3D Point Cloud Outliers and Noise Reduction Using Neural Networks
Communications in computer and information science(2023)
摘要
3D point clouds find widespread use in various areas of computing research, such as 3D reconstruction, point cloud segmentation, navigation, and assisted driving, to name a few examples. A point cloud is a collection of coordinates that represent the shape or surface of an object or scene. One way to generate these point clouds is by using RGB-D cameras. However, one major issue when using point clouds is the presence of noise and outliers caused by various factors, such as environmental conditions, object reflectivity, and sensor limitations. Classification and segmentation tasks can become complex when point clouds contain noise and outliers. This paper proposes a method to reduce outliers and noise in 3D point clouds. Our proposal builds on a deep learning architecture called PointCleanNet, which we modified by adding extra convolutional layers to extract feature maps that help classify point cloud outliers. We demonstrate the effectiveness of our proposed method in improving outlier classification and noise reduction in non-dense point clouds. We achieved this by including a low-density point cloud dataset in the training stage, which helped our method classify outliers more efficiently than PointCleanNet and Luo, S, et al.
更多查看译文
关键词
3d point cloud outliers,noise reduction,neural networks
AI 理解论文
溯源树
样例
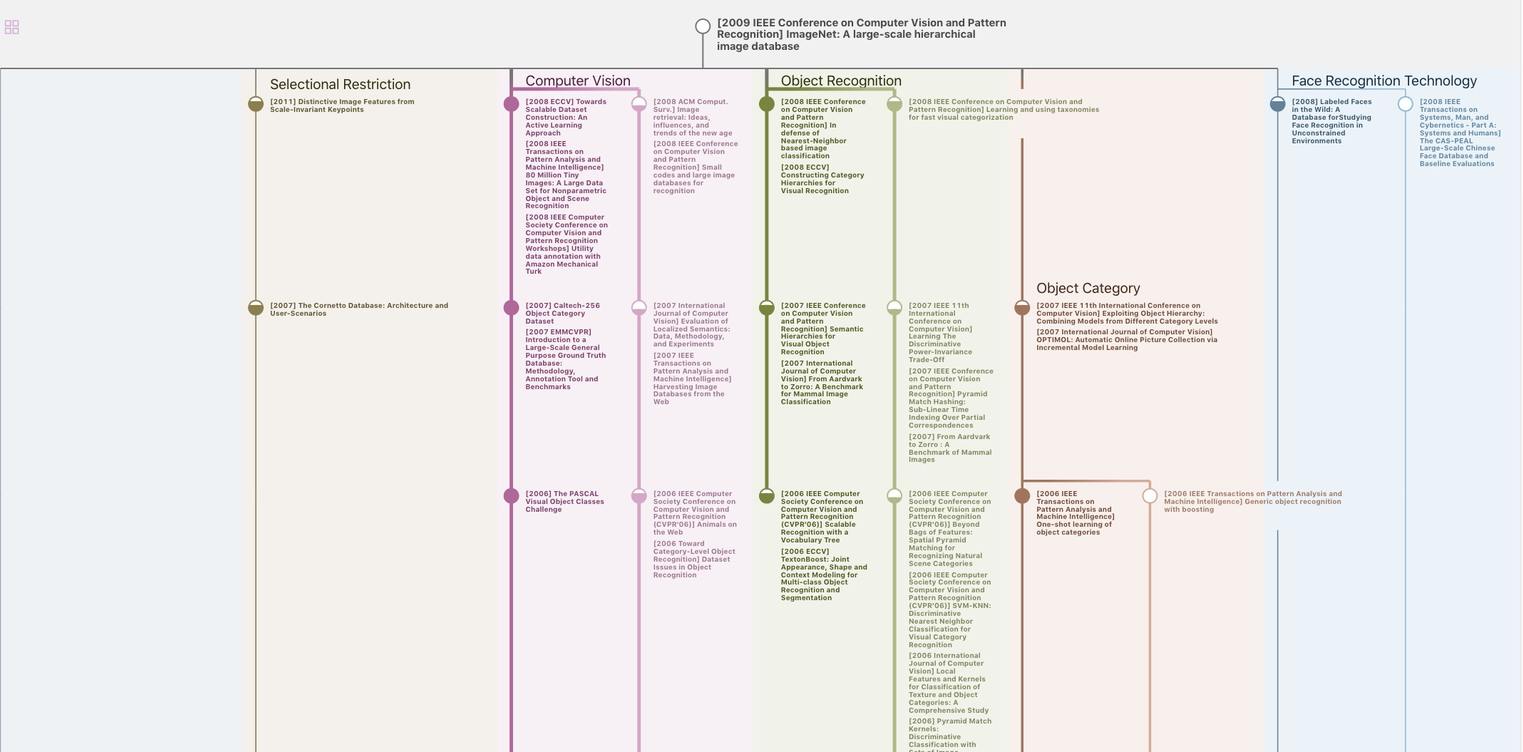
生成溯源树,研究论文发展脉络
Chat Paper
正在生成论文摘要