A Digital Twin of the Infant Microbiome to Predict Neurodevelopmental Deficits
Research Square (Research Square)(2023)
摘要
Abstract Growing evidence that dysbiosis in the infant microbiome can lead to impaired cognitive development suggests a promising target for interventions. However, the precise mechanisms of microbial influence on neurodevelopment, to inform effective clinical interventions, remain unclear. The complexity of the gut ecosystem, with thousands of cross-talking microbial colonizers, together with sparsely observed abundance profiles, has limited progress. Here we introduce a computational framework to learn an approximate “digital twin” of the maturing infant microbiome, that once learned, can reliably forecast detailed ecosystem trajectories unfolding over weeks from few initial observations. This generative model (Q-net), inferred at the level of taxonomic classes of microbes automatically from standard 16S rRNA profiles, is used to uncover actionable patterns driving developmental fate in early life. We infer the Q-net from longitudinal fecal samples of preterm infants (n = 88, with 398 fecal samples and 32,942 abundance estimates for observed 91 microbial classes) from distinct cohorts recruited in two sites (2-5 samples per subject collected weekly), and is validated to forecast temporal dynamics of key bacterial classes in outof-sample subjects (Rsquared=0.64) over weeks from <= 2 initial observations. Additionally, by contrasting the digital twins inferred separately for typical and suboptimally developing sub-cohorts, we suggest a general approach to estimate the risk (A-delta) of future anomalous deviation of microbiome maturation trajectory for individual patients. We show that the A-delta risk predicts eventual developmental deficit in individuals within the first 2-4 weeks of life, demonstrated by carrying out out-of-sample classification of preterm infants into categories of typical vs sub-optimal head circumference growth (a proxy for cognitive development) with AUC ~76% (positive predictive value 95% +/- 1.8% at 98% specificity) at 30 weeks postmenstrual age (PMA). Finally, we investigate the role of specific microbial classes and their abundances over time, delivery mode and infant diet in atypical microbiome trajectories. Stepping beyond correlative association and diversity estimates, our digital twins reveal deep n-way interactions of bacterial classes from sparse observational data, and may open the door to patient-specific precision interventions.
更多查看译文
关键词
infant microbiome,neurodevelopmental deficits,digital twin
AI 理解论文
溯源树
样例
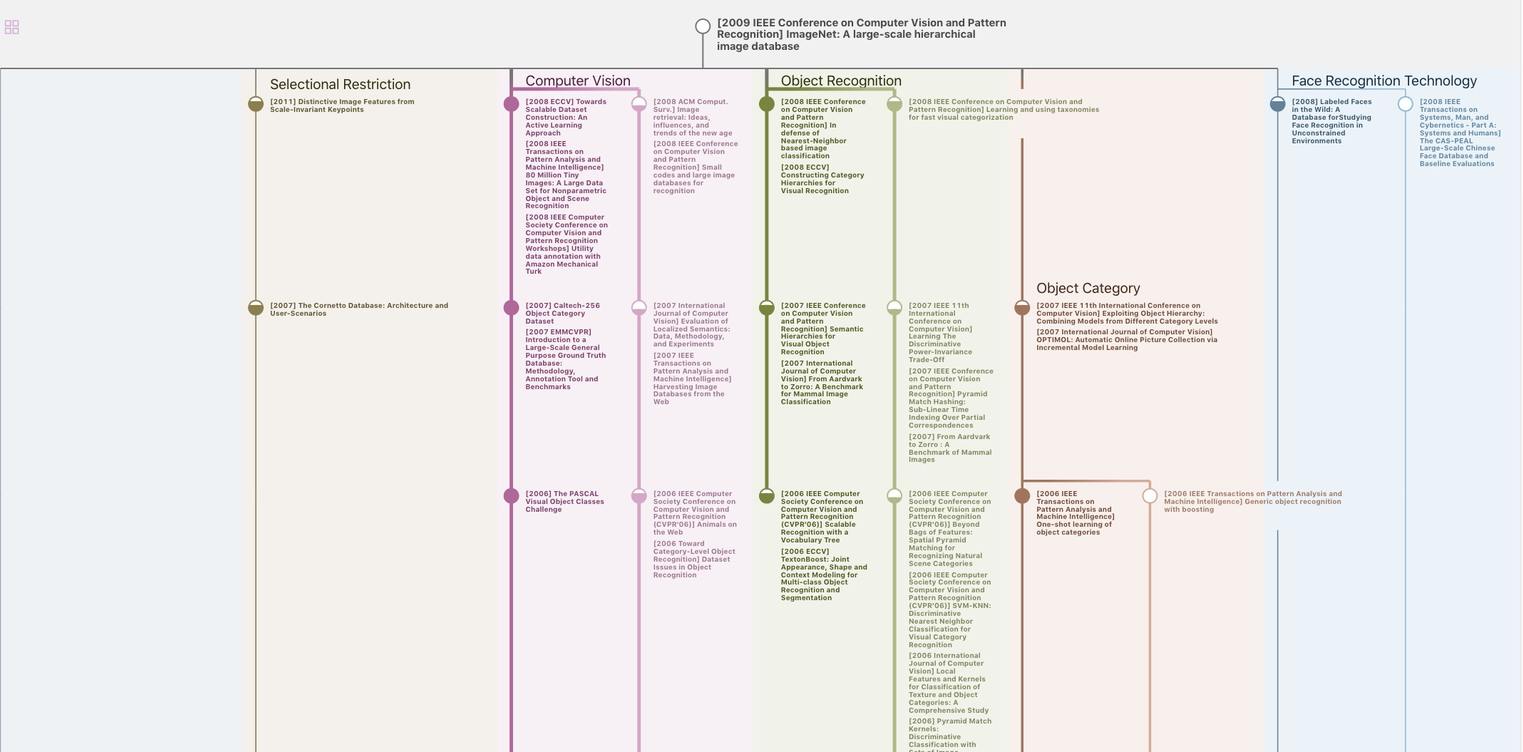
生成溯源树,研究论文发展脉络
Chat Paper
正在生成论文摘要