LEVERAGING FUNCTIONAL GENOMIC ANNOTATIONS AND GENOME COVERAGE TO IMPROVE POLYGENIC PREDICTION OF COMPLEX TRAITS WITHIN AND BETWEEN ANCESTRIES
European neuropsychopharmacology(2023)
摘要
Polygenic risk score (PRS) can facilitate precision medicine by identifying individuals of high genetic risk of disease, but its medical applications are currently impeded by the limited prediction accuracy in most complex diseases. The prediction accuracy is even lower when PRS is applied across ancestries, potentially leading to enlarged health disparity between populations. Hence, powerful methods that generate more accurate PRS are critical to propelling the roll-out of precision medicine. In this study, we developed a new Bayesian method, called SBayesRC, to improve PRS by integrating summary statistics from genome-wide association studies (GWAS) with functional genomic annotations, which provide orthogonal information about the causal variants underlying complex traits. Our method is scalable to whole-genome variant analysis and refines signals from functional annotations by allowing them to affect both causal variant probability and causal effect distribution. We applied SBayesRC to 50 complex traits including diseases using 7.4 million imputed common SNPs with 96 functional genomic annotations (comprising both discrete and continuous annotations), considered both within European ancestry and trans-ancestry prediction using datasets from UK Biobank, Lifelines Cohort, GIANT consortium, Biobank Japan (East Asian ancestry or EAS), and PAGE (mixed African ancestry or AFR), and made comparisons with the best methods in the literature. Additionally, we investigated factors that affect prediction accuracy when exploiting annotation data, and considered connections between the genetic architecture of functional categories and their contributions to prediction accuracy. Compared to the baseline method SBayesR which does not use annotations, SBayesRC improved prediction accuracy by 14% in European ancestry and by up to 34% in trans-ancestry prediction, across different traits. Our method outperformed the best method for within European ancestry prediction using annotations, MegaPRS, by 5%, and the best method for cross-ancestry prediction using annotations, PolyPred-S, by 15% averaged across ancestries (20% in EAS), and that without annotations but jointly models GWAS summary statistics from multiple populations, PRS-CSx, by 14% and 41% when using additional set of summary data from the target population of EAS and AFR ancestry, respectively. Investigation of factors affecting prediction accuracy identified a significant interaction between SNP density and annotation information that use of 7M SNPs can amplify the benefits of incorporating annotations into prediction as compared to 1M HapMap3 SNPs. This significant interaction is consistently observed across methods, suggesting that this is capturing a biological signal independent of the prediction methods. Functional partitioning analysis highlights a major contribution of evolutionary constrained regions to prediction accuracy, with a per-SNP contribution comparable to that in the coding sequence, and the largest per-SNP contribution from non-synonymous SNPs. In conclusion, the method proposed in this study is a powerful tool to improve polygenic prediction in complex traits and diseases. Given the significant interaction between SNP density and annotation information, we anticipate further improved prediction accuracy in the future when the method is applied to whole-genome sequence data with high-quality trait-relevant annotations.
更多查看译文
AI 理解论文
溯源树
样例
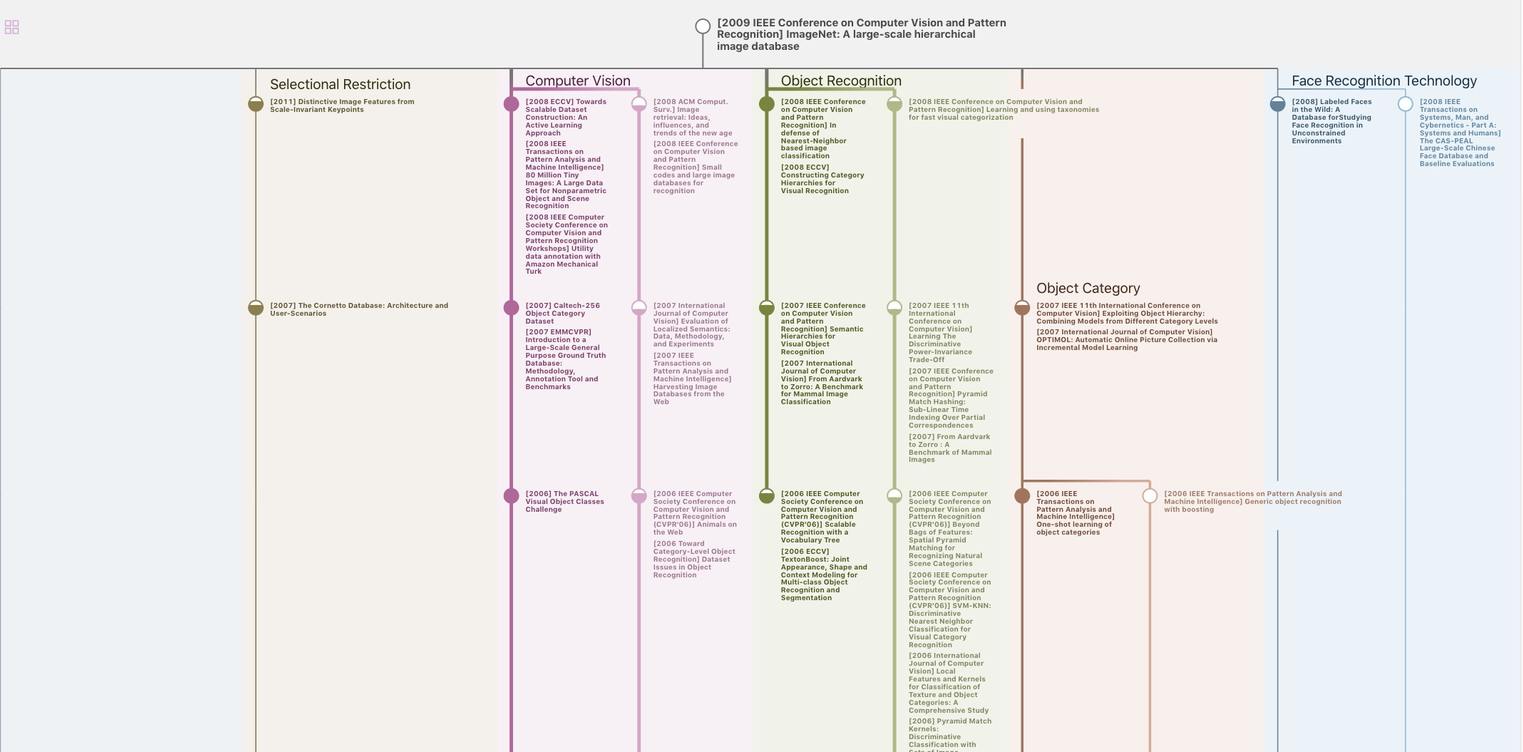
生成溯源树,研究论文发展脉络
Chat Paper
正在生成论文摘要