ConvNets Match Vision Transformers at Scale
arXiv (Cornell University)(2023)
摘要
Many researchers believe that ConvNets perform well on small or moderately sized datasets, but are not competitive with Vision Transformers when given access to datasets on the web-scale. We challenge this belief by evaluating a performant ConvNet architecture pre-trained on JFT-4B, a large labelled dataset of images often used for training foundation models. We consider pre-training compute budgets between 0.4k and 110k TPU-v4 core compute hours, and train a series of networks of increasing depth and width from the NFNet model family. We observe a log-log scaling law between held out loss and compute budget. After fine-tuning on ImageNet, NFNets match the reported performance of Vision Transformers with comparable compute budgets. Our strongest fine-tuned model achieves a Top-1 accuracy of 90.4%.
更多查看译文
关键词
Computer Vision
AI 理解论文
溯源树
样例
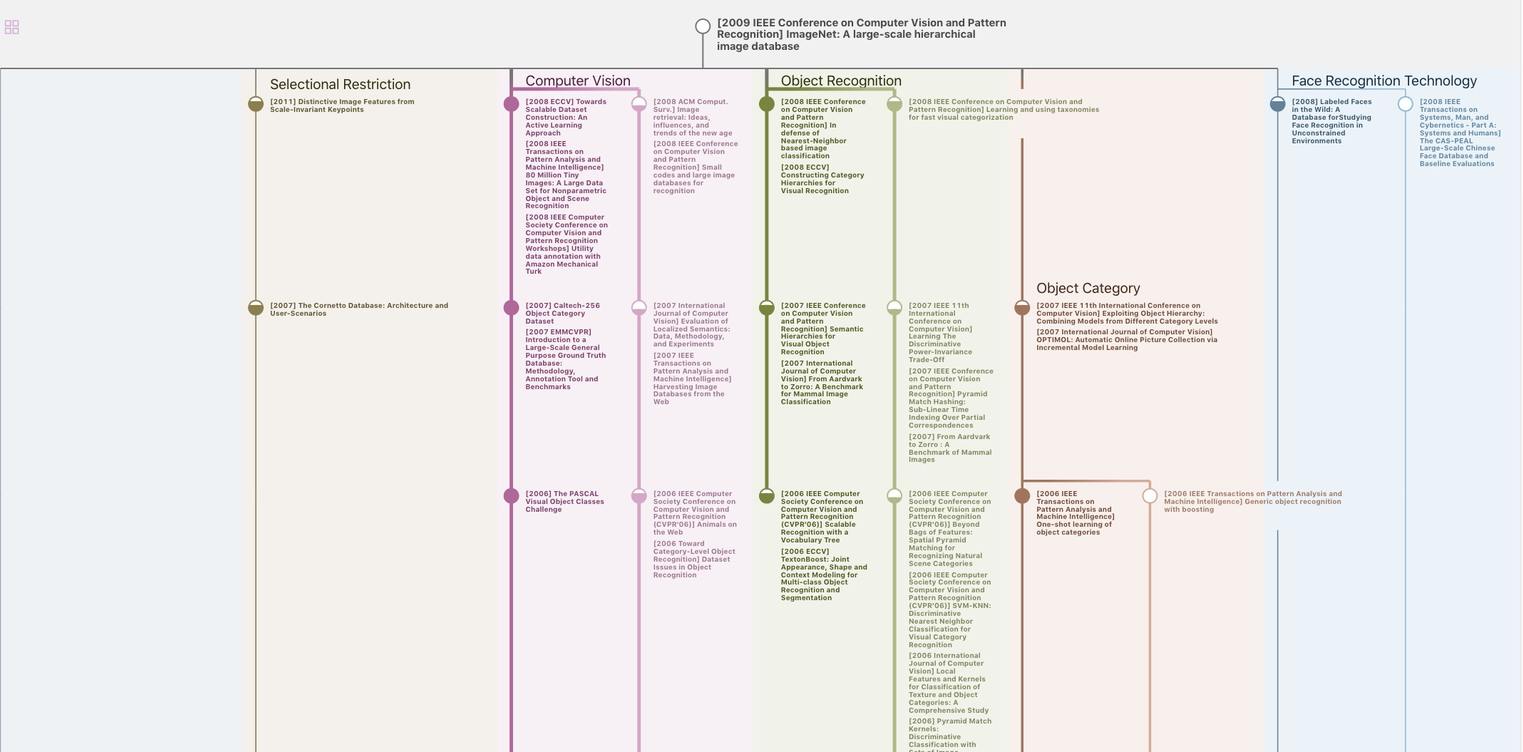
生成溯源树,研究论文发展脉络
Chat Paper
正在生成论文摘要