Structure Similarity Preservation Learning for Asymmetric Image Retrieval
IEEE TRANSACTIONS ON MULTIMEDIA(2024)
摘要
Asymmetric image retrieval is a task that seeks to balance retrieval accuracy and efficiency by leveraging lightweight and large models for the query and gallery sides, respectively. The key to asymmetric image retrieval is realizing feature compatibility between different models. Despite the great progress, most existing approaches either rely on classifiers inherited from gallery models or simply impose constraints at the instance level, ignoring the structure of embedding space. In this work, we propose a simple yet effective structure similarity preserving method to achieve feature compatibility between query and gallery models. Specifically, we first train a product quantizer offline with the image features embedded by the gallery model. The centroid vectors in the quantizer serve as anchor points in the embedding space of the gallery model to characterize its structure. During the training of the query model, anchor points are shared by the query and gallery models. The relationships between image features and centroid vectors are considered as structure similarities and constrained to be consistent. Moreover, our approach makes no assumption about the existence of any labeled training data and thus can be extended to an unlimited amount of data. Comprehensive experiments on large-scale landmark retrieval demonstrate the effectiveness of our approach.
更多查看译文
关键词
Feature extraction,Image retrieval,Training,Computational modeling,Adaptation models,Data models,Task analysis,Asymmetric image retrieval,multimedia search
AI 理解论文
溯源树
样例
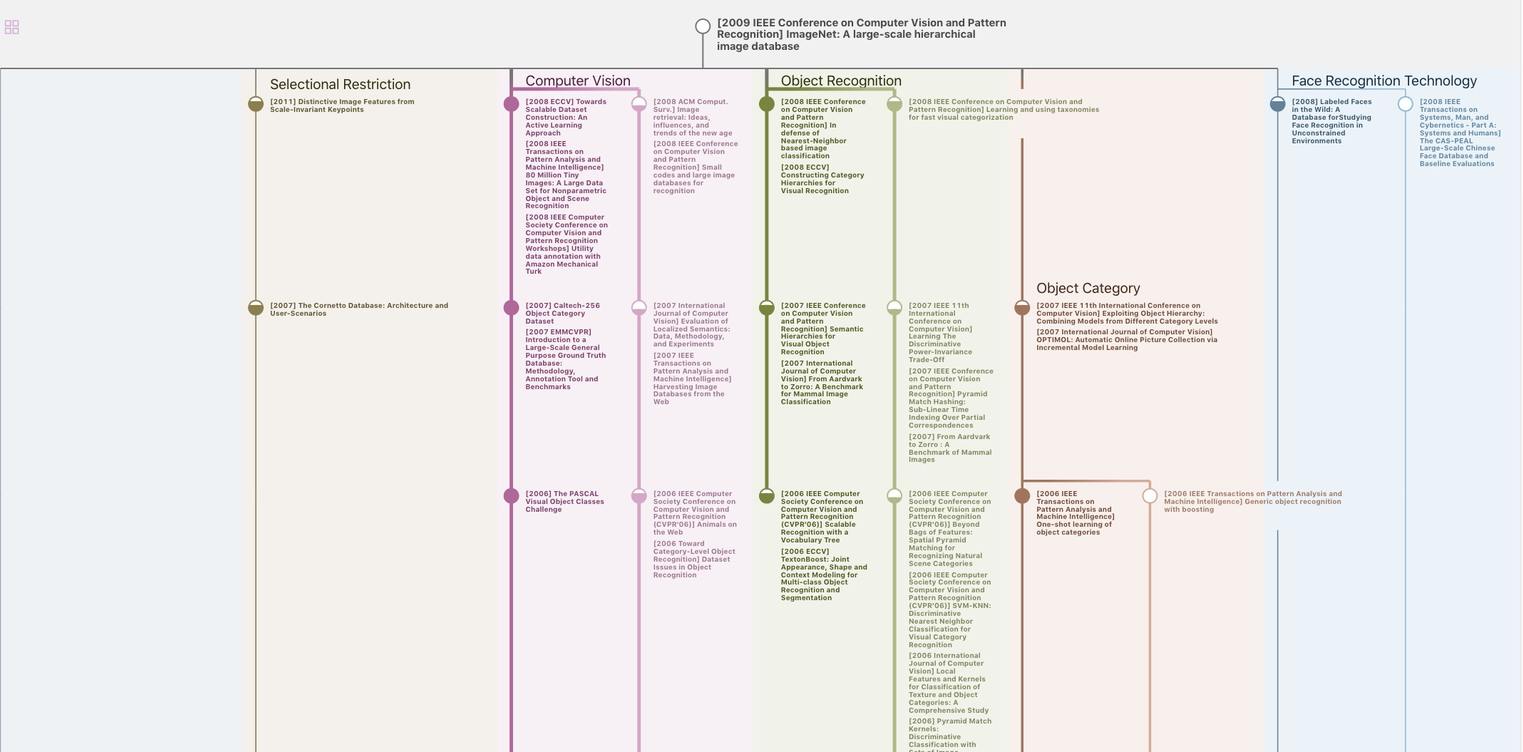
生成溯源树,研究论文发展脉络
Chat Paper
正在生成论文摘要