A Joint Entity-Relation Detection and Generalization Method Based on Syntax and Semantics for Chinese Intangible Cultural Heritage Texts
ACM JOURNAL ON COMPUTING AND CULTURAL HERITAGE(2024)
Abstract
Annotation of a natural language corpus not only facilitates researchers in extracting knowledge from it but also helps achieve deeper mining of the corpus. However, an annotated corpus in the humanities knowledge domain is lacking. In addition, the semantic annotation of humanities texts is difficult, because it requires a high domain background for researchers and even requires the participation of domain experts. Based on this, this study proposes a method for detecting entities and relations in a domain that lacks an annotated corpus, as well as provides a referenceable idea for constructing conceptual models based on textual instances. Based on syntactic and semantic features, this study proposes SPO triple recognition rules from the perspective of giving priority to predicates and generalization rules from the perspective of a triple’s content and the meaning of its predicate. The recognition rules are used to extract text-descriptive SPO triples centered on predicates. After clustering and adjusting triples, the generalization rules proposed in this study are used to obtain coarse-grained entities and relations, and then form a conceptual model. This study recognizes SPO triples with high precision and summarization from descriptive texts, generalizes them, and then forms a domain conceptual model. Our proposed method provides a research idea for entity-relation detection in a domain with a missing annotated corpus, and the formed domain conceptual model provides a reference for building a domain Linked Data Graph. The feasibility of the method is verified through practice on texts related to the four traditional Chinese festivals.
MoreTranslated text
Key words
Digital humanity,intangible cultural heritage,relation extraction,Linked Data
AI Read Science
Must-Reading Tree
Example
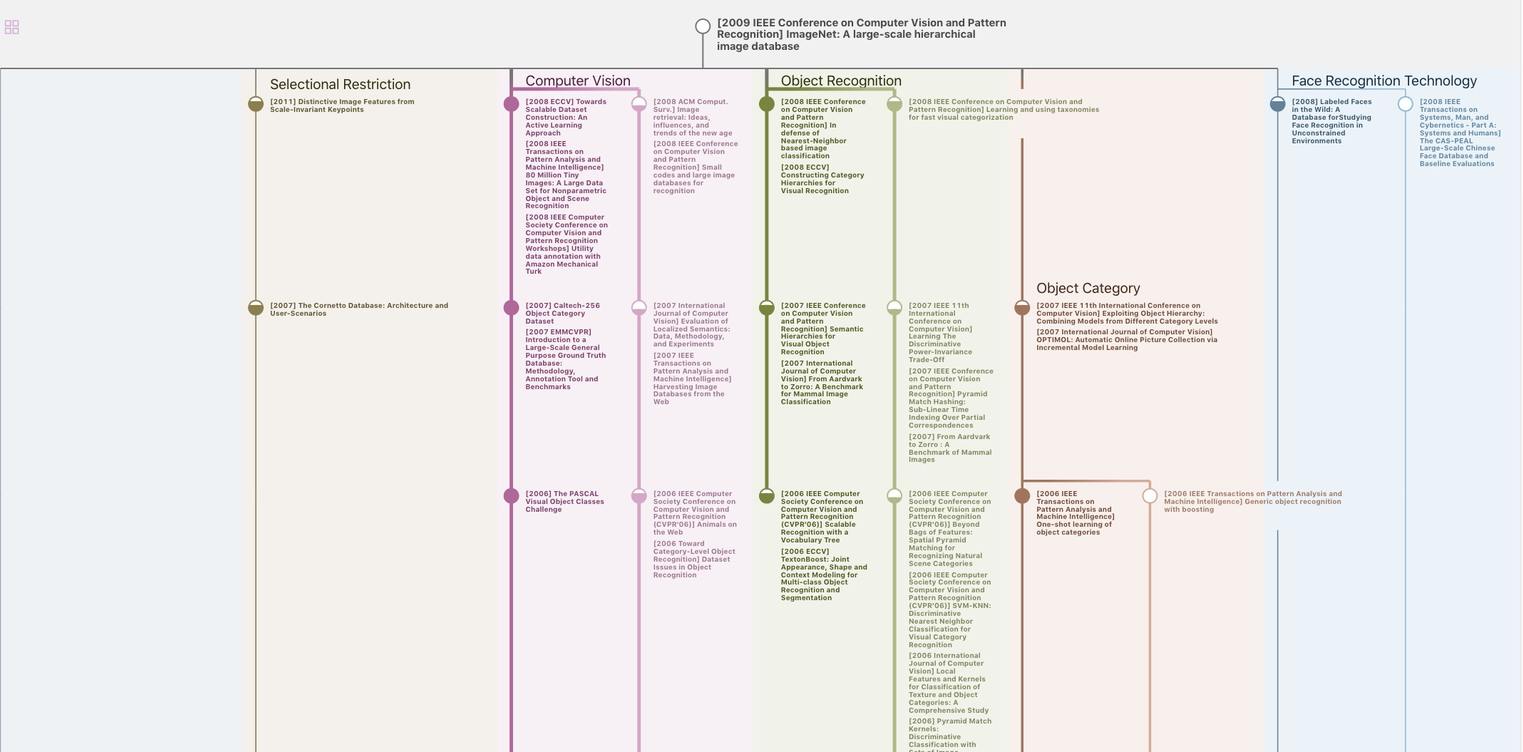
Generate MRT to find the research sequence of this paper
Chat Paper
Summary is being generated by the instructions you defined