Experimental Investigation of Machine Learning Based Soft-Failure Management Using the Optical Spectrum
arXiv (Cornell University)(2023)
摘要
The demand for high-speed data is exponentially growing. To conquer this, optical networks have undergone significant changes, getting more complex and versatile. The increasing complexity necessitates that the fault management be more adaptive to enhance network assurance. In this paper, we experimentally compare the performance of soft-failure management of different machine learning algorithms. We further introduce a machine-learning-based soft-failure management framework. It utilizes a variational autoencoder-based generative adversarial network (VAE-GAN) running on optical spectral data obtained by optical spectrum analyzers. The framework is able to reliably run on a fraction of available training data as well as identify unknown failure types. The investigations show that the VAE-GAN outperforms the other machine learning algorithms when up to 10% of the total training data are available in identification tasks. Furthermore, the advanced training mechanism for the GAN shows a high F1-score for unknown spectrum identification. The failure localization comparison shows the advantage of a low complexity neural network in combination with a VAE over established machine learning algorithms.
更多查看译文
关键词
Optical fiber networks,Generative adversarial networks,Optical receivers,Location awareness,Adaptive optics,Training,Machine learning algorithms
AI 理解论文
溯源树
样例
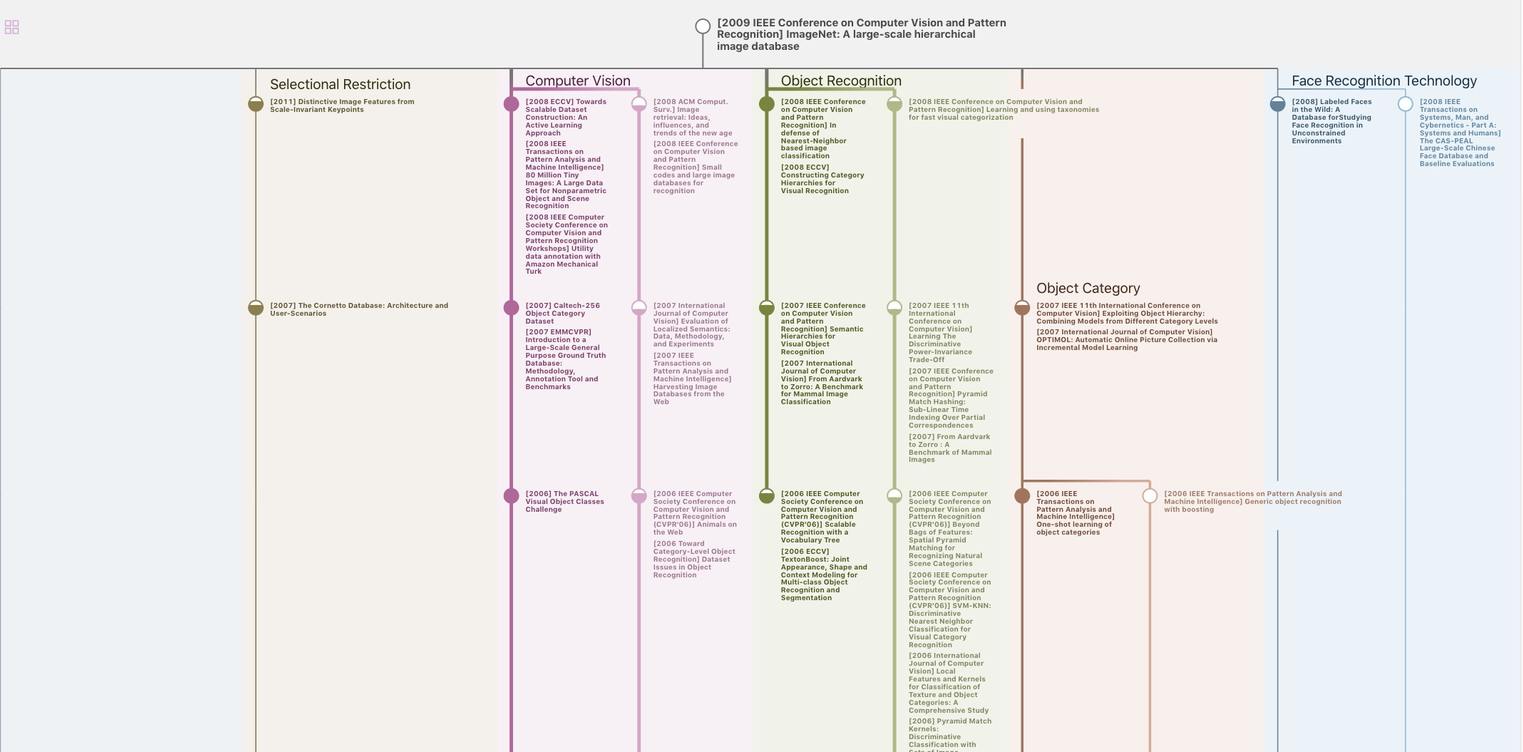
生成溯源树,研究论文发展脉络
Chat Paper
正在生成论文摘要