Quantum Heavy-tailed Bandits
arXiv (Cornell University)(2023)
摘要
In this paper, we study multi-armed bandits (MAB) and stochastic linear bandits (SLB) with heavy-tailed rewards and quantum reward oracle. Unlike the previous work on quantum bandits that assumes bounded/sub-Gaussian distributions for rewards, here we investigate the quantum bandits problem under a weaker assumption that the distributions of rewards only have bounded $(1+v)$-th moment for some $v\in (0,1]$. In order to achieve regret improvements for heavy-tailed bandits, we first propose a new quantum mean estimator for heavy-tailed distributions, which is based on the Quantum Monte Carlo Mean Estimator and achieves a quadratic improvement of estimation error compared to the classical one. Based on our quantum mean estimator, we focus on quantum heavy-tailed MAB and SLB and propose quantum algorithms based on the Upper Confidence Bound (UCB) framework for both problems with $\Tilde{O}(T^{\frac{1-v}{1+v}})$ regrets, polynomially improving the dependence in terms of $T$ as compared to classical (near) optimal regrets of $\Tilde{O}(T^{\frac{1}{1+v}})$, where $T$ is the number of rounds. Finally, experiments also support our theoretical results and show the effectiveness of our proposed methods.
更多查看译文
关键词
Bandit Optimization,Adversarial Multi-Armed Bandits,Contextual Bandits,Quantum Machine Learning,Fault-tolerant Quantum Computation
AI 理解论文
溯源树
样例
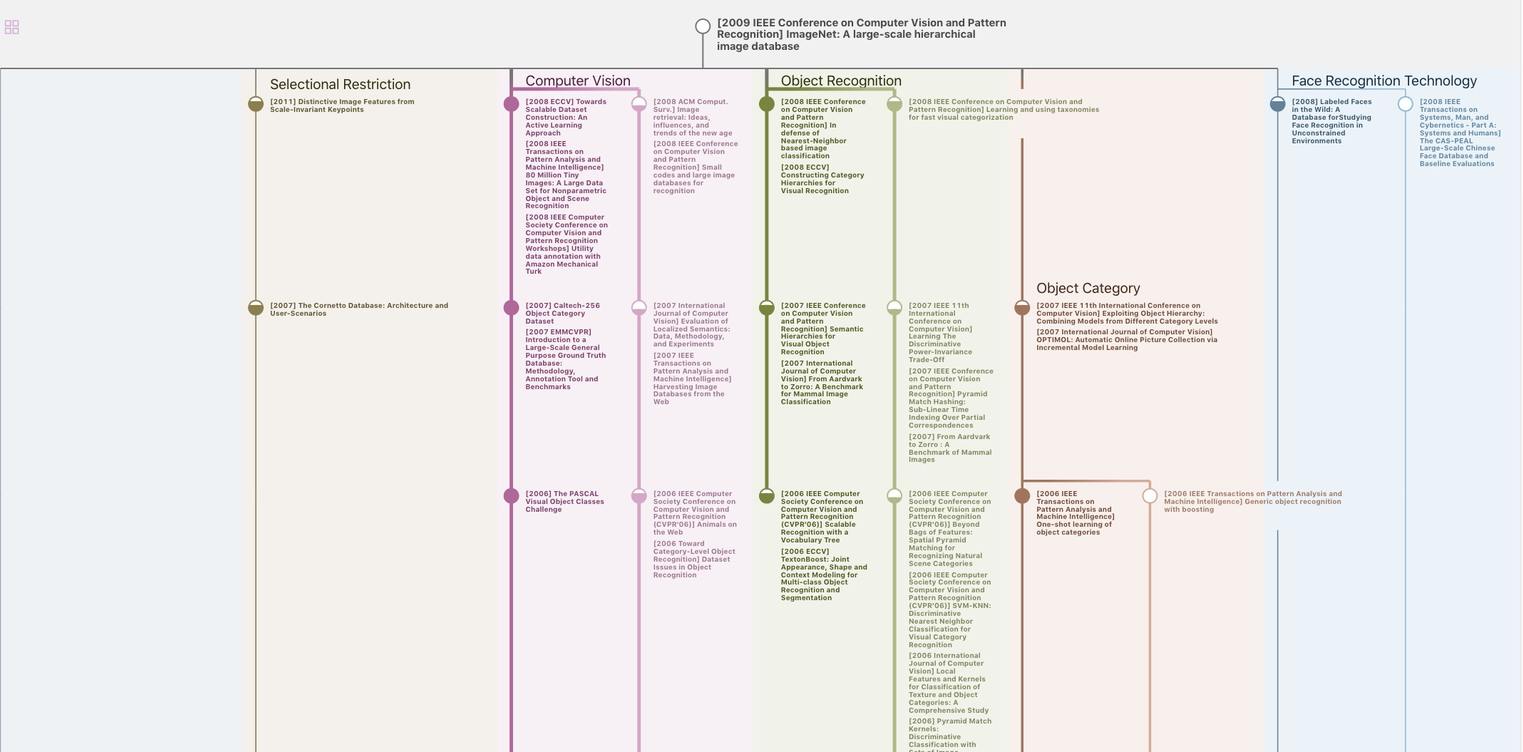
生成溯源树,研究论文发展脉络
Chat Paper
正在生成论文摘要