A semi-automatic tool to quantify training load in endurance athletes
European Journal of Preventive Cardiology(2023)
关键词
training load,endurance athletes,semi-automatic
AI 理解论文
溯源树
样例
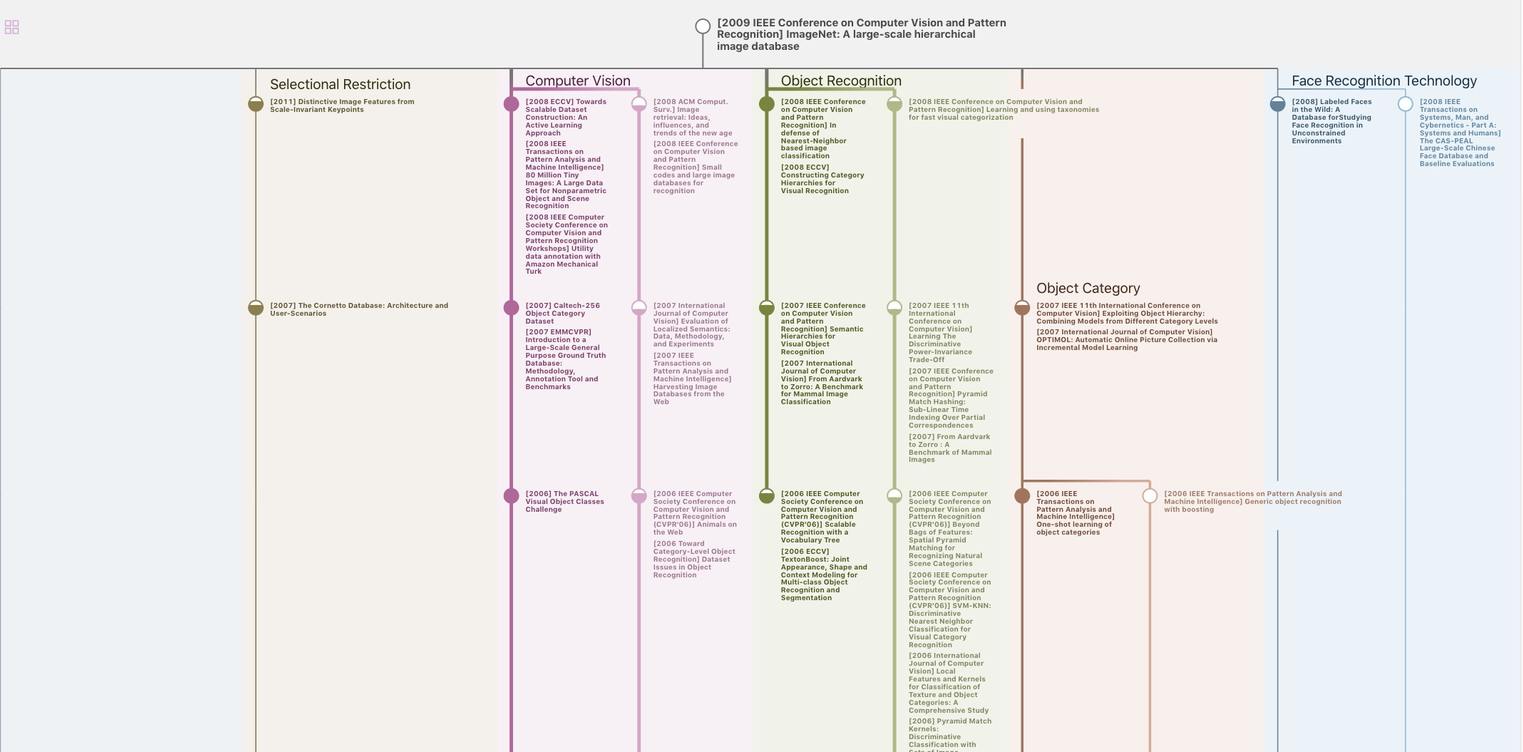
生成溯源树,研究论文发展脉络
Chat Paper
正在生成论文摘要
European Journal of Preventive Cardiology(2023)