PREVALENCE OF POST-COVID-19 CONDITION IN PATIENTS WITH CHRONIC KIDNEY DISEASE, ON DIALYSIS AND LIVING WITH A KIDNEY TRANSPLANT
Nephrology Dialysis Transplantation(2023)SCI 2区
UMC | Erasmus MC | Univ Med Ctr Groningen | Leiden Univ Med Ctr LUMC | UMC Utrecht | Amsterdam UMC | Dutch Kidney Patients Assoc NVN | Radboud Univ Nijmegen Med Ctr | Canisius Wilhelmina Hosp | Maasstad Hosp
Abstract
Background and Aims The prevalence of post-COVID-19 condition (PCC) is estimated to be 13% in healthy individuals. We analyzed the prevalence and disease burden of PCC in patients with chronic kidney disease (CKD) G4/5, dialysis patients and kidney transplant recipients (KTR). Method Patients participated in the RECOVAC study, in which SARS-CoV-2 antibodies were measured in CKD G4/5, dialysis patients and KTR after the second and third COVID-19 vaccination in the Netherlands. A questionnaire was sent to 4868 participants one year after initial vaccination asking for the presence of long-lasting symptoms after diagnosis in COVID-19 positive patients, or since the start of the pandemic in COVID-19 negative patients. PCC was defined according to the WHO clinical case definition. Blood samples at one month after the second and third vaccination were analysed with anti-RBD IgG ELISA. COVID-19 diagnosis was assessed by questionnaire or positive anti-nucleocapsid IgG antibodies. Logistic regression analysis was used to compare the presence of one or more long-lasting symptoms between COVID-19 positive and negative patients. In COVID-19 positive patients, we likewise identified predictors of PCC by backward selection and estimated the association between log-transformed antibody levels and PCC. Results 2747 patients were included, of which 222 patients with CKD G4/5, 390 dialysis patients and 2135 KTR. PCC was present in 25%, 16%, and 21% of CKD G4/5 patients, dialysis patients and KTR with high or very high symptom burden in 57%, 61% and 71%, respectively. In COVID-19 negative patients, long-lasting symptoms were present in 15%, 13% and 18%, respectively. COVID-19 positive patients (n = 1004) were at higher odds of having one or more long-lasting symptoms compared with COVID-19 negative patients (n = 1743) (OR: 1.33 [1.09–1.61], p = .005). Predictors of PCC were chronic lung disease (adjusted OR 2.04 [1.18#x2013;3.50], p = .01) and hospital/ICU admission (adjusted OR 5.03 [3.22-7.86], p < .001). Log anti-RBD IgG antibody level was negatively associated with PCC (adjusted OR: 0.79 [0.66#x2013;0.94], p = .008). Conclusion Patients with CKD G4/5, dialysis patients and KTR are at risk for PCC with a high symptom burden, especially if antibody levels after COVID-19 vaccination are low.
MoreTranslated text
求助PDF
上传PDF
View via Publisher
AI Read Science
AI Summary
AI Summary is the key point extracted automatically understanding the full text of the paper, including the background, methods, results, conclusions, icons and other key content, so that you can get the outline of the paper at a glance.
