures the matching error between the predicted contour and the ground truth, which efficiently minimizes the global contour matching distance. Extensive experiments on CTW1500, Total-Text, MSRA-TD500, and ICDAR2015 demonstrate that DKE achieves a good tradeoff between accuracy and efficiency in scene text detection.
更多查看译文
关键词
deformable kernel expansion model,text,detection,scene
AI 理解论文
溯源树
样例
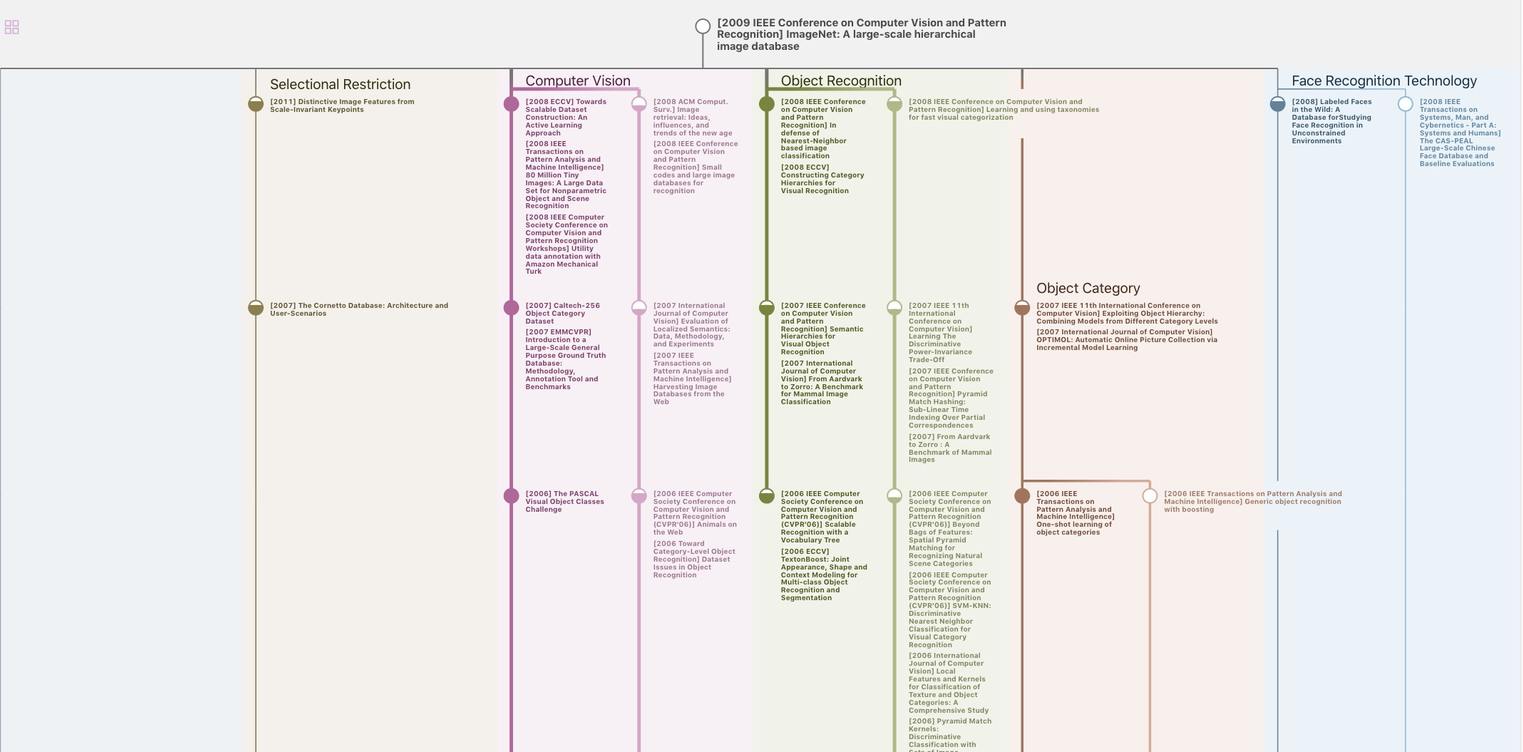
生成溯源树,研究论文发展脉络
Chat Paper
正在生成论文摘要