An Evaluation of Modalities for Action Recognition
openalex(2023)
Abstract
Inductive biases of neural networks play a crucial role in their ability to learn robust representations for visual tasks. Human action recognition is a particularly challenging domain where inductive biases in the form of input modalities have profound impacts on the algorithms’ success. However, past research has not yet identified the optimal set of modalities to use for action recognition. In this thesis, a number of modalities are evaluated and compared using various metrics, including overall accuracy, per-class performance, and amount of contextual information encoded. This is done by training a deep 3D convolutional neural network on each input modality, while controlling variables like video length and dataset, which reveal characteristics of each modality. It is shown that certain modalities lend themselves better to specific network architectures, tasks, and data sets, which has relevance to real-world action recognition systems and future research in the field of multi-modal action recognition.
MoreTranslated text
Key words
Action Recognition,Human Activity Analysis,Backpropagation Learning,Image Recognition,Feedforward Neural Networks
AI Read Science
Must-Reading Tree
Example
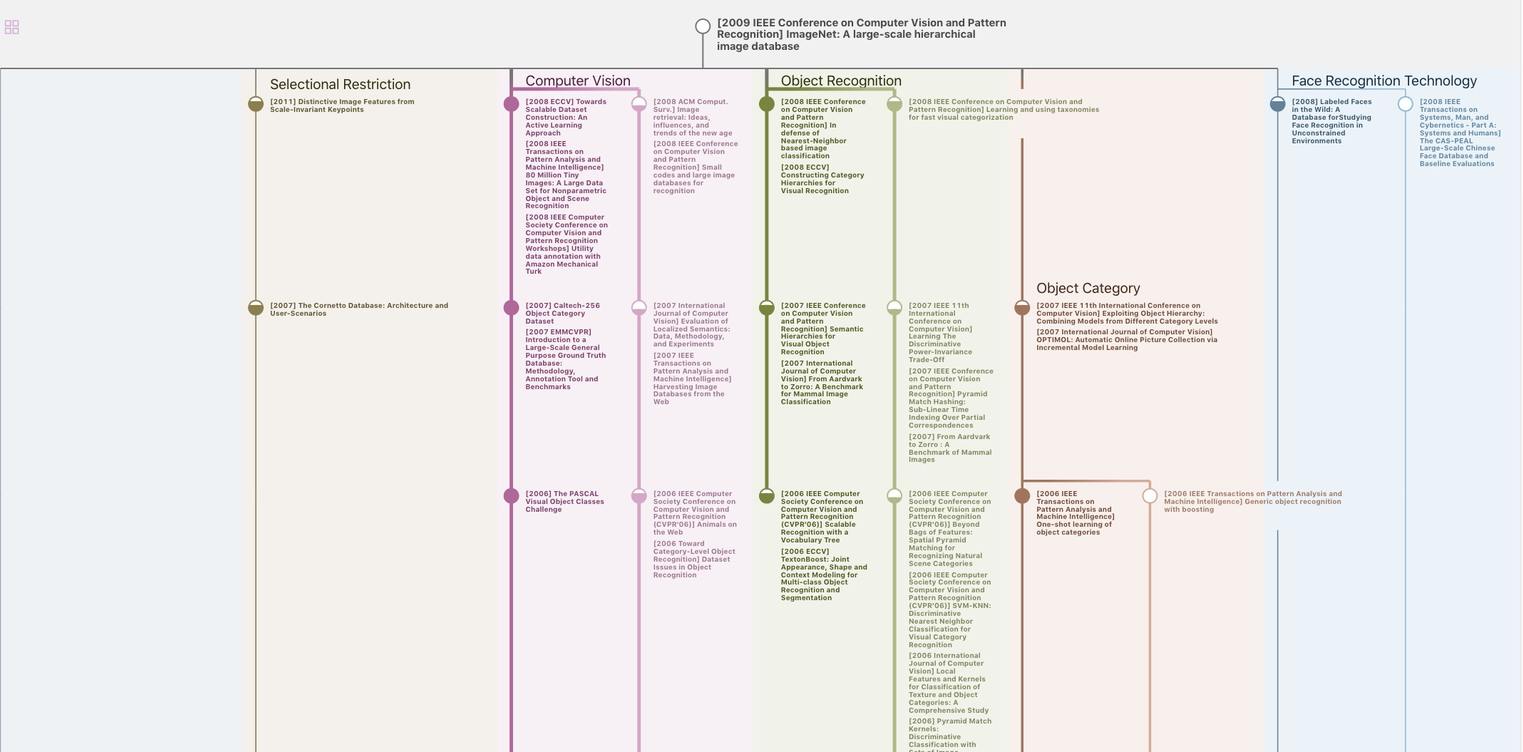
Generate MRT to find the research sequence of this paper
Chat Paper
Summary is being generated by the instructions you defined