Example
Background
Key content
Introduction
Methods
Results
Related work
Fund
Key content
- Pretraining has recently greatly promoted the development of natural language processing (NLP)
- We show that M6 outperforms the baselines in multimodal downstream tasks, and the large M6 with 10 parameters can reach a better performance
- We propose a method called M6 that is able to process information of multiple modalities and perform both single-modal and cross-modal understanding and generation
- The model is scaled to large model with 10 billion parameters with sophisticated deployment, and the 10 -parameter M6-large is the largest pretrained model in Chinese
- Experimental results show that our proposed M6 outperforms the baseline in a number of downstream tasks concerning both single modality and multiple modalities We will continue the pretraining of extremely large models by increasing data to explore the limit of its performance
Upload PDF to Generate Summary
Must-Reading Tree
Example
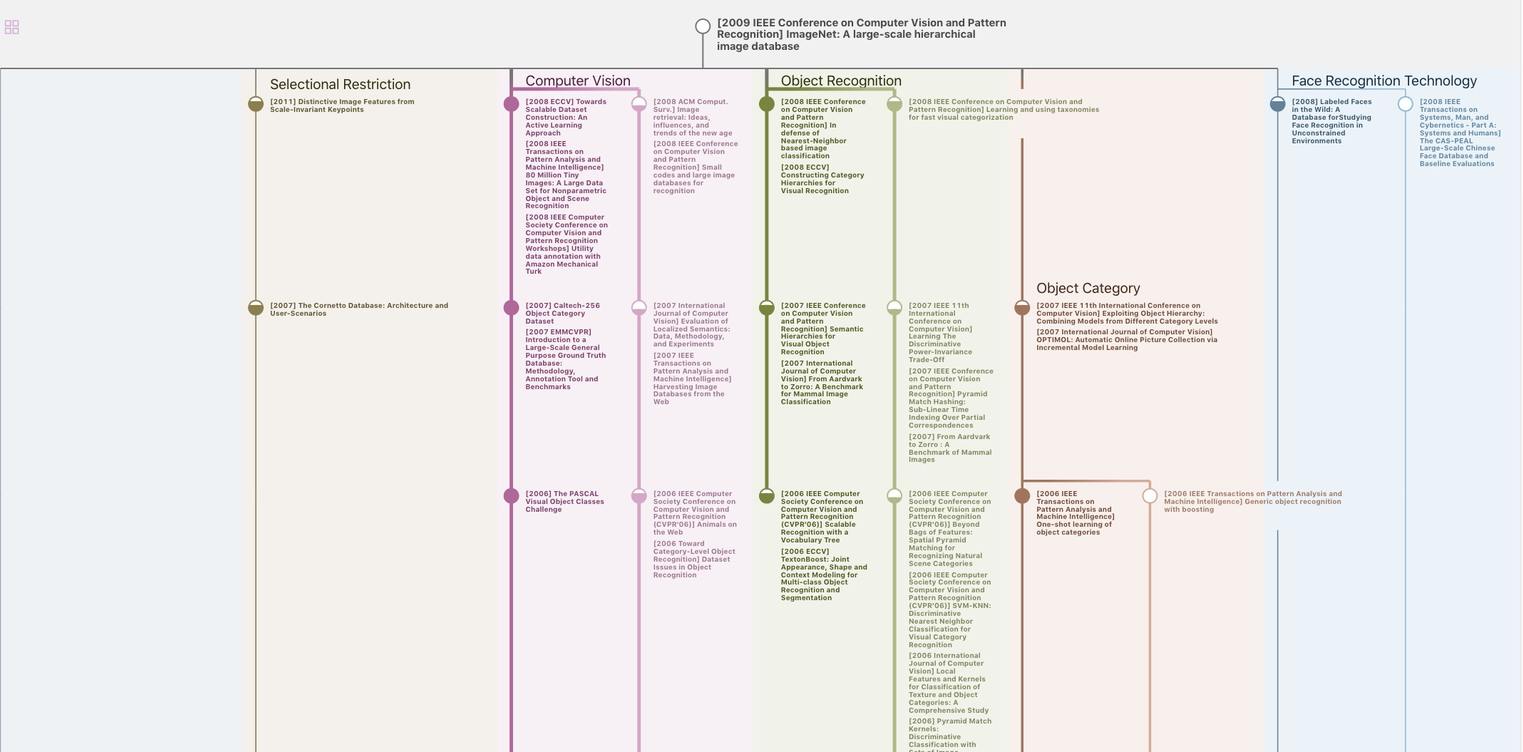
Generate MRT to find the research sequence of this paper
Data Disclaimer
The page data are from open Internet sources, cooperative publishers and automatic analysis results through AI technology. We do not make any commitments and guarantees for the validity, accuracy, correctness, reliability, completeness and timeliness of the page data. If you have any questions, please contact us by email: report@aminer.cn
Chat Paper
GPU is busy, summary generation fails
Rerequest