Short-Term Fine-Grained Regional MUF Prediction for HF Communication Based on Time-Series Decomposition
IEEE TRANSACTIONS ON ANTENNAS AND PROPAGATION(2023)
摘要
High-frequency (HF) communication plays a vital role in military and commercial fields because of its characteristics of transhorizon propagation. Accurate prediction of the maximum usable frequency (MUF) is critical to improve the efficiency of HF ionospheric propagation. Deep learning has demonstrated remarkable performance in time-series prediction in recent years. However, the MUF is a nonstationary time series with complex properties of multiple time scales. Therefore, we must fully explore the features of the MUF sequence to provide reliable support for selecting deep-learning prediction models. In this article, we first decompose the real-world MUF-measured data from vertical sounding stations to obtain different components. After analyzing the phenomena of each component at different positions, we propose the capability requirements of the MUF prediction model and verify the capability requirements by comparing the existing most advanced deep-learning prediction models. Then, we calculate the complexity of the MUF sequence based on multiscale entropy. Through the entropy series at different time scales, the key to improving the model's prediction performance is the fitting ability of the nonlinear change of the long-term trend component in the short-period scale. Furthermore, we propose a quantitative prediction scheme of MUF based on multiscale entropy similarity tolerance. Based on the Fano inequality, we calculate and compare the upper and lower bounds of the predictability on the quantization sequence and the original data and verify the effectiveness of the proposed scheme. Through experimental results, as the latitude decreases, the models based on time-series decomposition have better prediction performance over the state-of-the-art schemes. Compared to nontime-series decomposition deep-learning models, the root mean square error (RMSE) of the predicted MUF values for four cities, ranging from low to high latitude, decreased by an average of 0.11, 0.20, 0.02, and 0.03 MHz, respectively.
更多查看译文
关键词
Predictive models,Deep learning,Time series analysis,Entropy,Data models,Market research,Loss measurement,high-frequency communication,ionospheric vertical sounding,maximum usable frequency (MUF),predictability,time-series decomposition
AI 理解论文
溯源树
样例
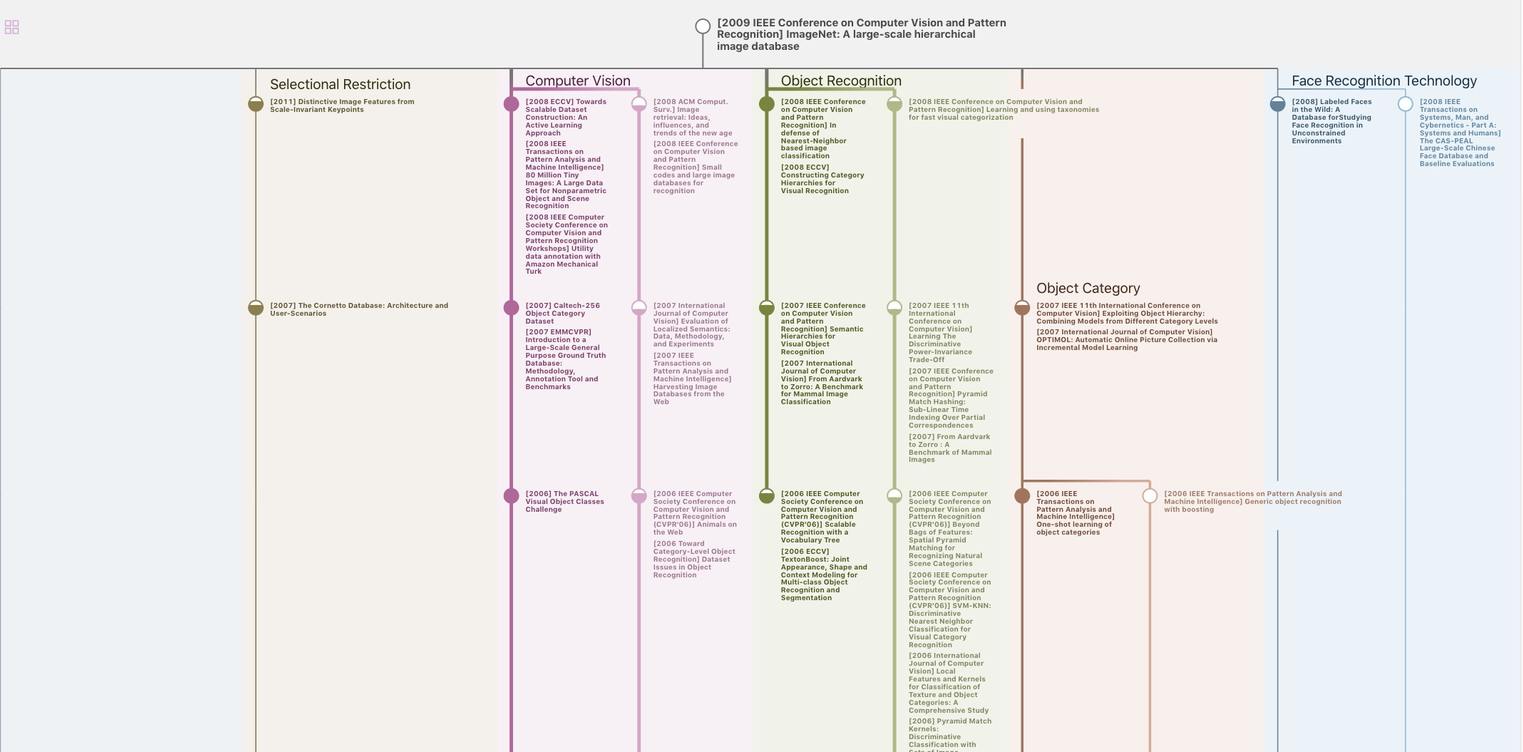
生成溯源树,研究论文发展脉络
Chat Paper
正在生成论文摘要