Involving Prediction of Dynamic Modulus in Asphalt Mix Design with Machine Learning and Mechanical-Empirical Analysis
Construction & building materials(2023)
Abstract
Dynamic modulus (|E*|) plays a dominant role in comprehensively capturing the mechanical behavior of asphalt mixture. Many researchers tried to consider |E*| as a performance indicator for a mix design stage, but the cost of time and labor for experimentally measuring the |E*| is high. Moreover, establishing harmonized |E*| criterion is unrealistic. To involve |E*| in an asphalt mix design, this paper improved the Superpave volumetric mixture design via controlling the performance (rutting) of mixtures, which were estimated by incorporating machine learning (ML) predictive models of |E*| into the mechanical-empirical approach. The data from the concatenation of the original |E*| database and volumetric properties data extracted from the NCHRP 9-19 report were involved. The different ML models including Support Vector Regression (SVR), kernel ridge regression (KRR), artificial Neural Networks (ANN), Gaussian process regression (GPR), gradient boosting (GB), and eXtreme gradient boosting (XGBoost) were optimized, trained and tested, and their performances were compared to empirical Witczak's predictive equations. To illustrate the improved asphalt mix design, the case study was presented. Candidate asphalt mixtures were prepared by mixing the selected gradation and different asphalt content and subject to a series of |E*| predictions using the best ML model, which were then used to calculate the permanent deformation of these mixtures with elastic layered analysis and transfer functions in MATLAB. The results show that among all the ML models, XGBoost to predict the |E*| at actual scale has the largest prediction accuracy (R2 = 0.9867), and its prediction accuracy is significantly higher than Witczak's equations. Feature importance analysis suggested that no matter which scale of |E*| is predicted, the main important factors are test conditions and asphalt binder properties. The relationship between asphalt contents and estimated rutting by the proposed procedure agrees with the engineering experience, which suggests the feasibility of the proposed asphalt mix design procedure.
MoreTranslated text
Key words
Asphalt mixture,Dynamic modulus,Machine learning,MEPDG,Rutting
AI Read Science
Must-Reading Tree
Example
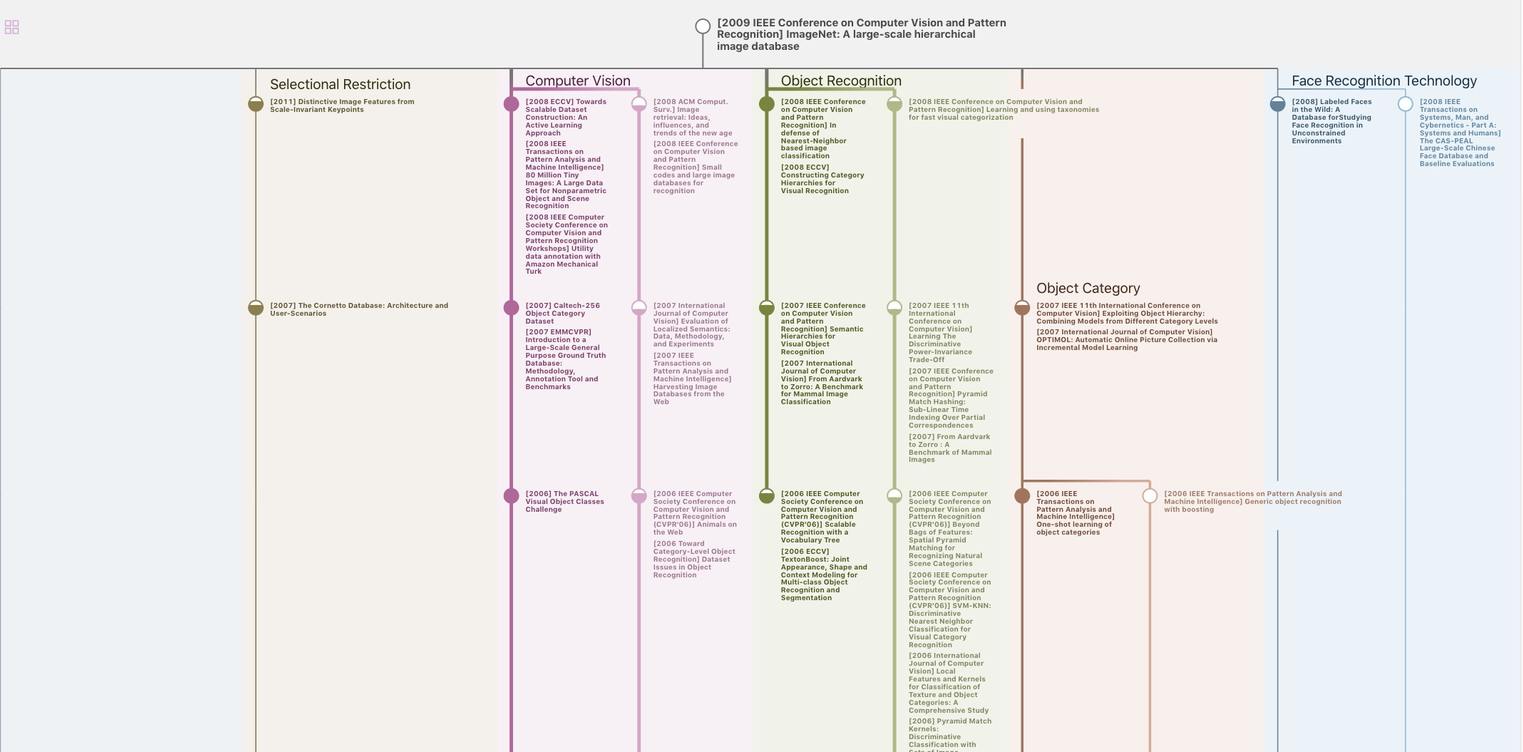
Generate MRT to find the research sequence of this paper
Chat Paper
Summary is being generated by the instructions you defined