A latent process approach to change-point detection of mixed-type observations
QUALITY ENGINEERING(2024)
摘要
Mixed-type observations, such as continuous measurements, discrete counts, and binary outcomes, are commonly present in many applications. The change-point detection with mixed-type observations is challenging since it is difficult to quantify the hidden association among mixed-type observations. In this work, we propose a latent process method to model the mixed observations in a joint manner, and effectively detect the changes. Bayesian parameter estimation and inference are developed for the proposed method by combining the discrete particle filter (DPF) and sequential Monte Carlo (SMC) algorithms. Such an algorithm can efficiently update the high dimensional proposal distribution and can exploit the discrete and continuous natures of the latent processes simultaneously. The performance of the proposed method is illustrated by several numerical examples and a case study of civil-unrest data.
更多查看译文
关键词
Bayesian inference,discrete particle filter,latent process approach,mixed-type observations,sequential Monte Carlo
AI 理解论文
溯源树
样例
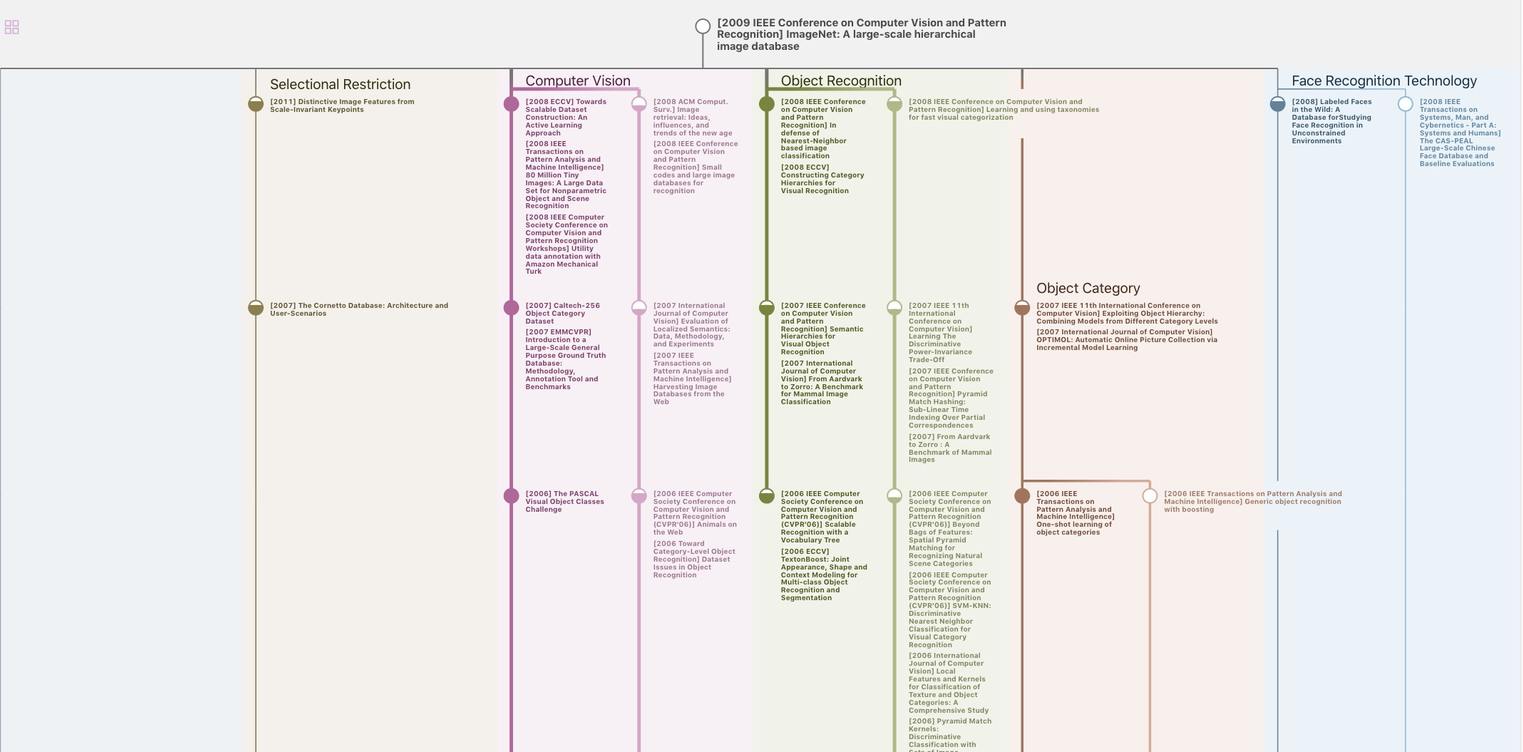
生成溯源树,研究论文发展脉络
Chat Paper
正在生成论文摘要