Data-driven Reduced-Order Modelling for Blood Flow Simulations with Geometry-Informed Snapshots
JOURNAL OF COMPUTATIONAL PHYSICS(2024)
摘要
Parametric reduced-order modelling often serves as a surrogate method for hemodynamics simulations to improve the computational efficiency in many-query scenarios or to perform real-time simulations. However, the snapshots of the method require to be collected from the same discretisation, which is a straightforward process for physical parameters, but becomes challenging for geometrical problems, especially for those domains featuring unparameterised and unique shapes, e.g. patient-specific geometries. In this work, a data-driven surrogate model is proposed for the efficient prediction of blood flow simulations on similar but distinct domains. The proposed surrogate model leverages group surface registration to parameterise those shapes and formulates corresponding hemodynamics information into geometry-informed snapshots by the diffeomorphisms constructed between a reference domain and original domains. A non-intrusive reduced-order model for geometrical parameters is subsequently constructed using proper orthogonal decomposition, and a radial basis function interpolator is trained for predicting the reduced coefficients of the reduced-order model based on compressed geometrical parameters of the shape. Two examples of blood flowing through a stenosis and a bifurcation are presented and analysed. The proposed surrogate model demonstrates its accuracy and efficiency in hemodynamics prediction and shows its potential application toward real-time simulation or uncertainty quantification for complex patient-specific scenarios.
更多查看译文
关键词
Model Reduction,Data-Driven Modeling,Numerical Simulation,Probabilistic Design Optimization
AI 理解论文
溯源树
样例
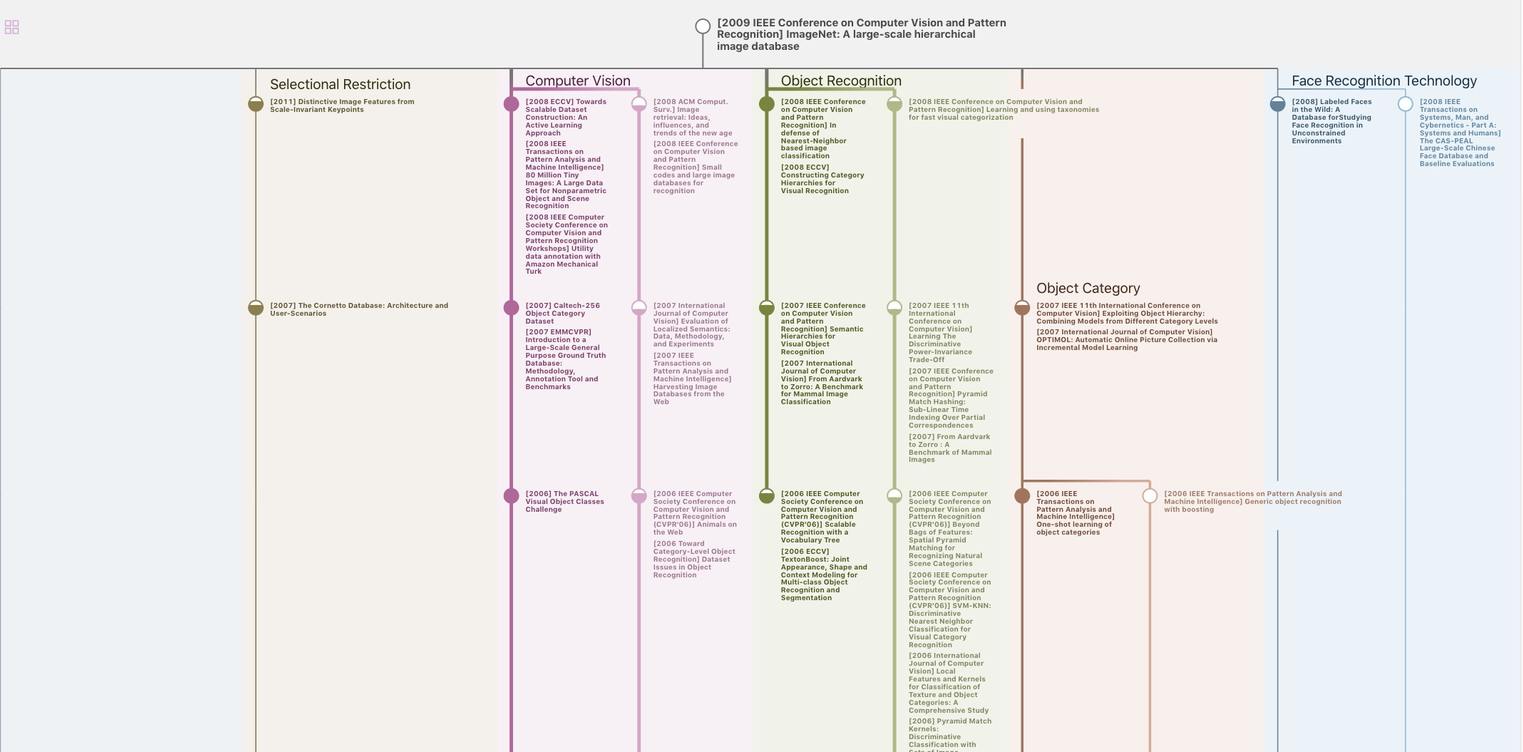
生成溯源树,研究论文发展脉络
Chat Paper
正在生成论文摘要