Effects of Attack Interference on Radio Signal Classification
Journal of physics Conference series(2023)
摘要
Signal recognition and classification are urgent issues in the field of radio monitoring. Although there have been many academic publications on the automatic modulation classification of radio signals, these studies have focused on how to use the new algorithms to increase signal recognition accuracy, and there is a lack of consideration of the effect of interference on classification accuracy. Based on publicly available datasets, RF datasets for Machine Learning, this paper uses the deep learning algorithm to generate an attack interference dataset and studies the impact of attack interference and noise on the classification accuracy of radio signals. The experimental results are as follows. Firstly, the presence of attack interference generally reduces the accuracy of radio signal classification. Secondly, the power of attack interference has a direct relationship with classification accuracy. Thirdly, under the condition of attack interference, the classification performance trends of the three open-source public datasets are consistent. This further illustrates how optimizing the modulation classification performance in the presence of interference is the key to applying the algorithm to practice.
更多查看译文
AI 理解论文
溯源树
样例
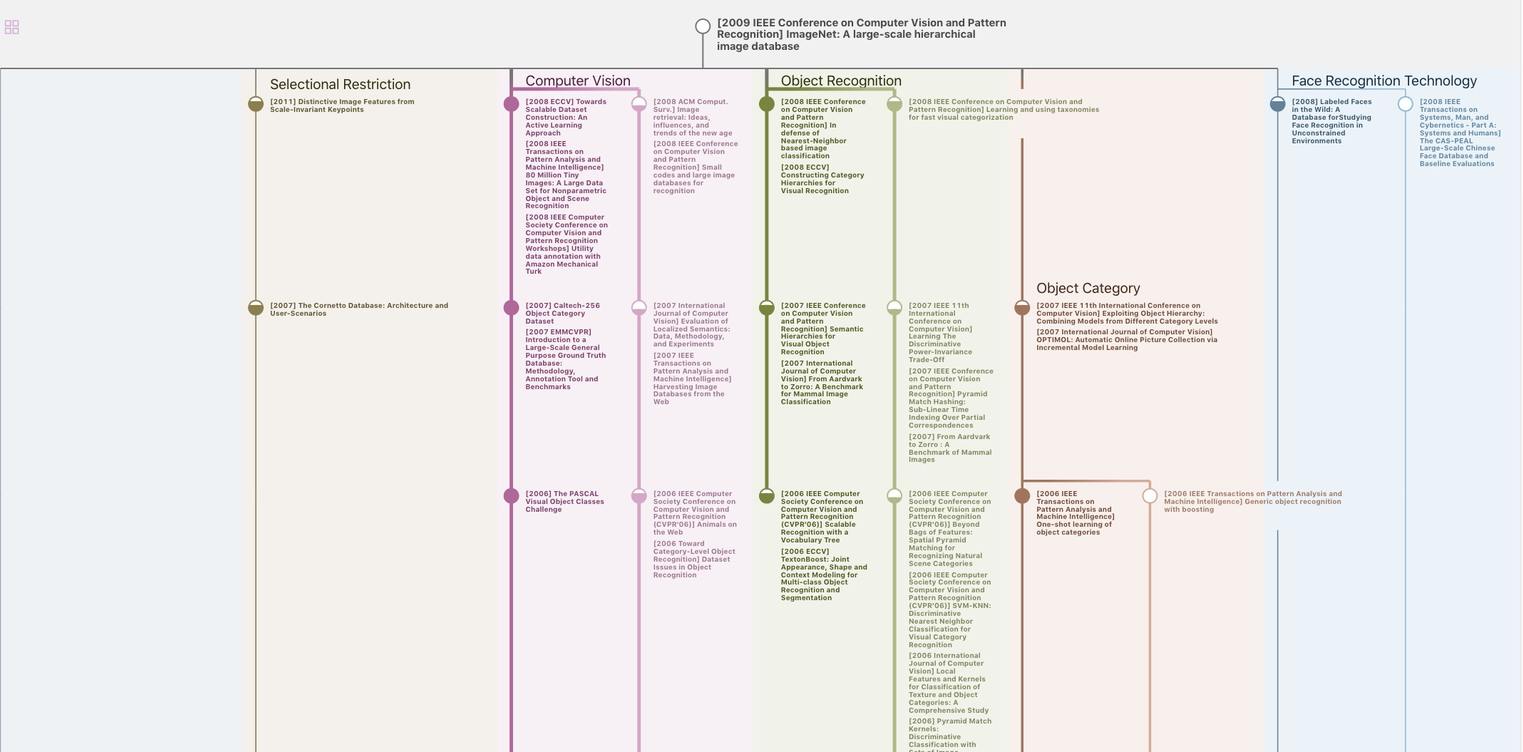
生成溯源树,研究论文发展脉络
Chat Paper
正在生成论文摘要