Original Research Article an Arti Fi Cial Neural Network Emulator of the Rangeland Hydrology and Erosion Model
INTERNATIONAL SOIL AND WATER CONSERVATION RESEARCH(2024)
摘要
Machine learning (ML) is becoming an ever more important tool in hydrologic modeling. Previous studies have shown the higher prediction accuracy of those ML models over traditional process -based ones. However, there is another advantage of ML which is its lower computational demand. This is important for the applications such as hydraulic soil erosion estimation over a large area and at a finer spatial scale. Using traditional models like Rangeland Hydrology and Erosion Model (RHEM) requires too much computation time and resources. In this study, we designed an Arti ficial Neural Network that is able to recreate the RHEM outputs (annual average runoff, soil loss, and sediment yield and not the daily storm event -based values) with high accuracy (Nash -Sutcliffe Ef ficiency z 1.0) and a very low computational time (13 billion times faster on average using a GPU). We ran the RHEM for more than a million synthetic scenarios and train the Emulator with them. We also, fine-tuned the trained Emulator with the RHEM runs of the real -world scenarios (more than 32,000) so the Emulator remains comprehensive while it works speci fically accurately for the real -world cases. We also showed that the sensitivity of the Emulator to the input variables is similar to the RHEM and it can effectively capture the changes in the RHEM outputs when an input variable varies. Finally, the dynamic prediction behavior of the Emulator is statistically similar to the RHEM. (c) 2023 International Research and Training Center on Erosion and Sedimentation, China Water and Power Press, and China Institute of Water Resources and Hydropower Research. Publishing services by Elsevier B.V. on behalf of KeAi Communications Co. Ltd. This is an open access article under the CC BYNC -ND license (http://creativecommons.org/licenses/by-nc-nd/4.0/).
更多查看译文
关键词
RHEM,Sediment yield,Soil loss,Runoff,Deep learning
AI 理解论文
溯源树
样例
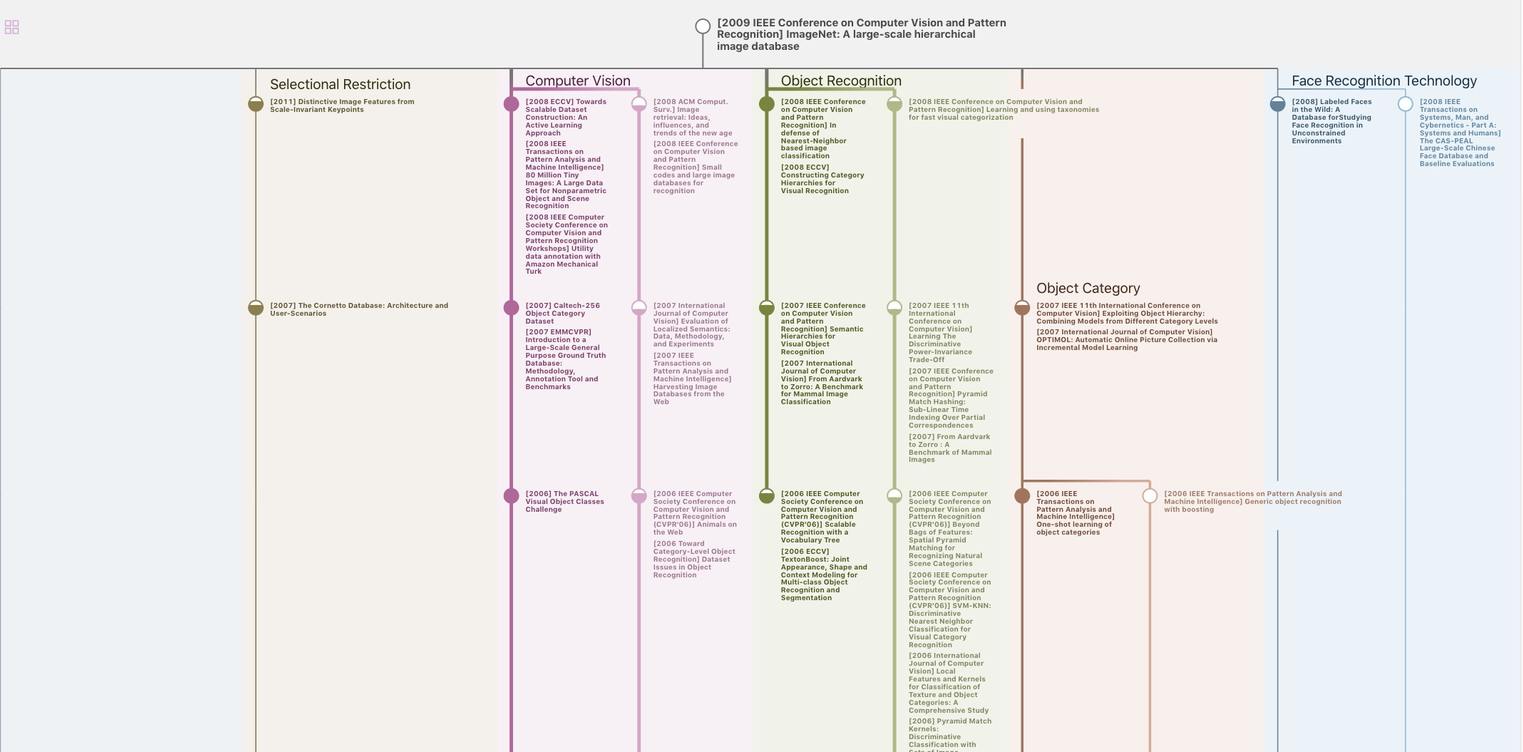
生成溯源树,研究论文发展脉络
Chat Paper
正在生成论文摘要